Multivariate Pattern Analysis of Lifelong Premature Ejaculation Based on Multiple Kernel Support Vector Machine
FRONTIERS IN PSYCHIATRY(2022)
摘要
ObjectiveThis study aimed to develop an effective support vector machine (SVM) classifier based on the multi-modal data for detecting the main brain networks involved in group separation of premature ejaculation (PE). MethodsA total of fifty-two patients with lifelong PE and 36 matched healthy controls were enrolled in this study. Structural MRI data, functional MRI data, and diffusion tensor imaging (DTI) data were used to process SPM12, DPABI4.5, and PANDA, respectively. A total of 12,735 features were reduced by the Mann-Whitney U test. The resilience nets method was further used to select features. ResultsFinally, 36 features (3 structural MRI, 7 functional MRI, and 26 DTI) were chosen in the training dataset. We got the best SVM model with an accuracy of 97.5% and an area under the curve (AUC) of 0.986 in the training dataset as well as an accuracy of 91.4% and an AUC of 0.966 in the testing dataset. ConclusionOur findings showed that the majority of the brain abnormalities for the classification was located within or across several networks. This study may contribute to the neural mechanisms of PE and provide new insights into the pathophysiology of patients with lifelong PE.
更多查看译文
关键词
machine learning, multivariate pattern analysis (MVPA), lifelong premature ejaculation (lifelong PE), neuroimaging, diffusion tensor imaging (DTI), MRI, support vector machine (SVM)
AI 理解论文
溯源树
样例
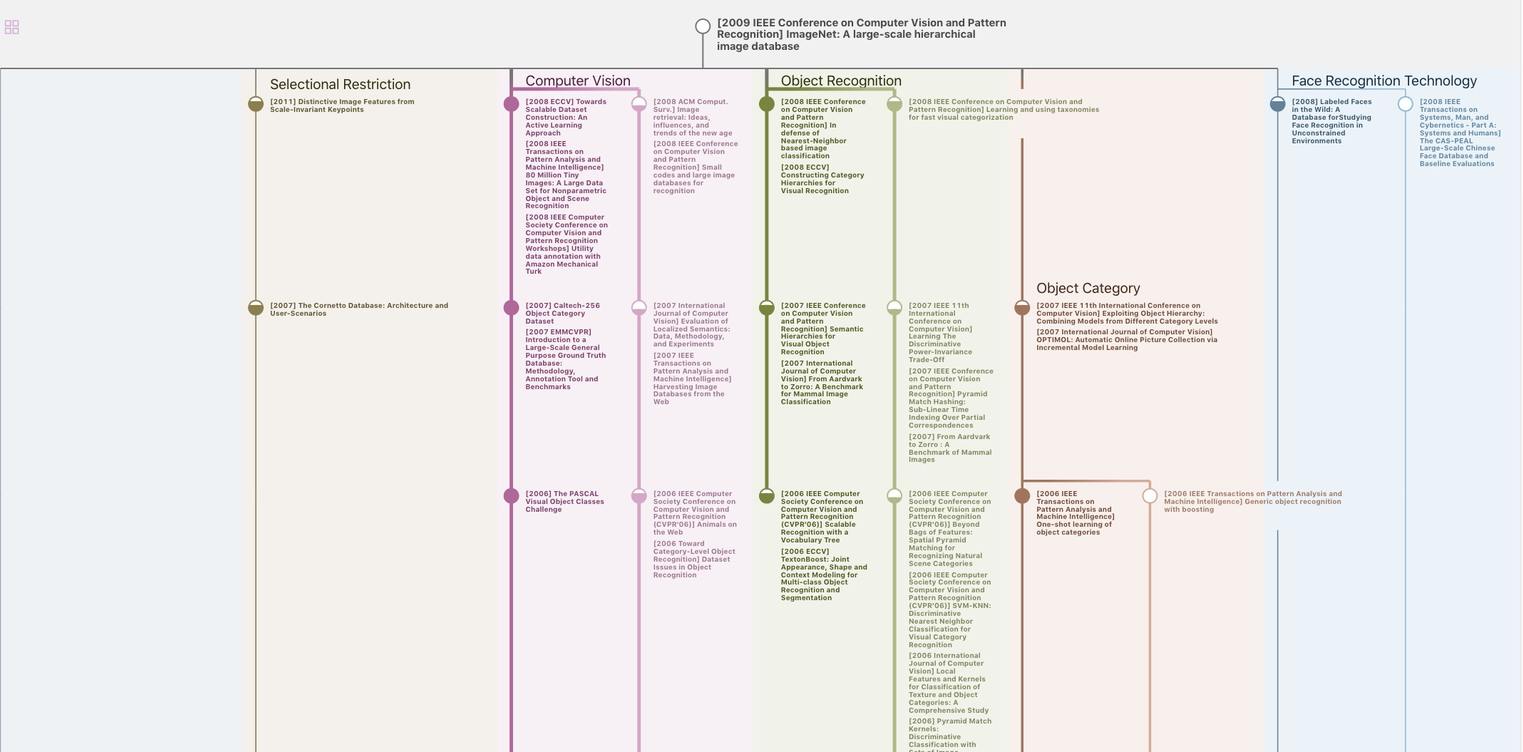
生成溯源树,研究论文发展脉络
Chat Paper
正在生成论文摘要