Sintering Quality Prediction Model Based on Semi-Supervised Dynamic Time Feature Extraction Framework.
SENSORS(2022)
摘要
In the sintering process, it is difficult to obtain the key quality variables in real time, so there is lack of real-time information to guide the production process. Furthermore, these labeled data are too few, resulting in poor performance of conventional soft sensor models. Therefore, a novel semi-supervised dynamic feature extraction framework (SS-DTFEE) based on sequence pre-training and fine-tuning is proposed in this paper. Firstly, based on the DTFEE model, the time features of the sequences are extended and extracted. Secondly, a novel weighted bidirectional LSTM unit (BiLSTM) is designed to extract the latent variables of original sequence data. Based on improved BiLSTM, an encoder-decoder model is designed as a pre-training model with unsupervised learning to obtain the hidden information in the process. Next, through model migration and fine-tuning strategy, the prediction performance of labeled datasets is improved. The proposed method is applied in the actual sintering process to estimate the FeO content, which shows a significant improvement of the prediction accuracy, compared to traditional methods.
更多查看译文
关键词
LSTM, semi-supervised learning, FeO content, soft sensor, encoder-decoder, dynamic feature extraction
AI 理解论文
溯源树
样例
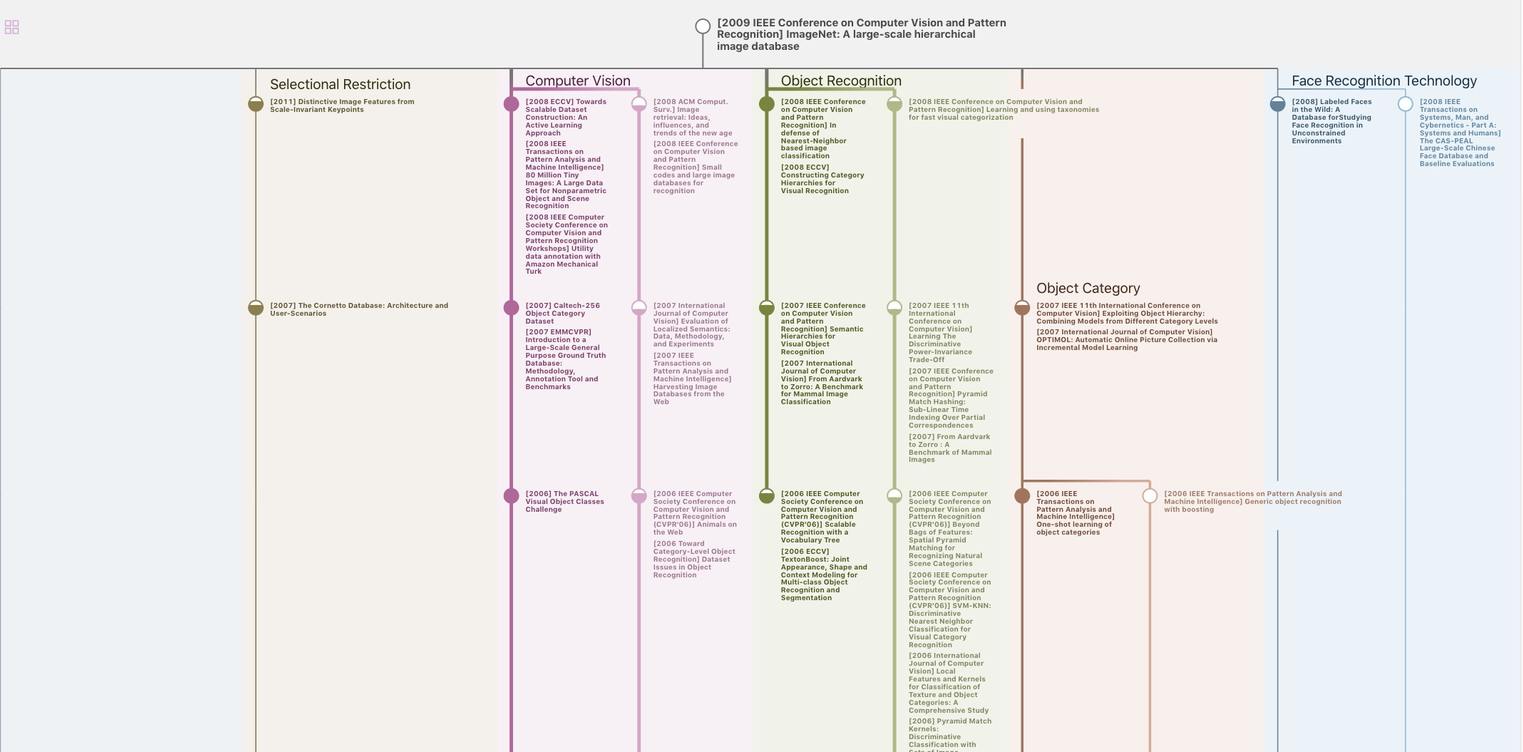
生成溯源树,研究论文发展脉络
Chat Paper
正在生成论文摘要