Using Interpretable Machine Learning to Identify Baseline Predictive Factors of Remission and Drug Durability in Crohn's Disease Patients on Ustekinumab
JOURNAL OF CLINICAL MEDICINE(2022)
摘要
Ustekinumab has shown efficacy in Crohn's Disease (CD) patients. To identify patient profiles of those who benefit the most from this treatment would help to position this drug in the therapeutic paradigm of CD and generate hypotheses for future trials. The objective of this analysis was to determine whether baseline patient characteristics are predictive of remission and the drug durability of ustekinumab, and whether its positioning with respect to prior use of biologics has a significant effect after correcting for disease severity and phenotype at baseline using interpretable machine learning. Patients' data from SUSTAIN, a retrospective multicenter single-arm cohort study, were used. Disease phenotype, baseline laboratory data, and prior treatment characteristics were documented. Clinical remission was defined as the Harvey Bradshaw Index <= 4 and was tracked longitudinally. Drug durability was defined as the time until a patient discontinued treatment. A total of 439 participants from 60 centers were included and a total of 20 baseline covariates considered. Less exposure to previous biologics had a positive effect on remission, even after controlling for baseline disease severity using a non-linear, additive, multivariable model. Additionally, age, body mass index, and fecal calprotectin at baseline were found to be statistically significant as independent negative risk factors for both remission and drug survival, with further risk factors identified for remission.
更多查看译文
关键词
Crohn's Disease, ustekinumab, predictive factors
AI 理解论文
溯源树
样例
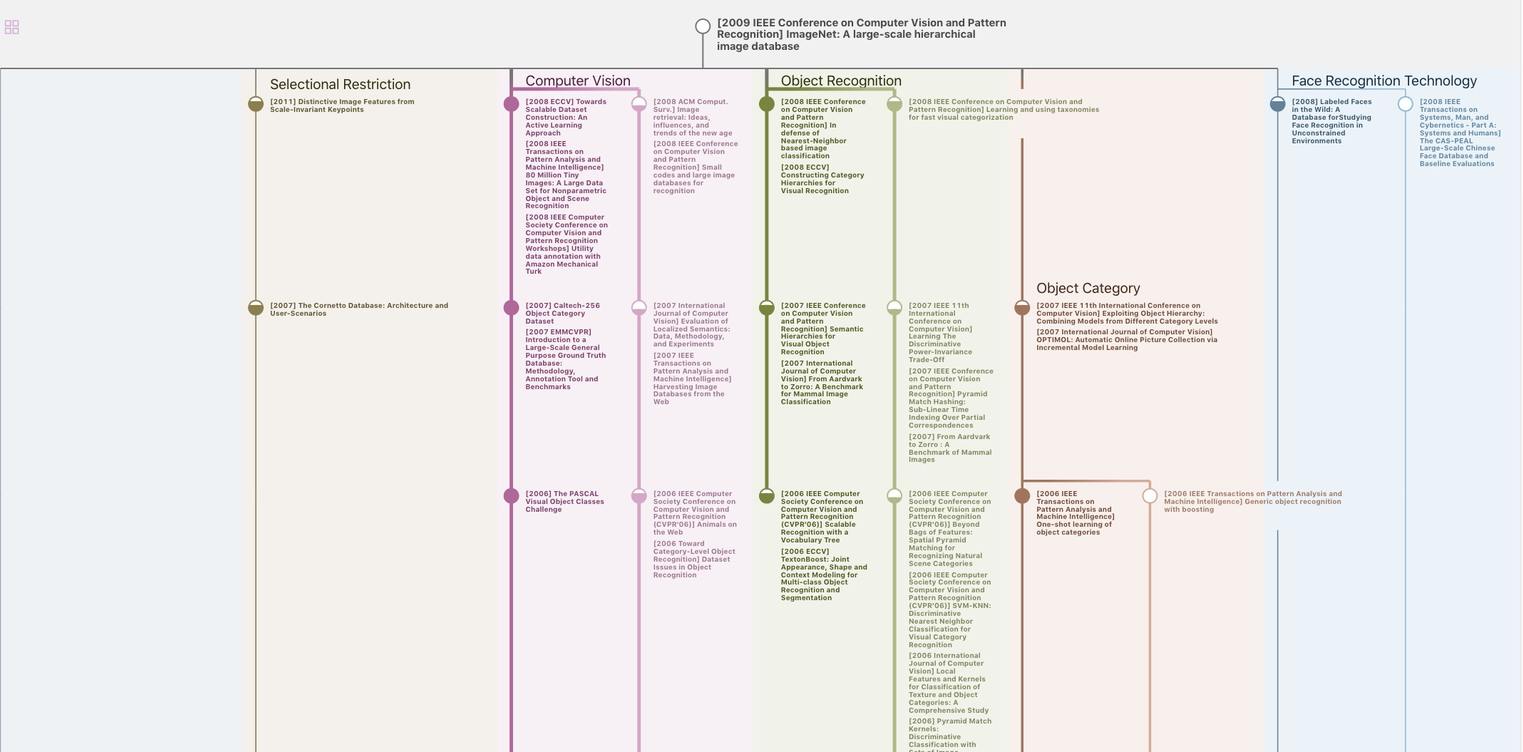
生成溯源树,研究论文发展脉络
Chat Paper
正在生成论文摘要