Visual Localization via Few-Shot Scene Region Classification
2022 International Conference on 3D Vision (3DV)(2022)
摘要
Visual (re)localization addresses the problem of estimating the 6-DoF (Degree of Freedom) camera pose of a query image captured in a known scene, which is a key building block of many computer vision and robotics applications. Recent advances in structure-based localization solve this problem by memorizing the mapping from image pixels to scene coordinates with neural networks to build 2D-3D correspondences for camera pose optimization. However, such memorization requires training by amounts of posed images in each scene, which is heavy and inefficient. On the contrary, few-shot images are usually sufficient to cover the main regions of a scene for a human operator to perform visual localization. In this paper, we propose a scene region classification approach to achieve fast and effective scene memorization with few-shot images. Our insight is leveraging a) pre-learned feature extractor, b) scene region classifier, and c) meta-learning strategy to accelerate training while mitigating overfitting. We evaluate our method on both indoor and outdoor benchmarks. The experiments validate the effectiveness of our method in the few-shot setting, and the training time is significantly reduced to only a few minutes.
1
1
Code available at: https://github.com/siyandong/SRC
更多查看译文
关键词
Visual Localization,Few Shot,Scene Region Classification,Scene Coordinate Regression
AI 理解论文
溯源树
样例
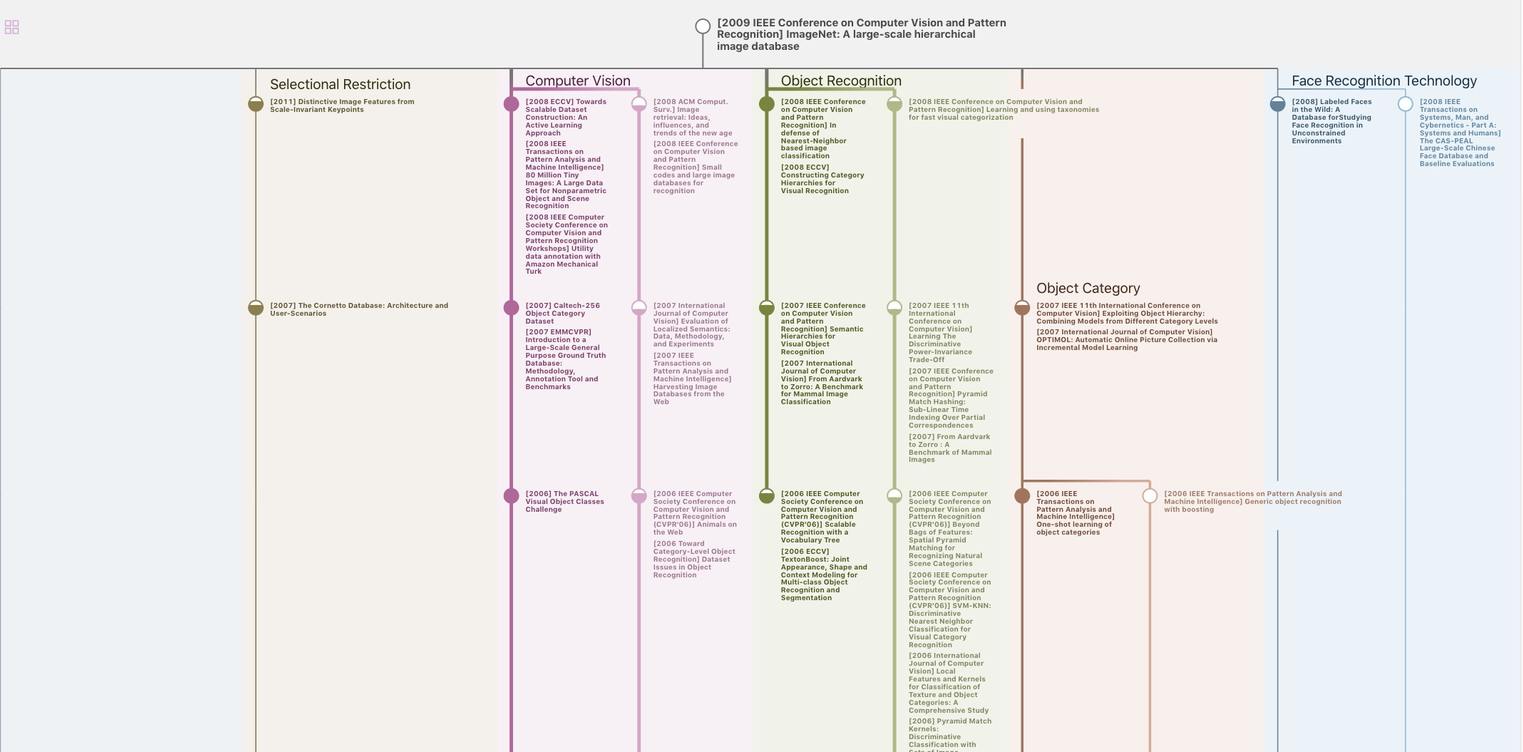
生成溯源树,研究论文发展脉络
Chat Paper
正在生成论文摘要