Reduced implication-bias logic loss for neuro-symbolic learning
Machine Learning(2024)
摘要
Integrating logical reasoning and machine learning by approximating logical inference with differentiable operators is a widely used technique in the field of Neuro-Symbolic Learning. However, some differentiable operators could introduce significant biases during backpropagation, which can degrade the performance of Neuro-Symbolic systems. In this paper, we demonstrate that the loss functions derived from fuzzy logic operators commonly exhibit a bias, referred to as Implication Bias . To mitigate this bias, we propose a simple yet efficient method to transform the biased loss functions into Reduced Implication-bias Logic Loss (RILL) . Empirical studies demonstrate that RILL outperforms the biased logic loss functions, especially when the knowledge base is incomplete or the supervised training data is insufficient.
更多查看译文
关键词
Implication bias,Neuro-symbolic learning,Neural networks,Machine learning
AI 理解论文
溯源树
样例
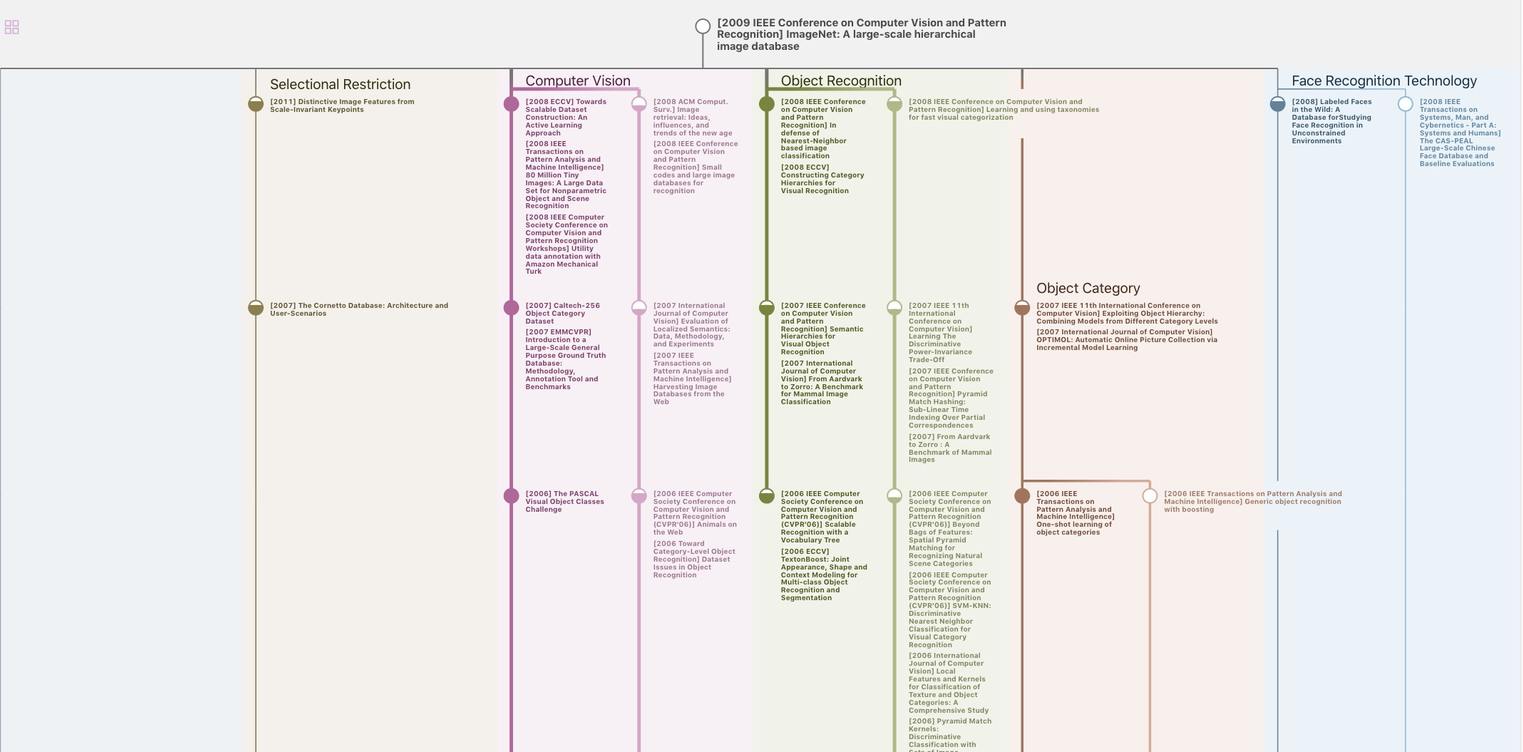
生成溯源树,研究论文发展脉络
Chat Paper
正在生成论文摘要