Lyapunov Design for Robust and Efficient Robotic Reinforcement Learning
arxiv(2022)
摘要
Recent advances in the reinforcement learning (RL) literature have enabled roboticists to automatically train complex policies in simulated environments. However, due to the poor sample complexity of these methods, solving RL problems using real-world data remains a challenging problem. This paper introduces a novel cost-shaping method which aims to reduce the number of samples needed to learn a stabilizing controller. The method adds a term involving a Control Lyapunov Function (CLF) -- an `energy-like' function from the model-based control literature -- to typical cost formulations. Theoretical results demonstrate the new costs lead to stabilizing controllers when smaller discount factors are used, which is well-known to reduce sample complexity. Moreover, the addition of the CLF term `robustifies' the search for a stabilizing controller by ensuring that even highly sub-optimal polices will stabilize the system. We demonstrate our approach with two hardware examples where we learn stabilizing controllers for a cartpole and an A1 quadruped with only seconds and a few minutes of fine-tuning data, respectively. Furthermore, simulation benchmark studies show that obtaining stabilizing policies by optimizing our proposed costs requires orders of magnitude less data compared to standard cost designs.
更多查看译文
关键词
reinforcement,learning,robust
AI 理解论文
溯源树
样例
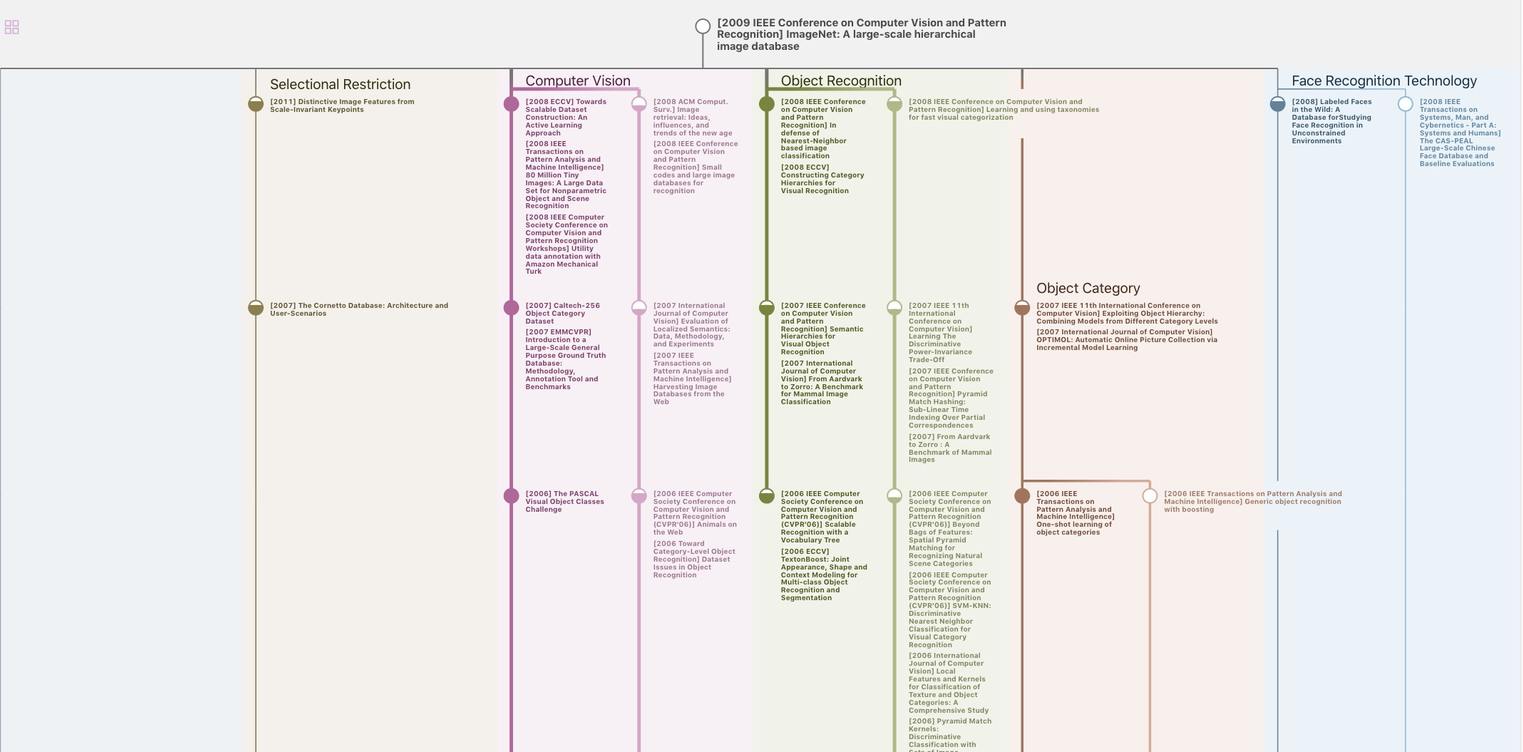
生成溯源树,研究论文发展脉络
Chat Paper
正在生成论文摘要