Learning Relative Feature Displacement for Few-Shot Open-Set Recognition
IEEE TRANSACTIONS ON MULTIMEDIA(2023)
摘要
Few-shot learning (FSL) usually assumes that the query is drawn from the same label space as the support set, while queries from unknown classes may emerge unexpectedly in many open-world application scenarios. Such an open-set issue will limit the practical deployment of FSL systems, which remains largely unexplored. In this paper, we investigate the problem of few-shot open-set recognition (FSOR) and propose a novel solution, called Relative Feature Displacement Network (RFDNet), which empowers FSL systems to reject queries from unknown classes while accurately classifying those from known classes. First, we suggest a different relative feature displacement learning (RFDL) paradigm for FSOR, i.e., meta-learning a feature displacement relative to a pretrained reference feature embedding, based on our insightful observations on the randomness drift issue of previous meta-learning based for FSOR methods, as well as the generalization ability of the feature embedding pretrained for general classification. Second, we design the RFDNet framework to implement the RFDL paradigm, which is mainly featured by a task aware RFD generator and a marginal open-set loss. Comprehensive experiments on three public datasets, i.e., mini ImageNet, CIFARFS and tiered ImageNet, demonstrate that RFDNet can consistently outperform the state-of-the-art methods, achieving improvement of 5.2%, 2.0% and 1.7% respectively, in terms of AUROC for unknown-class rejection under the 5-way 5-shot setting.
更多查看译文
关键词
Few-shot learning,open-set recognition,relative feature displacement,randomness drift
AI 理解论文
溯源树
样例
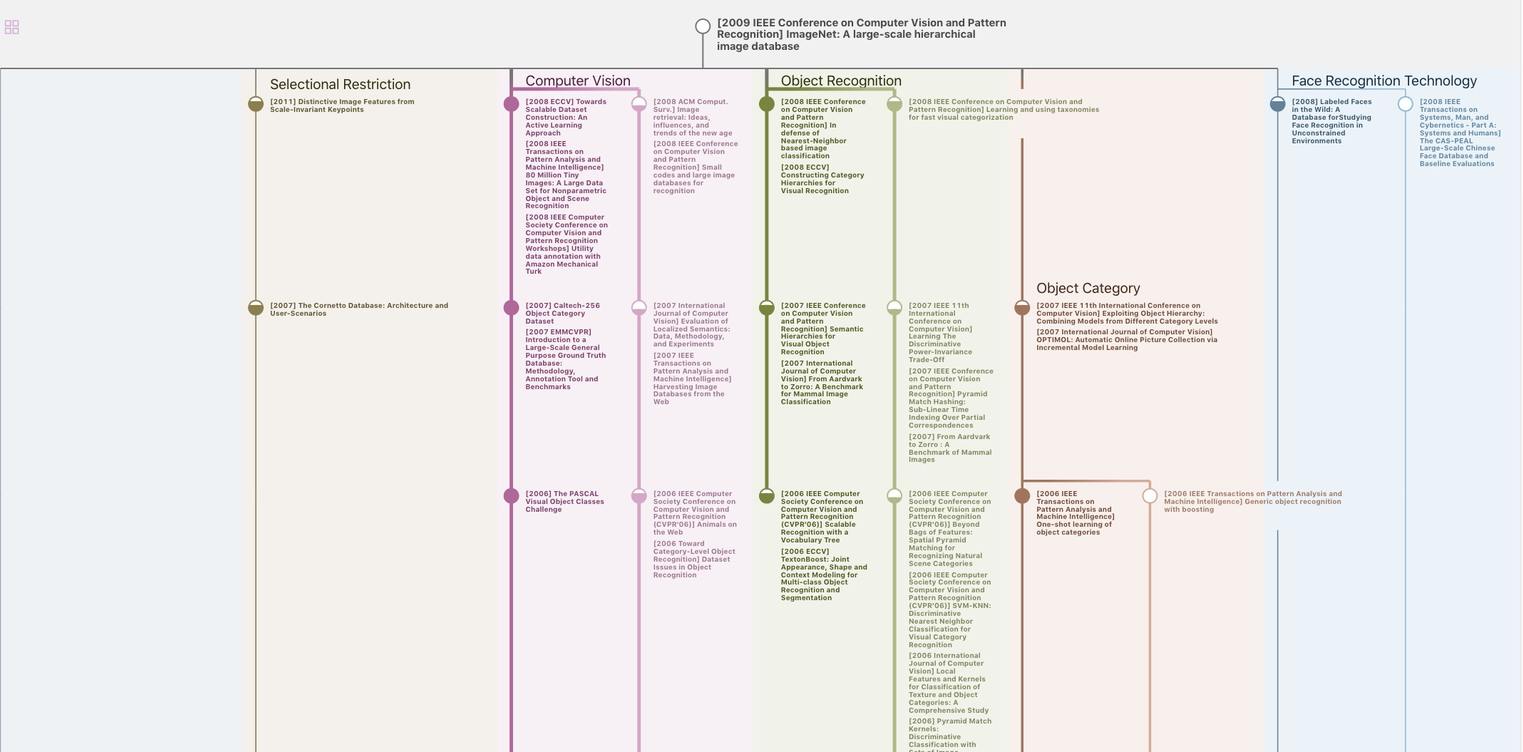
生成溯源树,研究论文发展脉络
Chat Paper
正在生成论文摘要