Scholastic: Graphical Human-AI Collaboration for Inductive and Interpretive Text Analysis
User Interface Software and Technology(2022)
摘要
ABSTRACT Interpretive scholars generate knowledge from text corpora by manually sampling documents, applying codes, and refining and collating codes into categories until meaningful themes emerge. Given a large corpus, machine learning could help scale this data sampling and analysis, but prior research shows that experts are generally concerned about algorithms potentially disrupting or driving interpretive scholarship. We take a human-centered design approach to addressing concerns around machine-assisted interpretive research to build Scholastic, which incorporates a machine-in-the-loop clustering algorithm to scaffold interpretive text analysis. As a scholar applies codes to documents and refines them, the resulting coding schema serves as structured metadata which constrains hierarchical document and word clusters inferred from the corpus. Interactive visualizations of these clusters can help scholars strategically sample documents further toward insights. Scholastic demonstrates how human-centered algorithm design and visualizations employing familiar metaphors can support inductive and interpretive research methodologies through interactive topic modeling and document clustering.
更多查看译文
关键词
qualitative research, interpretive research methods, interactive topic modeling, interactive document clustering, human-AI collaboration, visual analytics, text data
AI 理解论文
溯源树
样例
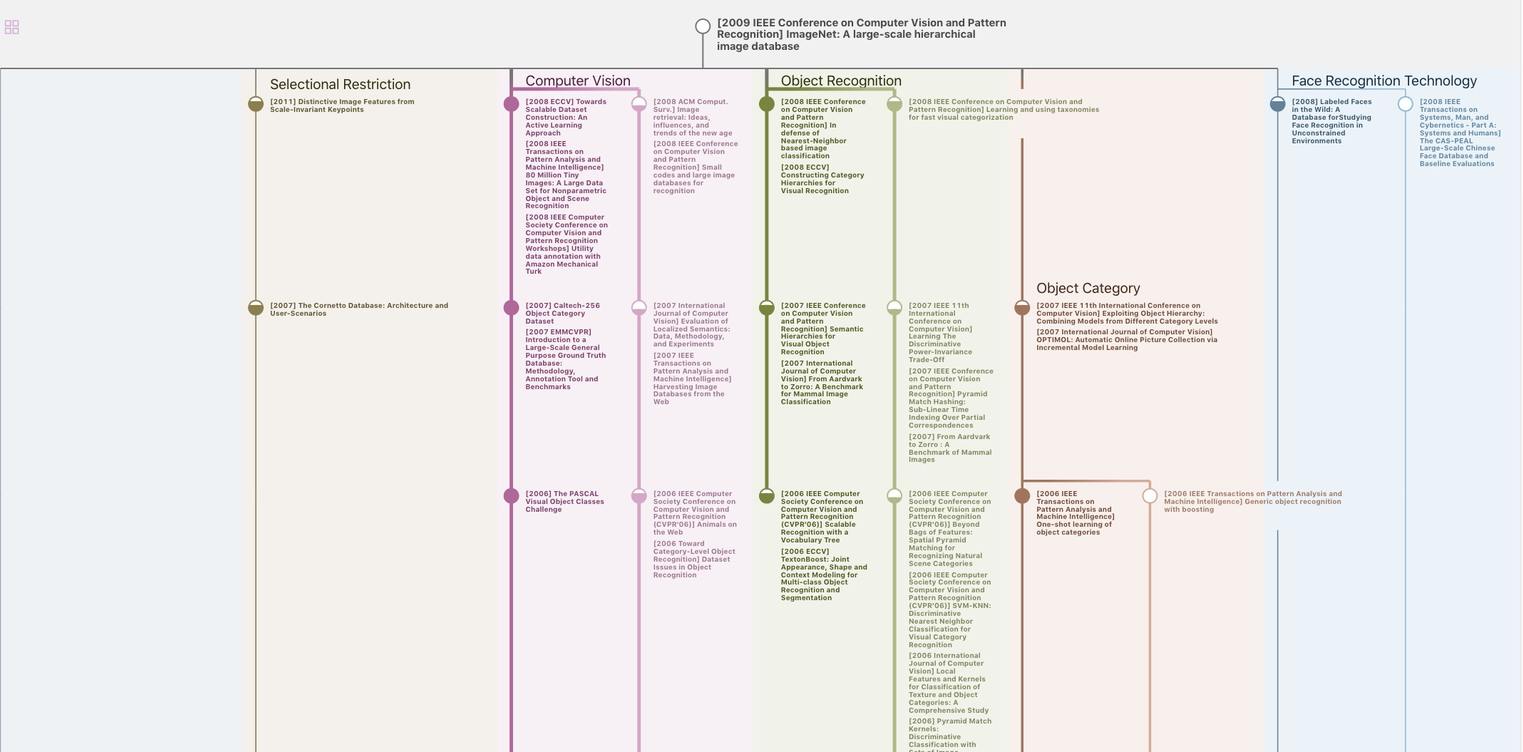
生成溯源树,研究论文发展脉络
Chat Paper
正在生成论文摘要