Analysis of Search Landscape Samplers for Solver Performance Prediction on a University Timetabling Problem
Parallel Problem Solving from Nature – PPSN XVII(2022)
摘要
Landscape metrics have proven their effectiveness in building predictive models, including when applied to University Timetabling, a highly neutral problem. In this paper, two Iterated Local Search algorithms sample search space to obtain over 100 landscape metrics. The only difference between the samplers is the exploration strategy. One uses neutral acceptance while the other only accepts strictly improving neighbors. Different sampling time budgets are considered in order to study the evolution of the fitness networks and the predictive power of their metrics. Then, the performance of three solvers, Simulated Annealing and two versions of a Hybrid Local Search, are predicted using a selection of landscape metrics. Using the data gathered, we are able to determine the best sampling strategy and the minimum sampling time budget for models that are able to effectively predict the performance of the solvers on unknown instances.
更多查看译文
关键词
University Timetabling, Performance prediction, Landscape analysis, Local search
AI 理解论文
溯源树
样例
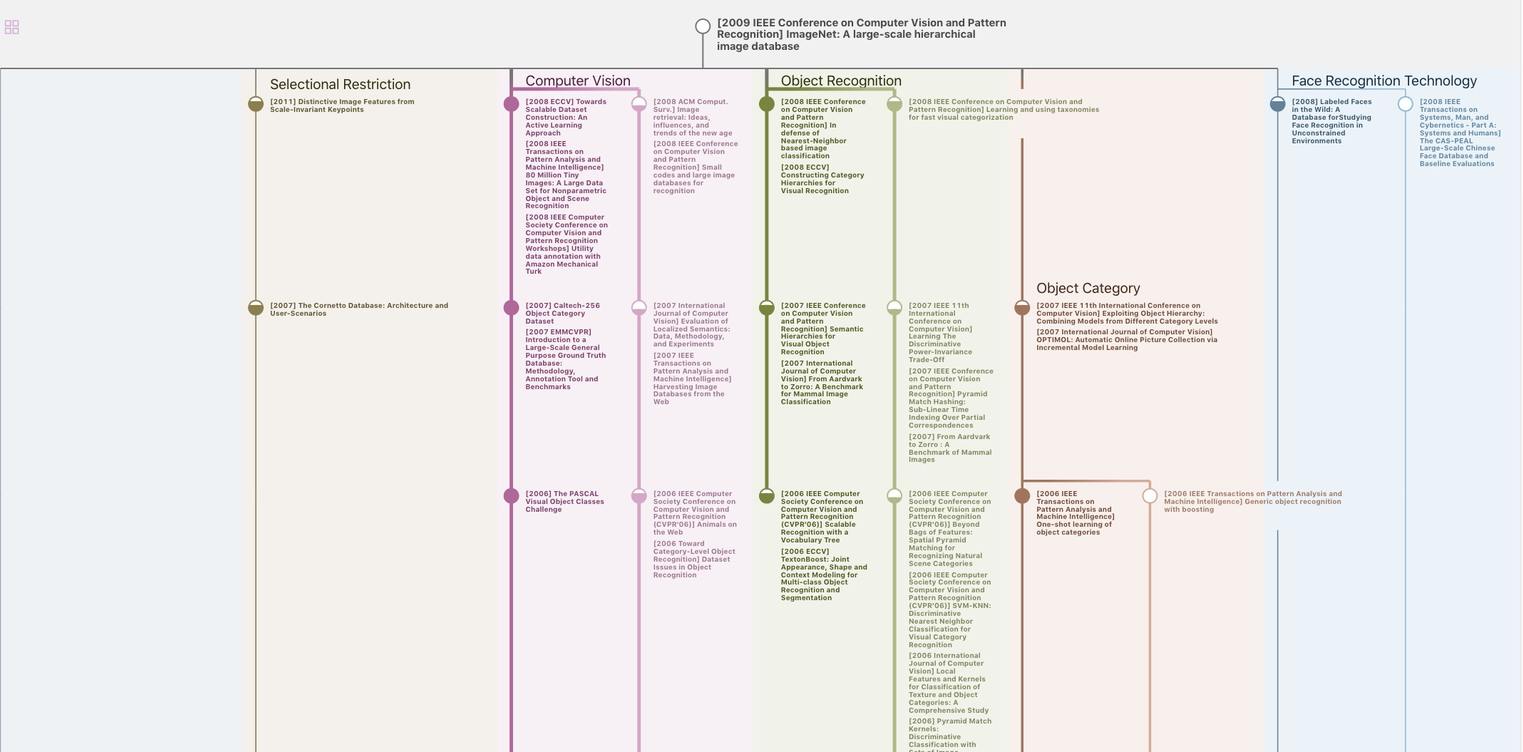
生成溯源树,研究论文发展脉络
Chat Paper
正在生成论文摘要