Swtru
Computers in Biology and Medicine(2022)
摘要
In the last decade, deep neural networks have been widely applied to medical image segmentation, achieving good results in computer-aided diagnosis tasks etc. However, the task of segmenting highly complex, low-contrast images of organs and tissues with high accuracy still faces great challenges. To better address this challenge, this paper proposes a novel model SWTRU (Star-shaped Window Transformer Reinforced U-Net) by combining the U-Net network which plays well in the image segmentation field, and the Transformer which possesses a powerful ability to capture global contexts. Unlike the previous methods that import the Transformer into U-Net, an improved Star-shaped Window Transformer is introduced into the decoder of the SWTRU to enhance the decision-making capability of the whole method. The SWTRU uses a redesigned multi-scale skip-connection model, which retains the inductive bias of the original FCN structure for images while obtaining fine-grained features and coarse-grained semantic information. Our method also presents the FFIM (Filtering Feature Integration Mechanism) to integration and dimensionality reduction of the fused multi-layered features, which reduces the computation. Our SWTRU yields 0.972 DICE on CHLISC for liver and tumor segmentation, 0.897 DICE on LGG for glioma segmentation, and 0.904 DICE on ISIC2018 for skin diseases’ segmentation, achieves substantial improvements over the current SoTA across 9 different medical image segment methods. SWTRU can combine feature mapping from different scales, high-level semantics, and global contextual relationships, this architecture is effective in the medical image segmentation. The experimental findings indicate that SWTRU produces superior performance on the medical image segmentation tasks. • Propose a new model SWTRU combines CNN and Transformer. • Propose Star-shaped Window self-attention mechanism. • Design a new multiscale skip-connection mechanism. • Introduce the Filtering Feature Integration Mechanism to reduce the computation.
更多查看译文
关键词
Medical images segmentation,Transformers,Self-attention,Feature fusion,Full-scale skip connection
AI 理解论文
溯源树
样例
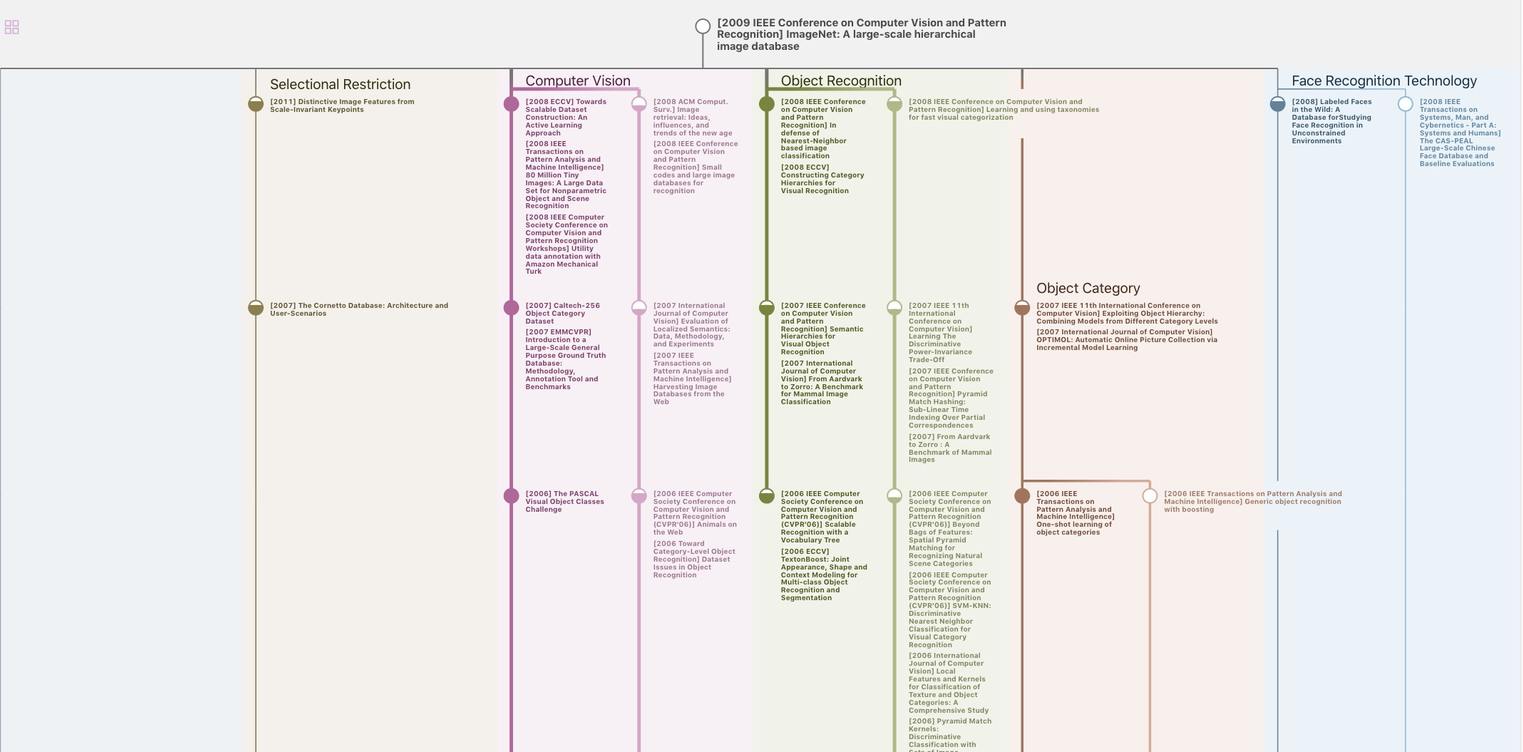
生成溯源树,研究论文发展脉络
Chat Paper
正在生成论文摘要