How does Heterophily Impact the Robustness of Graph Neural Networks?
Proceedings of the 28th ACM SIGKDD Conference on Knowledge Discovery and Data Mining(2022)
摘要
We bridge two research directions on graph neural networks (GNNs), by formalizing the relation between heterophily of node labels (i.e., connected nodes tend to have dissimilar labels) and the robustness of GNNs to adversarial attacks. Our theoretical and empirical analyses show that for homophilous graph data, impactful structural attacks always lead to reduced homophily, while for heterophilous graph data the change in the homophily level depends on the node degrees. These insights have practical implications for defending against attacks on real-world graphs: we deduce that separate aggregators for ego- and neighbor-embeddings, a design principle which has been identified to significantly improve prediction for heterophilous graph data, can also offer increased robustness to GNNs. Our comprehensive experiments show that GNNs merely adopting this design achieve improved empirical and certifiable robustness compared to the best-performing unvaccinated model. Additionally, combining this design with explicit defense mechanisms against adversarial attacks leads to an improved robustness with up to 18.33% performance increase under attacks compared to the best-performing vaccinated model.
更多查看译文
关键词
graph neural networks,robustness,heterophily
AI 理解论文
溯源树
样例
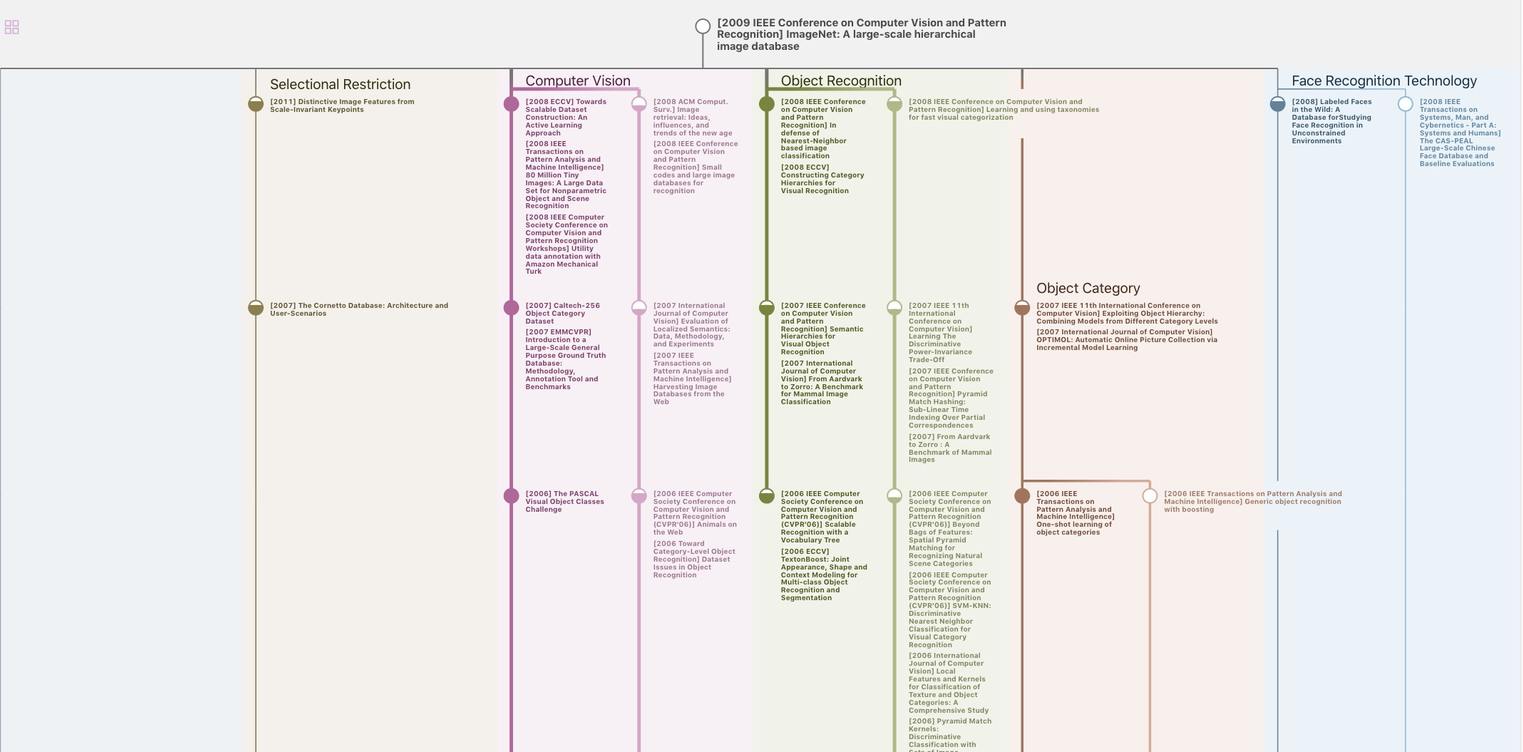
生成溯源树,研究论文发展脉络
Chat Paper
正在生成论文摘要