Elastic distances for time-series classification: Itakura versus Sakoe-Chiba constraints
Knowledge and Information Systems(2022)
摘要
In the field of time series data mining, the accuracy of the simple, but very successful nearest neighbor (NN) classifier directly depends on the chosen similarity measure. To improve the efficiency of elastic measures introduced to overcome the shortcomings of Euclidean distance, the Sakoe-Chiba band is usually applied as a constraint. In this paper, we provide a detailed analysis of the influence of the alternative Itakura parallelogram constraint on the accuracy of the NN classifier in combination with four well-known elastic measures, compared to the Sakoe-Chiba constraint and the unconstrained variants of these measures. The findings suggest that, although the Sakoe-Chiba band generally produces better results, for certain types of datasets the Itakura parallelogram represents a better choice.
更多查看译文
关键词
Time series,Elastic distances,Constraints,Classification
AI 理解论文
溯源树
样例
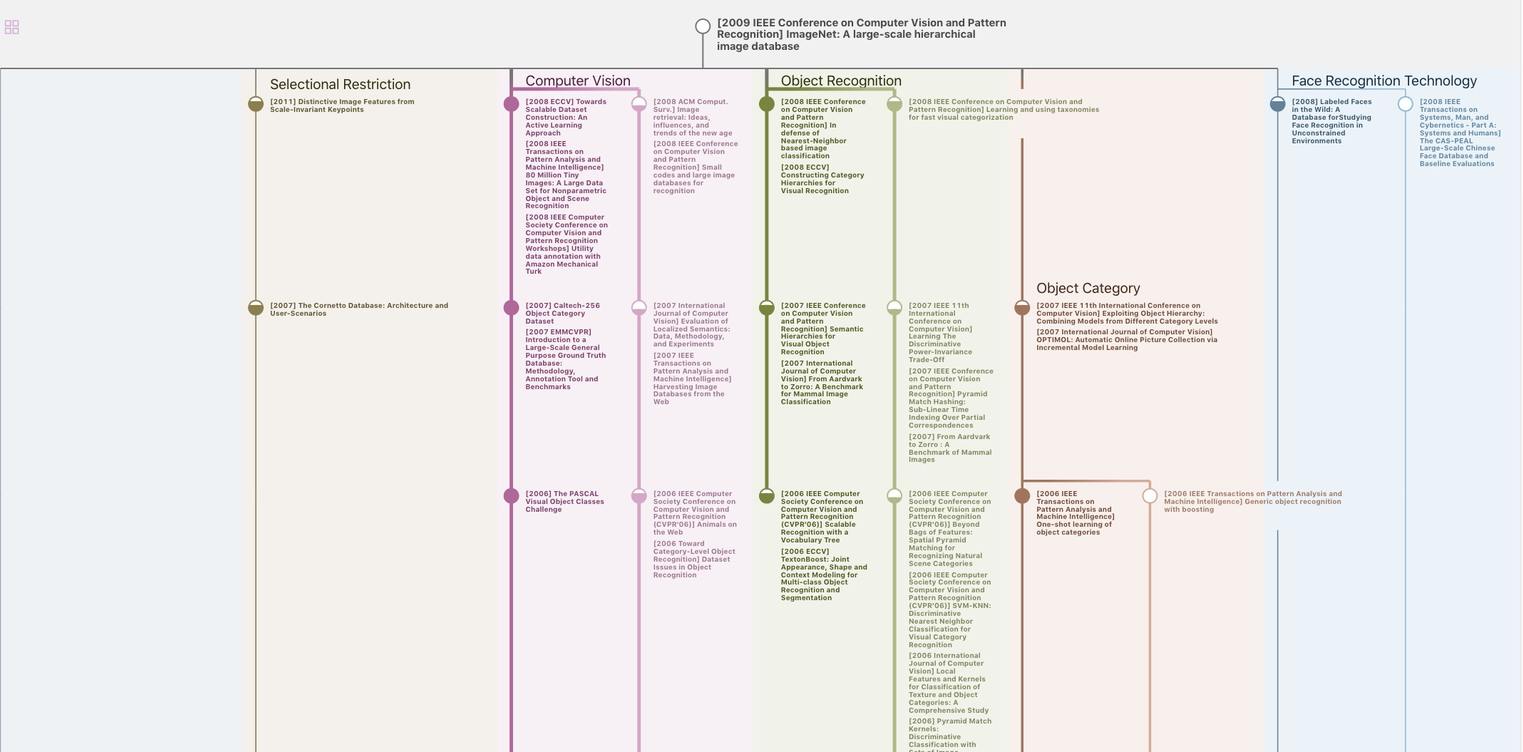
生成溯源树,研究论文发展脉络
Chat Paper
正在生成论文摘要