Photovoltaic Power intra‐ and day‐ahead Predictions with Differential Learning producing PDE‐modular Models based on the Node L‐transform Derivatives
Environmental Progress & Sustainable Energy(2022)
摘要
Predictions of photovoltaic (PV) energy supply, based on data statistics of weather and PV records, are required in short-intra and day-ahead planning of PV plant operations. Numerical weather prediction (NWP), based on physical consideration, can simulate the progress of local cloudiness, although their prognoses are usually delayed by a few hours and are not provided with the quality desired by PV operators. Differential polynomial neural network (D-PNN) is an unconventional hybrid regression technique, based on a novel learning strategy and able to resolve some problems in modeling patterns in high-dynamical and chaotic non-deterministic systems. D-PNN splits the general partial differential equation (PDE) of the k(th) order into a summation form of two-variable PDEs of a predetermined low order. PDE derivatives are transformed and separated into the Laplace counterparts, from which the unknown node originals are restored using the inverse L-operation. D-PNN searches for the best 2-input couples, inserting node by node into its changeable tree structure, to expand the modular sum model by usable PDE components. The PDE-modularization enhances the D-PNN capability in modeling high uncertainty in weather patterns in the ground-layer atmosphere, analogous to NWP. The designed PV intra- and day-ahead prediction schemes, using hourly increasing and fixed-time prediction horizons, were compared with different computing approaches based on differential, probabilistic and statistical learning. Machine learning (ML) models calculate the output PV power in each single hour separately or in an all-day processing series with trained input delay. The results are analyzed and interpreted for each day and prediction hour. The quantitative results for both prediction procedures are comparable, showing the average intraday 11.6% and all-day 9.6% (or rather 12.3% respectively) inaccuracy with respect to the daily peak power. Training data samples with spatial overlap in the regional scope and ML initialized similarity intervals are related to the fixed-day or hourly increased input-output delay. This approach can compensate for unpredictable midterm variances in local weather patterns (caused by terrain or convection anomalies) and possible errors in model initialization. PV power was converted to and restored from the clear sky index in the modeling input/output, which represents its relative values, disregarding the actual solar cycle time.
更多查看译文
关键词
differential equation, differential learning, differential network, Laplace transformation, PDE-component, periodic conversion, PVP modeling, rational conversion
AI 理解论文
溯源树
样例
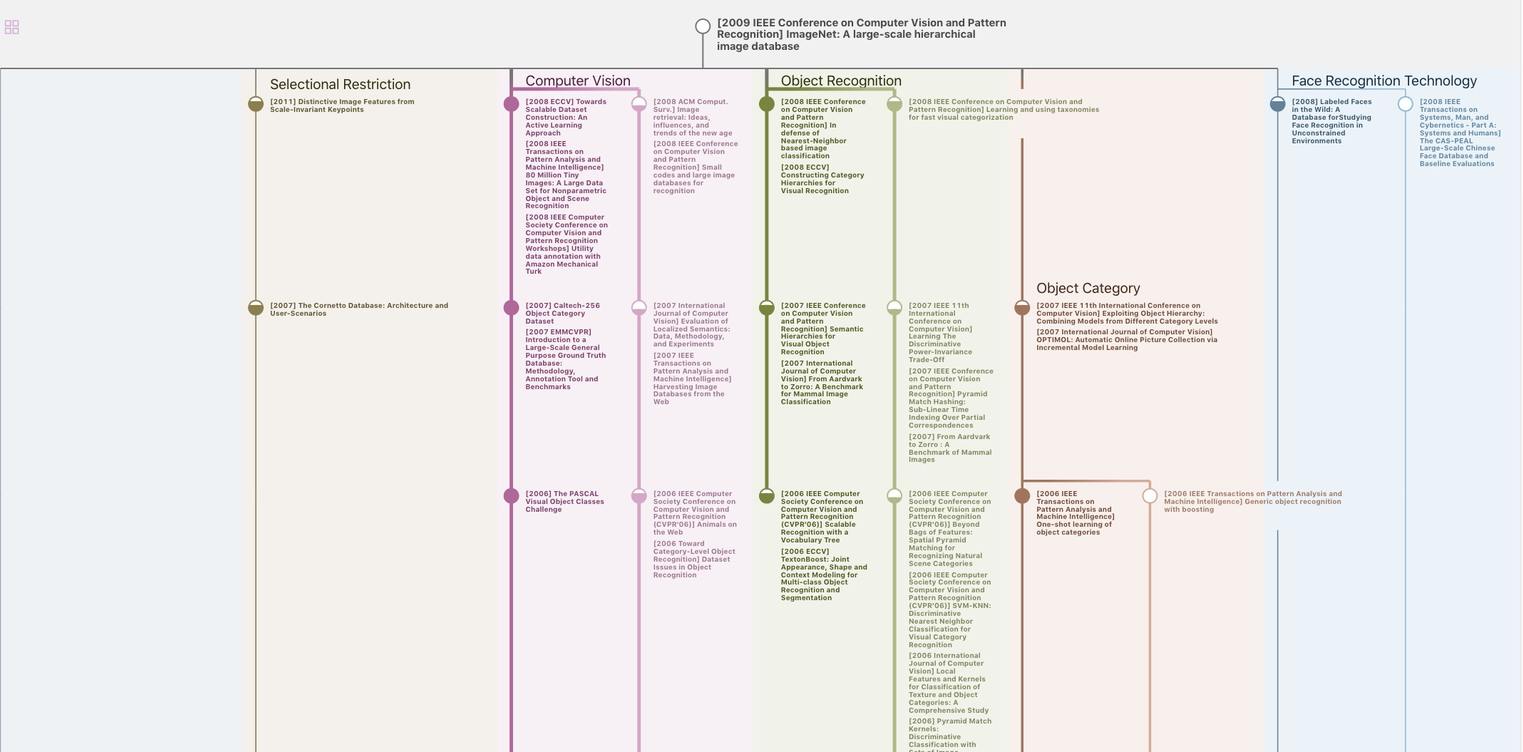
生成溯源树,研究论文发展脉络
Chat Paper
正在生成论文摘要