A comparative study on class-imbalanced gas turbine fault diagnosis
PROCEEDINGS OF THE INSTITUTION OF MECHANICAL ENGINEERS PART G-JOURNAL OF AEROSPACE ENGINEERING(2023)
摘要
Gas turbines are widely used in various fields, and the failure of gas turbines can cause catastrophic consequences. Health condition monitoring and fault diagnosis of gas turbines can detect faults timely, avoid serious faults, and significantly reduce maintenance costs. Thus, fault diagnosis of gas turbines has great significance. Current researches on gas turbine fault diagnosis mainly focus on the case of abundant fault samples. However, fault data are very rare and the number of normal samples is much larger than the number of fault samples in the industrial scene. This class-imbalance problem widely exists but is hardly focused in the field of gas turbine fault diagnosis. Aiming to solve this problem, this paper introduces the concept of class-imbalanced learning from the machine learning field, summarizes three kinds of class-imbalance addressment methods including oversampling, undersampling, and sample weighting, and proposes a new combination method of focal loss and random oversampling for addressing class-imbalance in deep neural networks, and performs a systematic comparative study on class-imbalanced gas turbine fault diagnosis. Experimental results show that class-imbalance can seriously reduce the fault diagnosis accuracy. Through these class-imbalance addressment methods, diagnosis accuracy is greatly improved. Comparative experiments also show that the proposed combination method can obtain the best diagnosis accuracy among all the compared methods in class-imbalanced situation. Through this comparative study, a detailed guideline for improving diagnosis accuracy under class-imbalanced circumstance is provided.
更多查看译文
关键词
Gas turbine, fault diagnosis, class-imbalanced learning, machine learning, deep neural network, focal loss
AI 理解论文
溯源树
样例
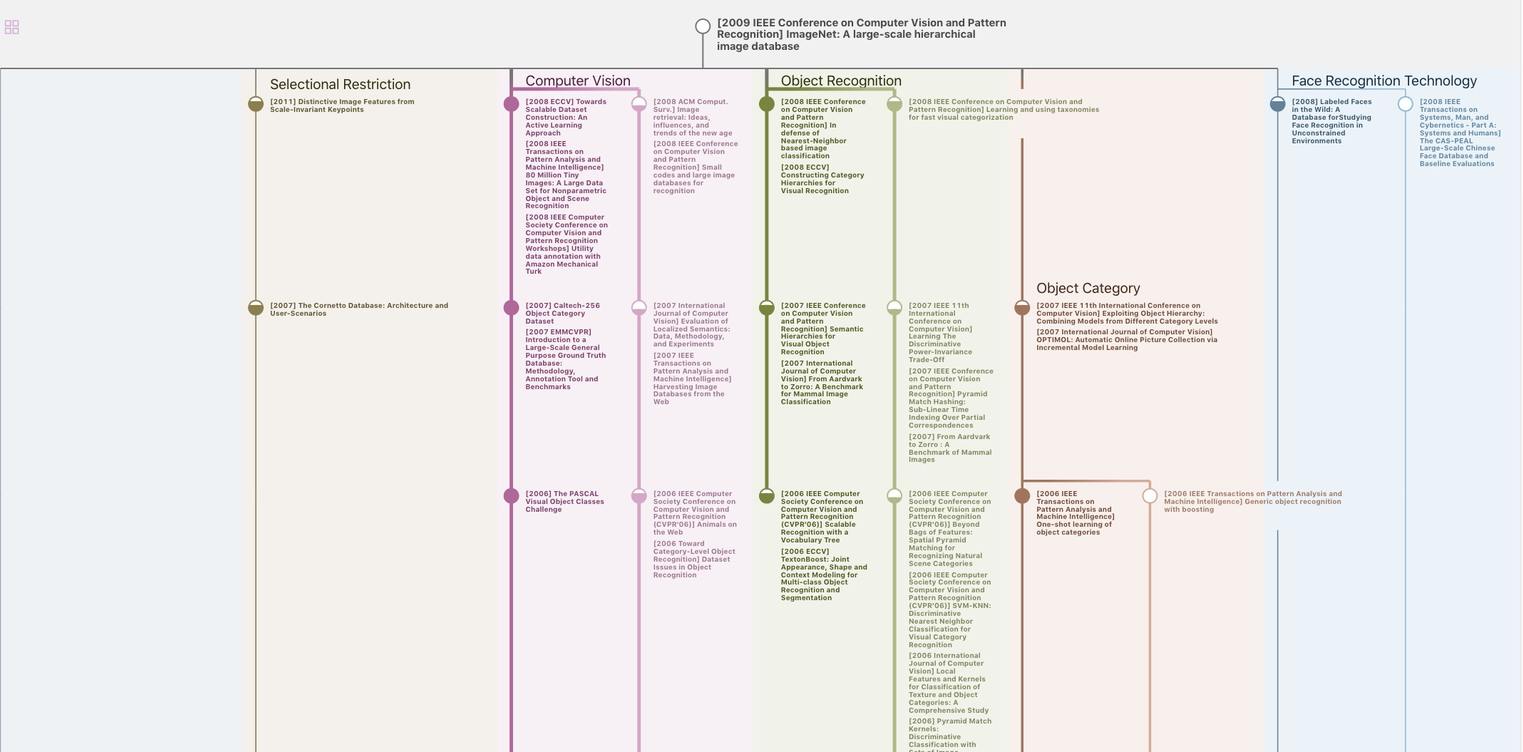
生成溯源树,研究论文发展脉络
Chat Paper
正在生成论文摘要