Target-class guided sample length reduction and training set selection of univariate time-series
Applied Intelligence(2022)
摘要
The novelty/anomaly detection in time-series (TS) data is an admired research domain, which is specifically a one-class classification (OCC) task, where only target-class samples are present during training and the samples from other classes are unavailable. The performance of OCC algorithms depends on quality and quantity of features and training samples because all the features/samples are not equally important for target-class representation. The present research focuses on OCC of univariate time-series (UTS) and proposes a novel way to acquire the knowledge of the target-class to ensure its strong separation from the other class samples. Apart from enormous training samples, the large sample length (span) increases the computing complexities together with its innate problem of curse of “dimensionality” (here, sample span is treated as dimension of time-series). In this context, the present article offers a concurrent way of target-class guided sample span reduction and training sample selection for UTS data. Initially, the vector representation is obtained using state-of-the-art dissimilarity-based representation (DBR) techniques and later, a novel target-class supervised sample span reduction algorithm is offered via Eigenspace analysis to obtain the minimal sample span. Furthermore, to select the most promising training samples as target-class representatives, state-of-the-art prototype methods are utilized. Finally, one-class support vector machine (OCSVM), 1-nearest neighbour (1-NN) and isolation forest (IF) are utilized to evaluate the performance of proposed approach. Intensive experiments are performed over the archive of 85 univariate datasets provided by University of California, Riverside (UCR) and University of East Anglia (UEA) (this repository is also known as UCR/UEA archive).
更多查看译文
关键词
Univariate time-series,One-class classification,Curse of dimensionality,Dissimilarity-based representation,Sample span,Prototype
AI 理解论文
溯源树
样例
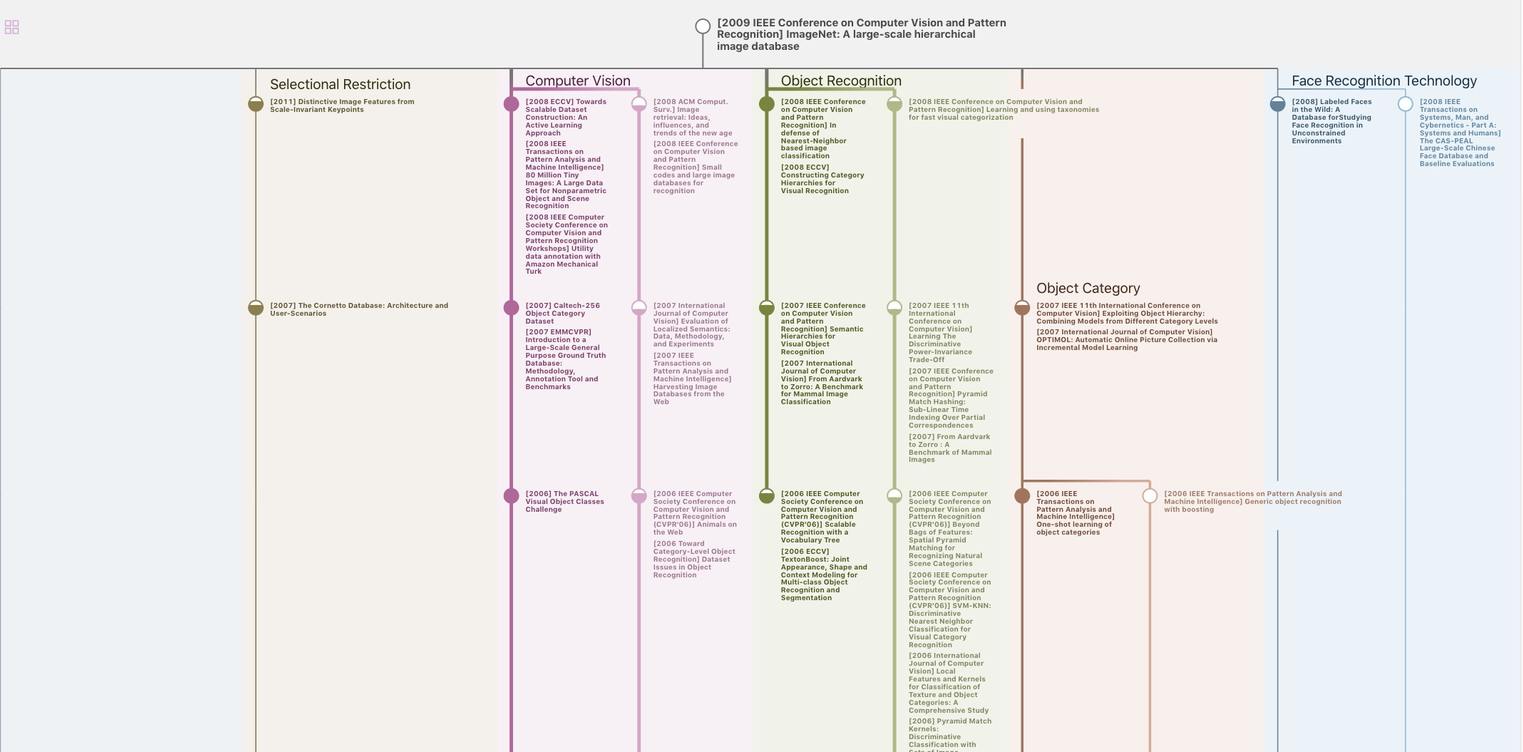
生成溯源树,研究论文发展脉络
Chat Paper
正在生成论文摘要