MaterIA: Single Image High-Resolution Material Capture in the Wild
COMPUTER GRAPHICS FORUM(2022)
摘要
We propose a hybrid method to reconstruct a physically-based spatially varying BRDF from a single high resolution picture of an outdoor surface captured under natural lighting conditions with any kind of camera device. Relying on both deep learning and explicit processing, our PBR material acquisition handles the removal of shades, projected shadows and specular highlights present when capturing a highly irregular surface and enables to properly retrieve the underlying geometry. To achieve this, we train two cascaded U-Nets on physically-based materials, rendered under various lighting conditions, to infer the spatially-varying albedo and normal maps. Our network processes relatively small image tiles (512 x 512 pixels) and we propose a solution to handle larger image resolutions by solving a Poisson system across these tiles. We complete this pipeline with analytical solutions to reconstruct height, roughness and ambient occlusion.
更多查看译文
关键词
Material Capture, SVBRDF, Shadow Removal, Deep Learning, Dataset Synthesis, Delighting
AI 理解论文
溯源树
样例
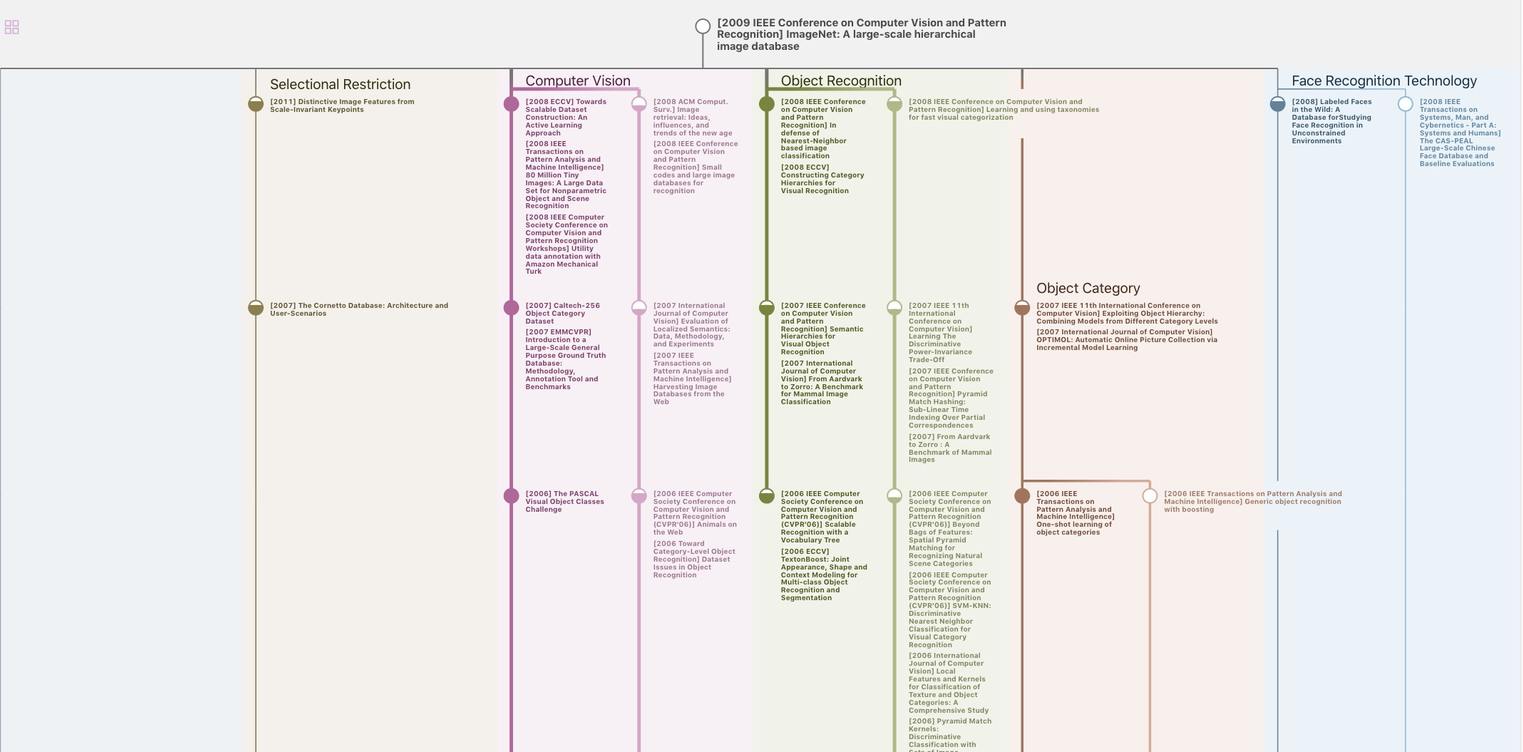
生成溯源树,研究论文发展脉络
Chat Paper
正在生成论文摘要