Why does batch normalization induce the model vulnerability on adversarial images?
WORLD WIDE WEB-INTERNET AND WEB INFORMATION SYSTEMS(2022)
摘要
Batch normalization is one of the most widely used components in deep neural networks. It can accelerate training, and boost model performance on normal samples. However, batch normalization induces vulnerability to models on adversarial examples, especially in medical images, and the reason is still not clear. In this paper, we aim to explain the vulnerability aroused by batch normalization under adversarial images. Specifically, we first discover that both natural and medical images contain a large number of trivial features, whose weights will be enlarged under adversarial attacks, and batch normalization can further enlarge their weights. Additionally, we find that batch normalization will reduce the inter-class margin of high-level features, leading to less tolerance to adversarial perturbations, thereby decreasing the model robustness. Moreover, we hypothesize that the smaller inter-class margin, the more difficult to attain the optimal classification space, which means batch normalization will restrict the performance of adversarial training. This further verifies that a narrower inter-class margin induced by batch normalization reduces the model robustness. Experiments on four benchmark datasets demonstrate our discovery, interpretation and hypothesis.
更多查看译文
关键词
Batch normalization, Adversarial examples, Model robustness, Interpretation
AI 理解论文
溯源树
样例
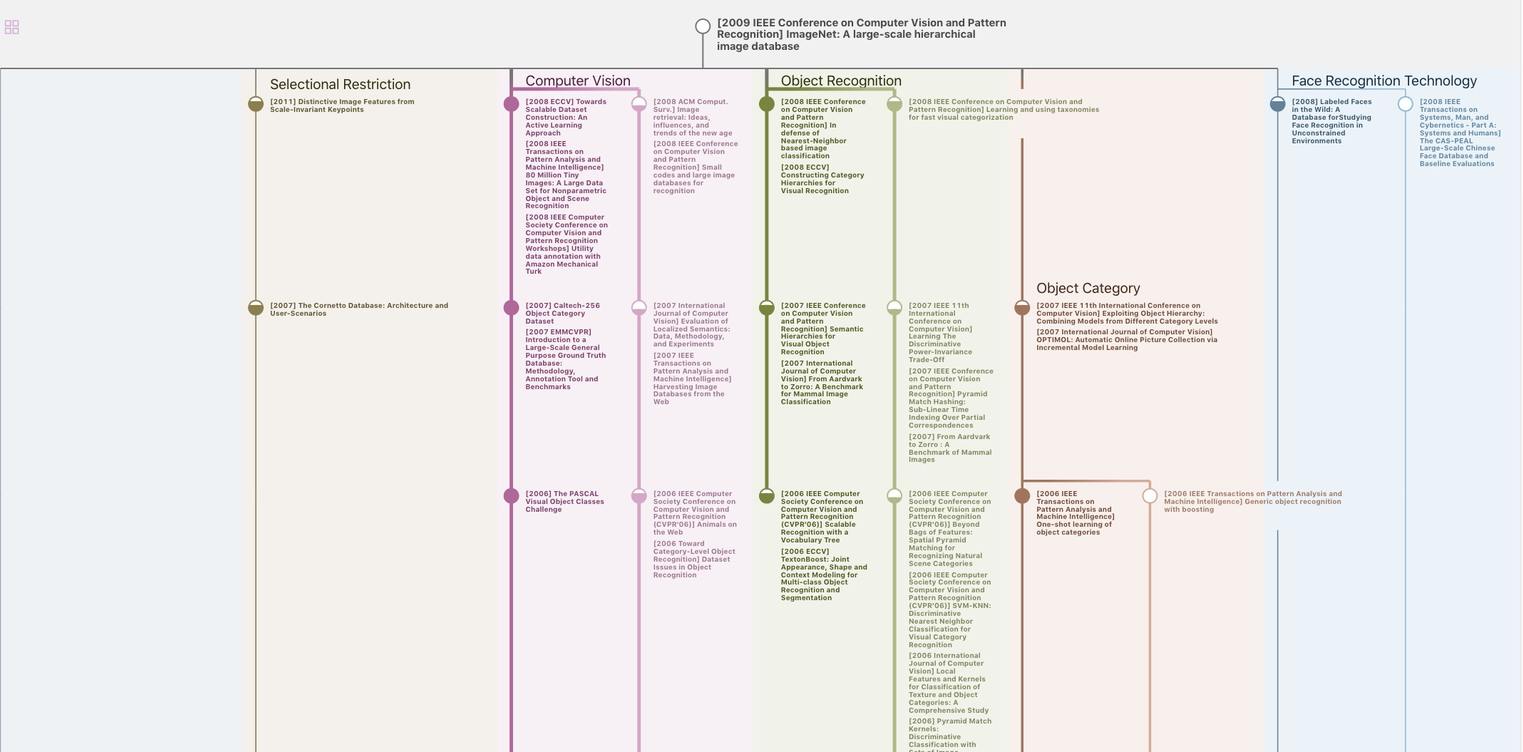
生成溯源树,研究论文发展脉络
Chat Paper
正在生成论文摘要