ALVS: Adaptive Live Video Streaming using deep reinforcement learning
Journal of Network and Computer Applications(2022)
摘要
Achieving a high Quality of Experience (QoE) in live event streaming is a challenging problem given a low-latency requirement and time-varying network conditions. Adaptive video bitrate and adaptive playback speed techniques are two separate control knobs to address this challenge. In this paper, we consider these two control parameters in a joint optimization problem and present a deep reinforcement learning (DRL) framework to maximize QoE for live streaming without any assumption about the environment or fixed rule-based heuristics. With the proposed DRL framework, our approach (ALVS) constructs the inference model to make a joint decision of adaptive playback speed and video quality level for the next video segment. Simulation results through real network traces show that ALVS outperforms both state-of-the-art DRL-based and rule-based algorithms in terms of QoE without sacrificing live latency and skipping any content.
更多查看译文
关键词
Adaptive playback speed,Deep reinforcement learning,Live streaming media and video quality
AI 理解论文
溯源树
样例
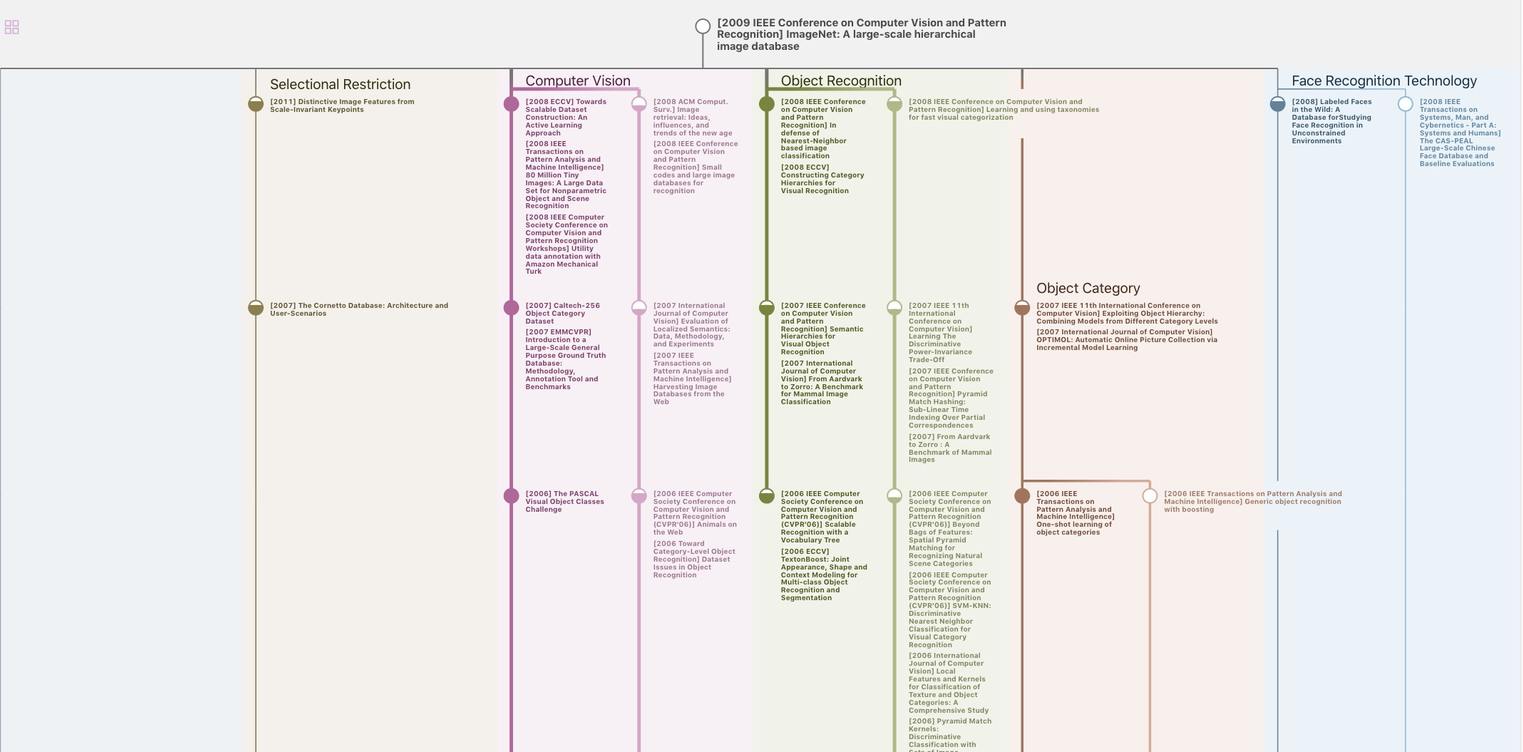
生成溯源树,研究论文发展脉络
Chat Paper
正在生成论文摘要