An approximate dynamic programming approach for solving an air combat maneuvering problem
Expert Systems with Applications(2022)
摘要
Within visual range air combat involves execution of highly complex and dynamic activities, requiring rapid, sequential decision-making to achieve success. Fighter pilots spend years perfecting tactics and maneuvers for these types of combat engagements, yet the ongoing emergence of unmanned, autonomous vehicle technologies elicits a natural question — can an autonomous unmanned combat aerial vehicle (AUCAV) be imbued with the necessary artificial intelligence to perform challenging air combat maneuvering tasks independently? We formulate and solve the air combat maneuvering problem (ACMP) to examine this important question, developing a Markov decision process (MDP) model to control a defending AUCAV seeking to destroy an attacking adversarial vehicle. The MDP model includes a 5-degree-of-freedom, point-mass aircraft state transition model to accurately represent both kinematics and energy while maneuvering. An approximate dynamic programming (ADP) approach is proposed wherein we develop and test an approximate policy iteration algorithm that implements value function approximation via neural network regression to attain high-quality maneuver policies for the AUCAV. A representative intercept scenario is specified for testing purposes wherein the AUCAV must engage and destroy an adversary aircraft attempting to penetrate the defended airspace. Several designed experiments are conducted to determine how aircraft velocity and adversary maneuvering tactics impact the efficacy of the proposed ADP solution approach and to enable efficient algorithm parameter tuning. ADP-generated policies are compared to two benchmark maneuver policies constructed from two reward shaping functions found in the ACMP literature, attaining improved mean probabilities of kill for 24 of 36 air combat situations considered.
更多查看译文
关键词
Air combat strategy,Approximate dynamic programming,Artificial intelligence,Defense,Reinforcement learning
AI 理解论文
溯源树
样例
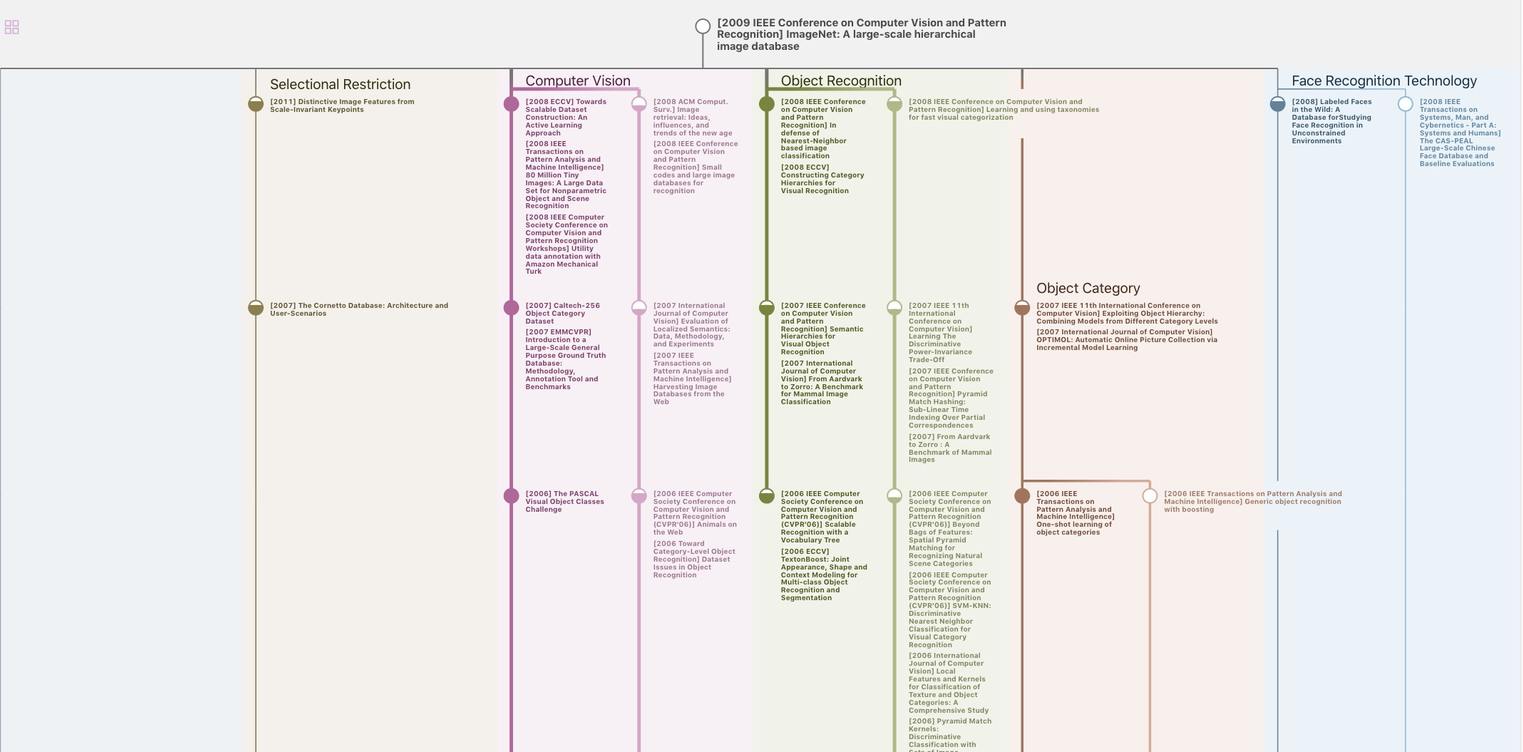
生成溯源树,研究论文发展脉络
Chat Paper
正在生成论文摘要