Discovering probabilistically weighted sequential patterns in uncertain databases
Applied Intelligence(2022)
摘要
Mining useful sequential patterns has been a recent trend in data mining as the real-life applications are mostly sequence oriented. Researchers have developed many algorithms to find frequent sub-sequences from sequential databases to find useful information. The emerging and tremendous development of technology has been increasing the number of applications that deal with uncertainty. Ordinary uncertain pattern mining algorithms deal with expected support or probabilistic frequentness of a pattern, ignoring the importance of individual items. However, in real-life, different items can have different importance. Some approaches consider the weight (importance) of items but fail to capture the interestingness of mined patterns. The objective of the work is to address weighted sequential uncertain pattern mining in Possible World Semantics (PWS) to better capture inherent relations among the items and events with different weights and developing a novel method uWSpan. Our proposed approach contains some pruning techniques to provide faster mining capability and introduces itemset extension for the first time in PWS. We have analyzed the performance of our proposed approach both theoretically and empirically where we found uWSpan efficient, scalable and effective. Our approach outperforms existing approaches most of the time when compared using approved datasets. We also analyzed the applicability, efficiency and effectiveness of our proposed method. Finally, the paper concludes with future research directions and a gist of the outcomes of the research.
更多查看译文
关键词
Uncertain sequential pattern, Probabilistic world, Weighted pattern, Expected support
AI 理解论文
溯源树
样例
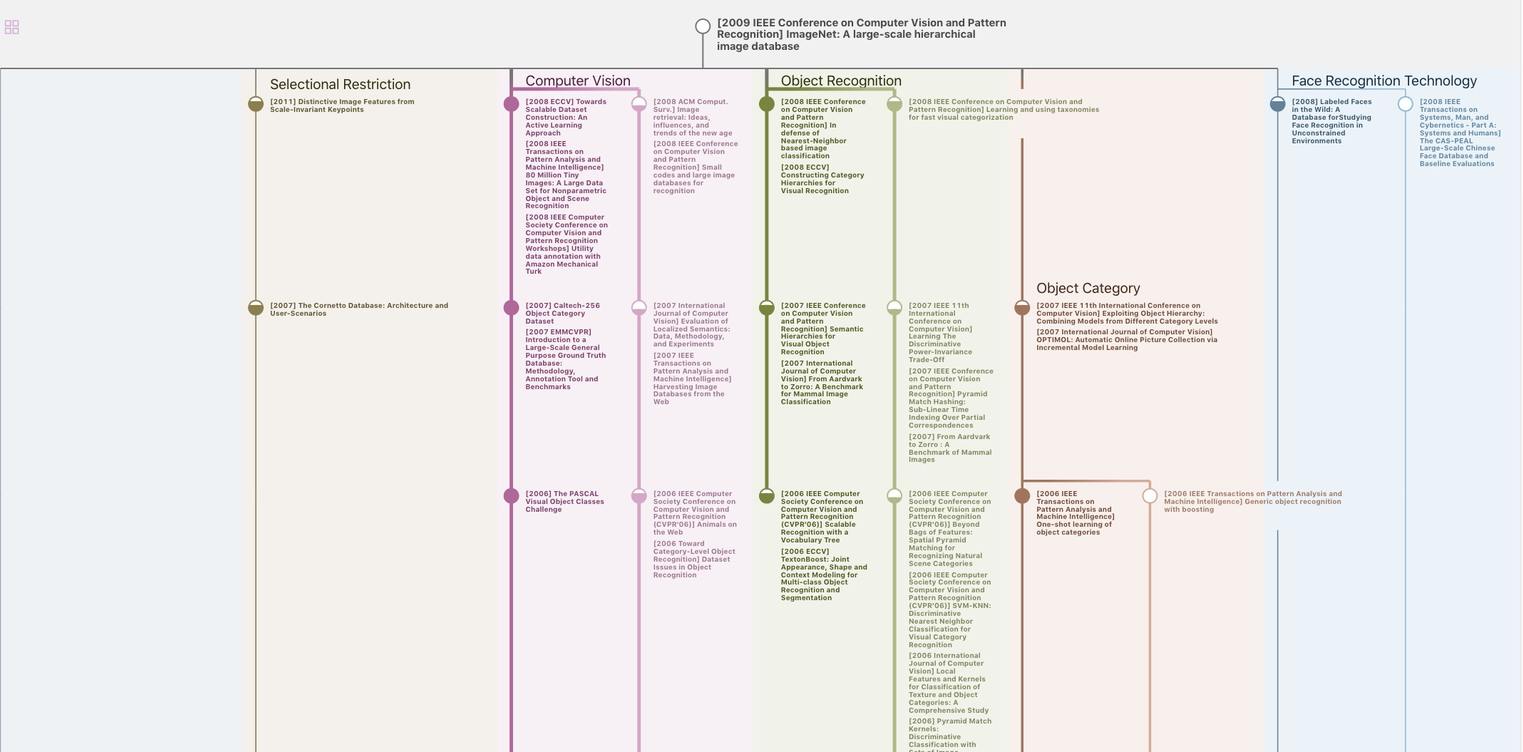
生成溯源树,研究论文发展脉络
Chat Paper
正在生成论文摘要