Controlling StyleGANs using rough scribbles via one-shot learning
COMPUTER ANIMATION AND VIRTUAL WORLDS(2022)
摘要
This paper tackles the challenging problem of one-shot semantic image synthesis from rough sparse annotations, which we call "semantic scribbles." Namely, from only a single training pair annotated with semantic scribbles, we generate realistic and diverse images with layout control over, for example, facial part layouts and body poses. We present a training strategy that performs pseudo labeling for semantic scribbles using the StyleGAN prior. Our key idea is to construct a simple mapping between StyleGAN features and each semantic class from a single example of semantic scribbles. With such mappings, we can generate an unlimited number of pseudo semantic scribbles from random noise to train an encoder for controlling a pretrained StyleGAN generator. Even with our rough pseudo semantic scribbles obtained via one-shot supervision, our method can synthesize high-quality images thanks to our GAN inversion framework. We further offer optimization-based postprocessing to refine the pixel alignment of synthesized images. Qualitative and quantitative results on various datasets demonstrate improvement over previous approaches in one-shot settings.
更多查看译文
关键词
GAN inversion, generative adversarial networks, image editing
AI 理解论文
溯源树
样例
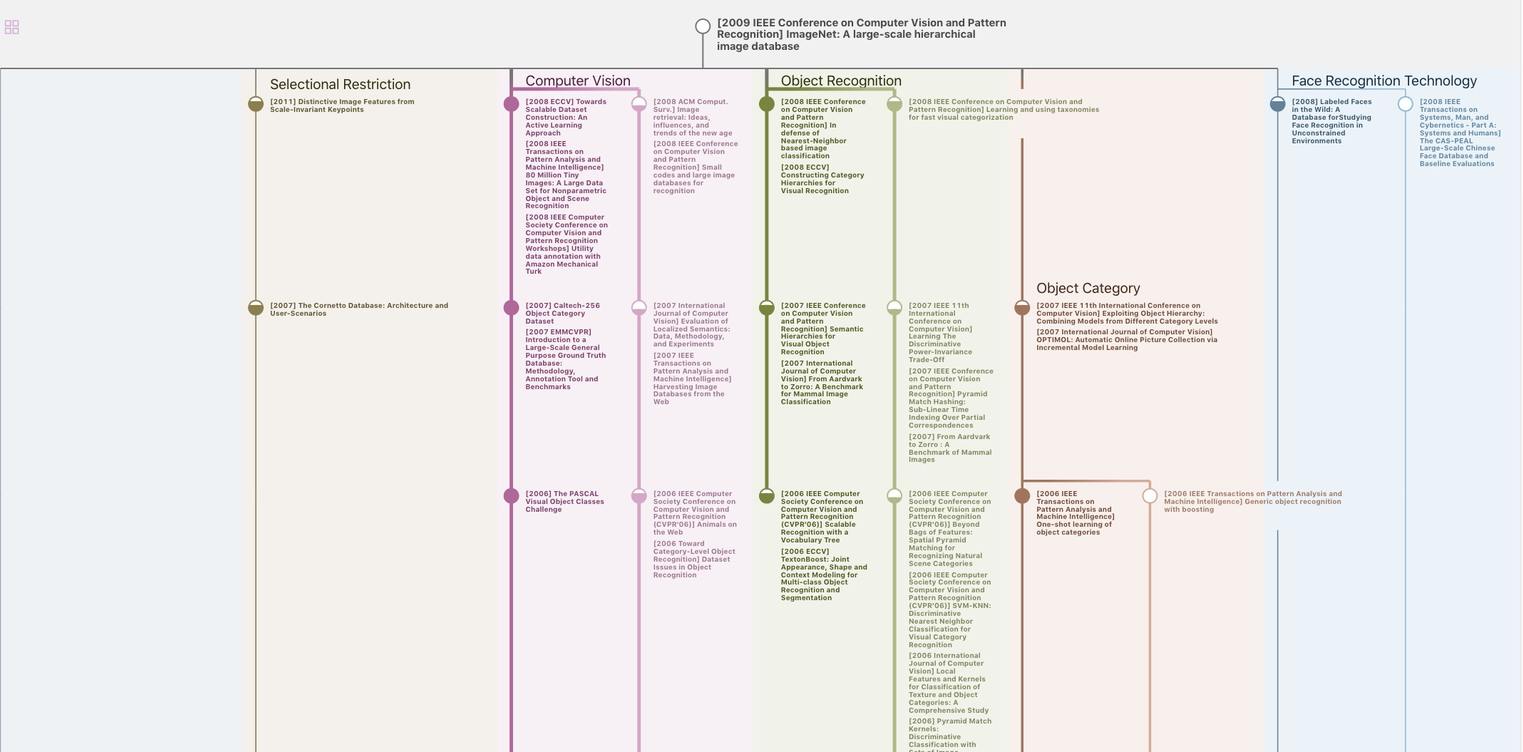
生成溯源树,研究论文发展脉络
Chat Paper
正在生成论文摘要