Lightweight multi-scale convolutional neural network for real time stereo matching
Image and Vision Computing(2022)
摘要
In order to accurately estimate disparities in textureless and slim regions, spatial pyramid pooling and stacked 3D CNN, which can capture global context information, are widely used in state-of-the-art stereo matching algorithms. Unfortunately, the computational complexity and high memory consumption make these methods not friendly to real-time applications such as autonomous driving and augmented realities. In order to balance the real-time performance and accuracy, we design lightweight multi-scale convolutional neural network for real-time stereo matching. First, Lightweight multi-scale 2D and 3D CNN modules are proposed for feature extraction and initial disparity computation respectively. Both of above modules only run on a low resolution to further reduce the amount of calculation. Second, multi-scale RGB images guided network is utilized to refine the final disparity estimation. Experiments on several datasets show that the proposed algorithm can achieve competitive results with speed of 64fps on a NIVDIA 1080 GPU.
更多查看译文
关键词
Stereo matching,Multi-scale,Refinement,Lightweight
AI 理解论文
溯源树
样例
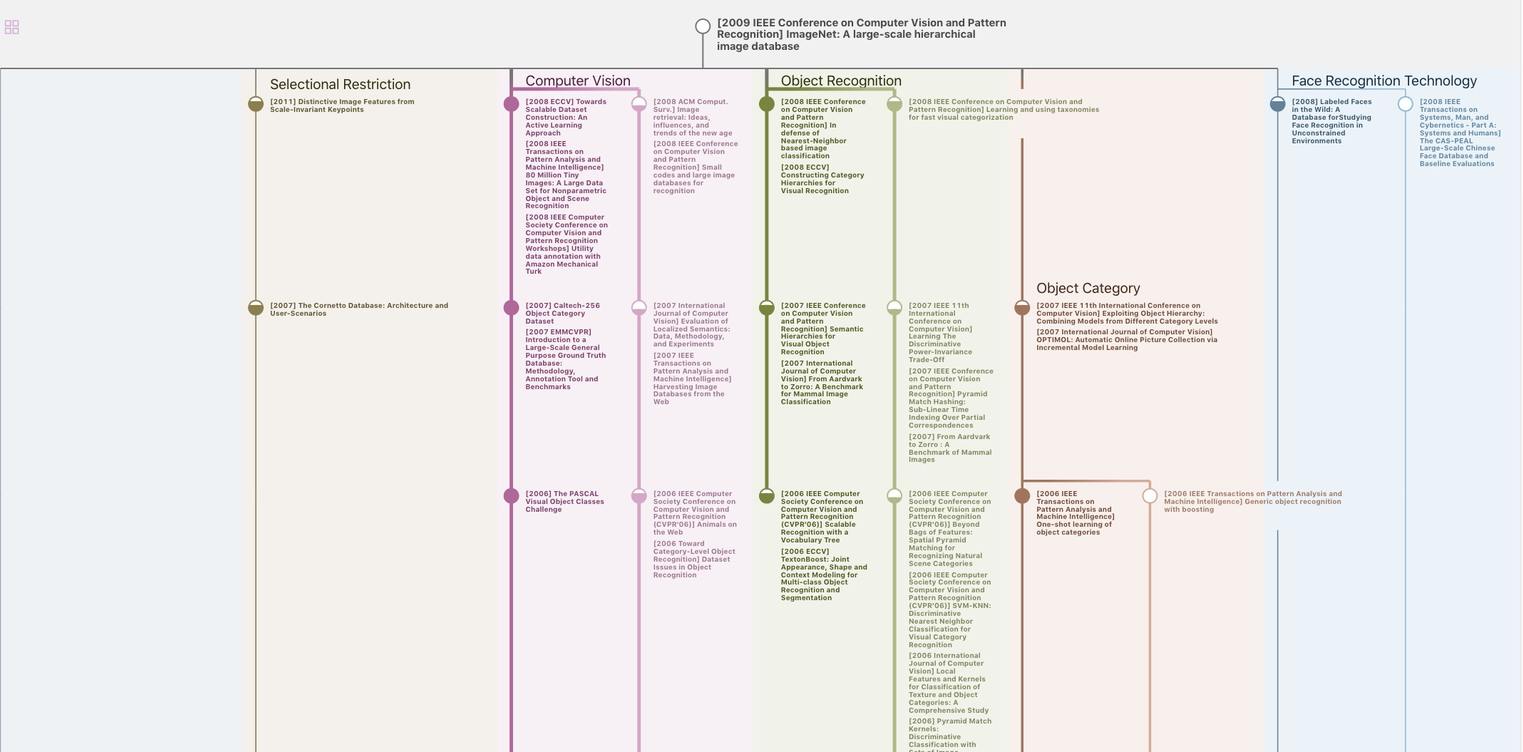
生成溯源树,研究论文发展脉络
Chat Paper
正在生成论文摘要