Artificial Neural Networks for the Prediction of the Reference Evapotranspiration of the Peloponnese Peninsula, Greece
WATER(2022)
摘要
The aim of the study was to investigate the utility of artificial neural networks (ANNs) for the estimation of reference evapotranspiration (ETo) on the Peloponnese Peninsula in Greece for two representative months of wintertime and summertime during 2016-2019 and to test if using fewer inputs could lead to satisfactory predictions. Datasets from sixty-two meteorological stations were employed. The available inputs were mean temperature (Tmean), sunshine (N), solar radiation (Rs), net radiation (Rn), vapour pressure deficit (es-ea), wind speed (u(2)) and altitude (Z). Nineteen Multi-layer Perceptron (MLP) and Radial Basis Function (RBF) models were tested and compared against the corresponding FAO-56 Penman Monteith (FAO PM) estimates of a previous study, via statistical indices. The MLP1 7-2 model with all the variables as inputs outperformed the rest of the models (RMSE = 0.290 mm d(-1), R-2 = 98%). The results indicate that even ANNs with simple architecture can be very good predictive models of ETo for the Peloponnese, based on the literature standards. The MLP1 model determined Tmean, followed by u(2,) as the two most influential factors for ETo. Moreover, when one input was used (Tmean, Rn), RBFs slightly outperformed MLPs (RMSE < 0.385 mm d(-1), R-2 >= 96%), which means that even a sole-input ANN resulted in satisfactory predictions of ETo.
更多查看译文
关键词
multilayer perceptron, MLP, radial basis function, RBF, ANN, neural networks, reference evapotranspiration, Peloponnese, Greece
AI 理解论文
溯源树
样例
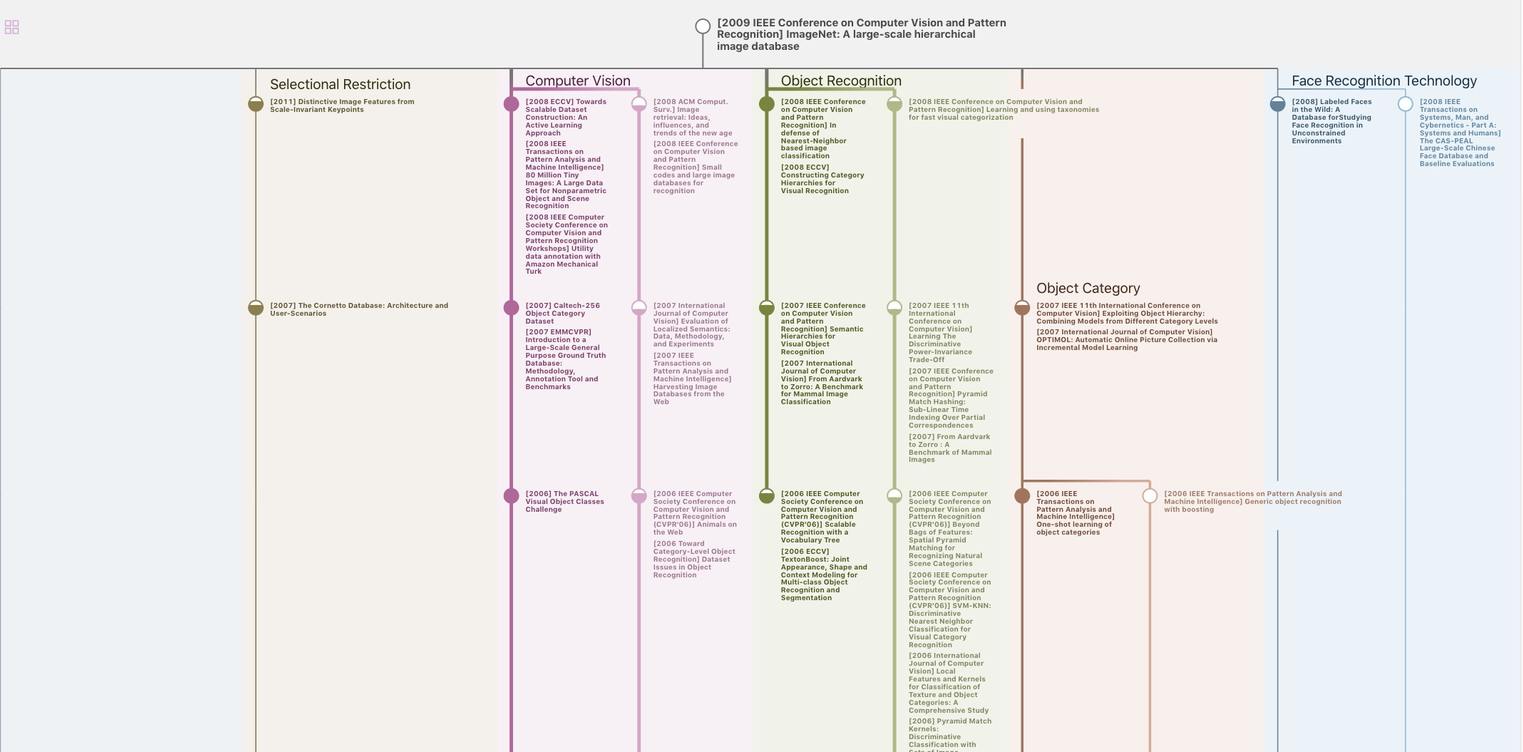
生成溯源树,研究论文发展脉络
Chat Paper
正在生成论文摘要