Error-Bounded Learned Scientific Data Compression with Preservation of Derived Quantities
APPLIED SCIENCES-BASEL(2022)
摘要
Scientific applications continue to grow and produce extremely large amounts of data, which require efficient compression algorithms for long-term storage. Compression errors in scientific applications can have a deleterious impact on downstream processing. Thus, it is crucial to preserve all the "known" Quantities of Interest (QoI) during compression. To address this issue, most existing approaches guarantee the reconstruction error of the original data or primary data (PD), but cannot directly control the problem of preserving the QoI. In this work, we propose a physics-informed compression technique that is composed of two parts: (i) reduction of the PD with bounded errors and (ii) preservation of the QoI. In the first step, we combine tensor decompositions, autoencoders, product quantizers, and error-bounded lossy compressors to bound the reconstruction error at high levels of compression. In the second step, we use constraint satisfaction post-processing followed by quantization to preserve the QoI. To illustrate the challenges of reducing the reconstruction errors of the PD and QoI, we focus on simulation data generated by a large-scale fusion code, XGC, which can produce tens of petabytes in a single day. The results show that our approach can achieve a high compression amount while accurately preserving the QoI within scientifically acceptable bounds.
更多查看译文
关键词
data compression, autoencoders, error guarantees, moment preservation, constraint satisfaction, quantization, fusion application
AI 理解论文
溯源树
样例
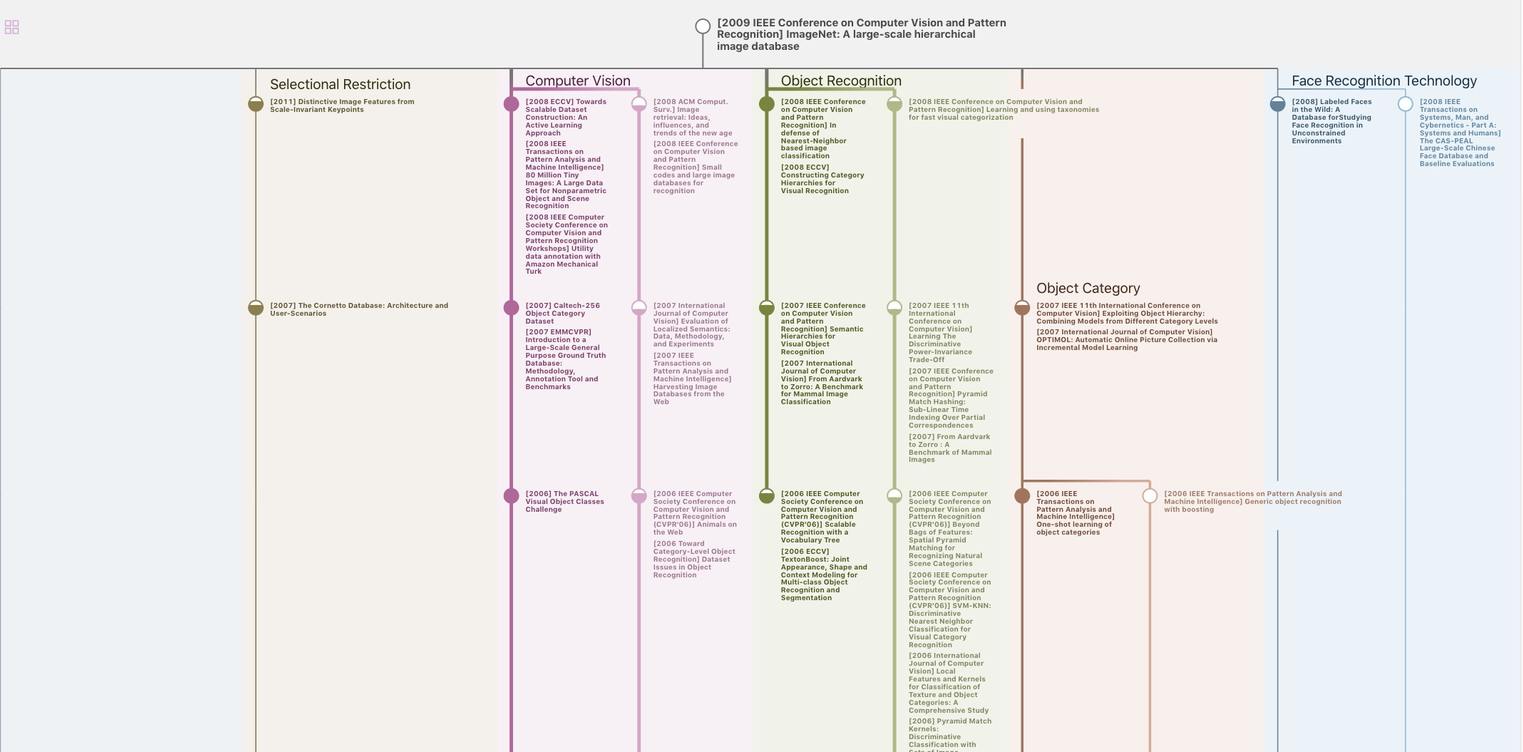
生成溯源树,研究论文发展脉络
Chat Paper
正在生成论文摘要