Prediction of effective stimulated reservoir volume after hydraulic fracturing utilizing deep learning
PETROLEUM SCIENCE AND TECHNOLOGY(2023)
摘要
It is very important to utilize hydraulic fracturing for unconventional reservoir development. Accurate prediction of effective stimulated reservoir volume (SRV) after fracturing facilitates production evaluation. However, the traditional methods to predict SRV are time consuming and the precision cannot fully meet the requirements. To overcome the shortcomings, a new approach was presented using deep learning which includes four procedures. Firstly, the datasets were collected by numerical simulation considering non-Darcy flow characteristics. Additionally, the Branched Deep Neural Network model (B-DNN) was established after data fusion through adding a branch neural network. Then the optimal hyperparameters were obtained after adjusting to satisfy model accuracy and reliability. Finally, the prediction results of B-DNN and convolutional neural network (CNN) and recurrent neural network (RNN) were compared. The results show that the model with Softplus activation function, four hidden layers, and 250 neurons in each layer would have the best calculation results. The proposed model has good agreement with actual field data which can reach 97%. Furthermore, compared with the CNN and RNN models, it is shown that the B-DNN model has considerable prediction accuracy and is less time-consuming. This deep learning model provides new insight for production evaluation in the fractured tight oil reservoir.
更多查看译文
关键词
Deep learning,effective stimulated reservoir volume,hydraulic fracturing,hyperparameter evaluation,tight oil
AI 理解论文
溯源树
样例
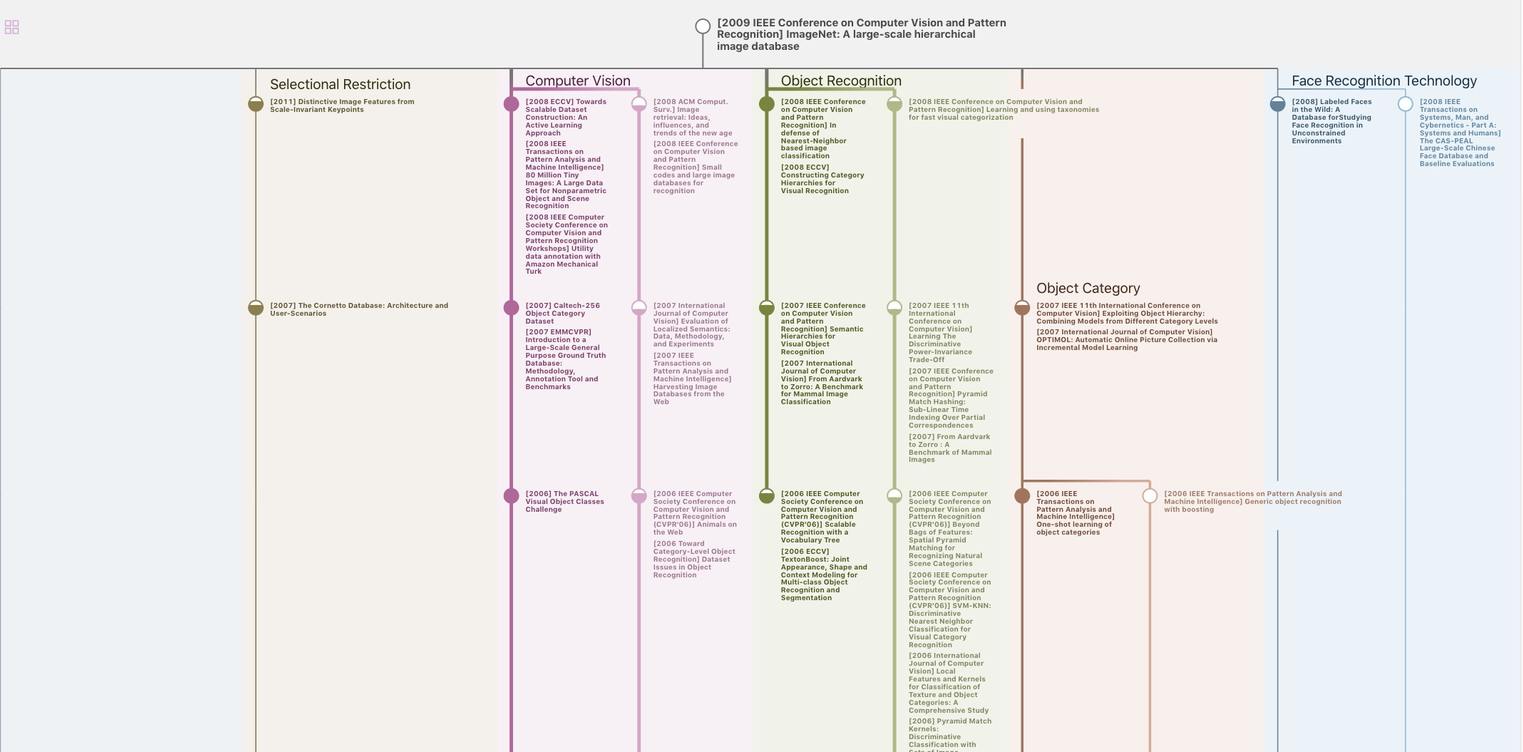
生成溯源树,研究论文发展脉络
Chat Paper
正在生成论文摘要