Predicting Dielectric Constant of Polyimide Composites Using Data-Driven Wavelet Kernel Deep Gaussian Process
IEEE Transactions on Dielectrics and Electrical Insulation(2022)
摘要
Materials design with the assistant of data-driven methods has attracted increasing attention. For the design and preparation of polyimide (PI) composites with different dielectric constants, traditional trial and error methods and existing data-driven methods still have difficulty meeting the accuracy requirements of engineering. Herein, a Morlet wavelet kernel deep Gaussian process regression (MWDGPR) method is proposed to predict the dielectric constant of PI composites filled with different nanoparticles; 40 PI-based composite films containing six different series fillers at various content are prepared, and the dielectric constant of prepared films are measured at 10
0
–10
7
Hz, to construct a dataset. Then, the MWDGPR model is constructed. The results show that the proposed model possesses excellent prediction performance, and the
${R}^{2}$
value is 0.9677, and the mean square error (MSE) value is 0.0323. Compared with other methods, the MWDGPR method has better generalization performance. It is expected that the method reported in this article could overcome the difficulty in measuring the dielectric constant of PI composites under extreme circumstance such as high-temperature field or high electric field in the future, as well as provide a new guideline in designing PI-based composites with proper dielectric constant.
更多查看译文
关键词
Deep Gaussian process (DGP),dielectric constant prediction,machine learning,polyimide (PI) nanocomposites,wavelet kernel function
AI 理解论文
溯源树
样例
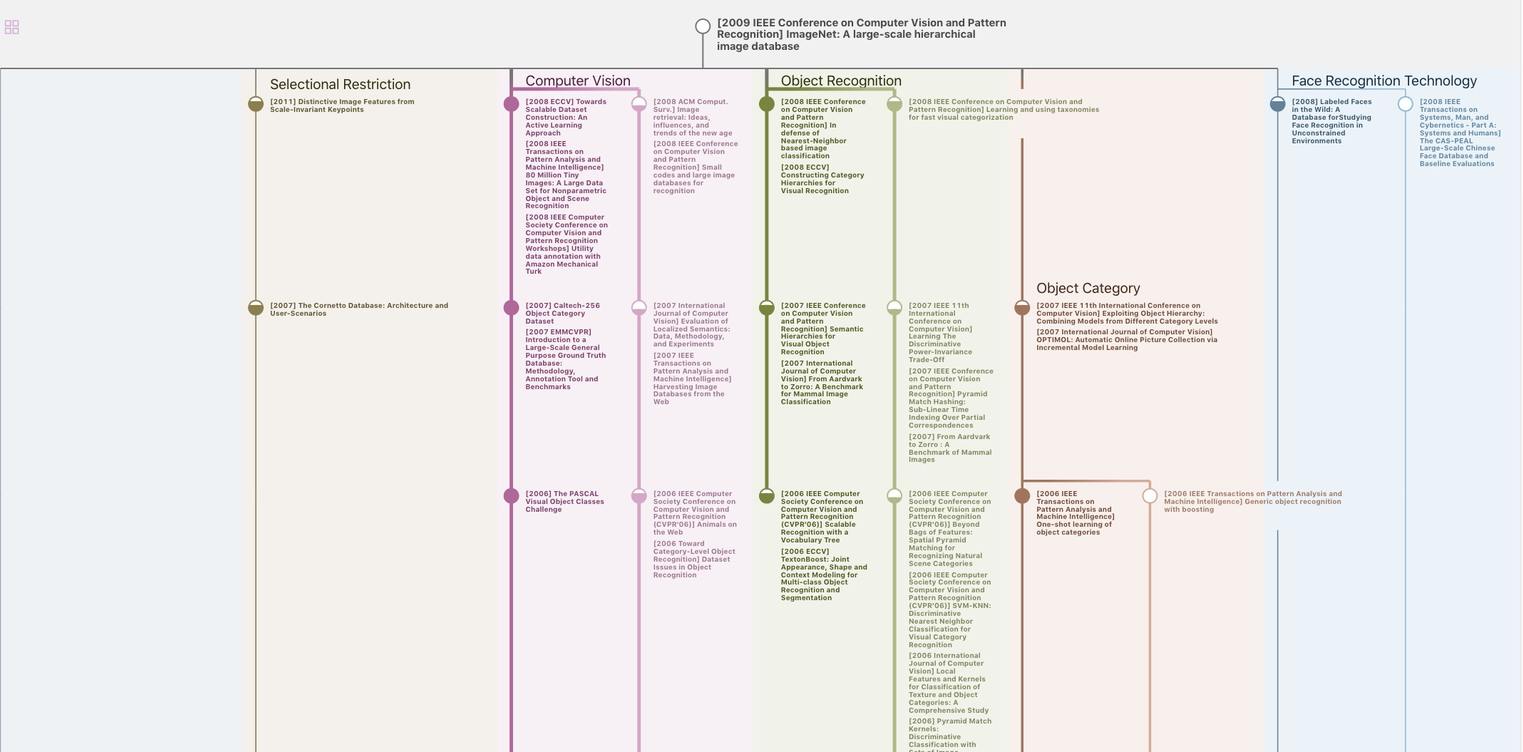
生成溯源树,研究论文发展脉络
Chat Paper
正在生成论文摘要