A bi-objective k-nearest-neighbors-based imputation method for multilevel data
Expert Systems with Applications(2022)
摘要
We propose a bi-objective algorithm based on the k-nearest neighbors (biokNN) method to perform imputation of missing values for data with multilevel structures with continuous variables. We define the imputation method as a bi-objective minimization problem and propose a solution algorithm based on a weighted objective function. The algorithm seeks imputed values that balance the dissimilarity between the k-nearest neighbors and the observations within the same cluster. The effectiveness of the proposed method is evaluated through a simulation study, and its results are compared with those of eight benchmark imputation methods. The simulation study is based on the generation of datasets with a varying-intercept–varying-slope multilevel model, and the results are compared both by using well-known accuracy metrics and by estimating the bias of the estimates after inference has been performed. Based on the simulation, the effects of different configurations of multilevel datasets are tested, including the number of clusters, their size, their similarity, the percentage of missing values, and the effect of imbalanced clusters. The results show that the proposed method outperforms the benchmark methods, especially in cases with high intraclass correlation. A comparison of fitted linear multilevel regression models shows that our method can also reduce the bias of the estimates and the coefficient of determination. Finally, the method is tested on three commonly used machine learning datasets and shows better accuracy in most cases compared with the benchmark methods.
更多查看译文
关键词
Multilevel data,Imputation,k-nearest neighbors
AI 理解论文
溯源树
样例
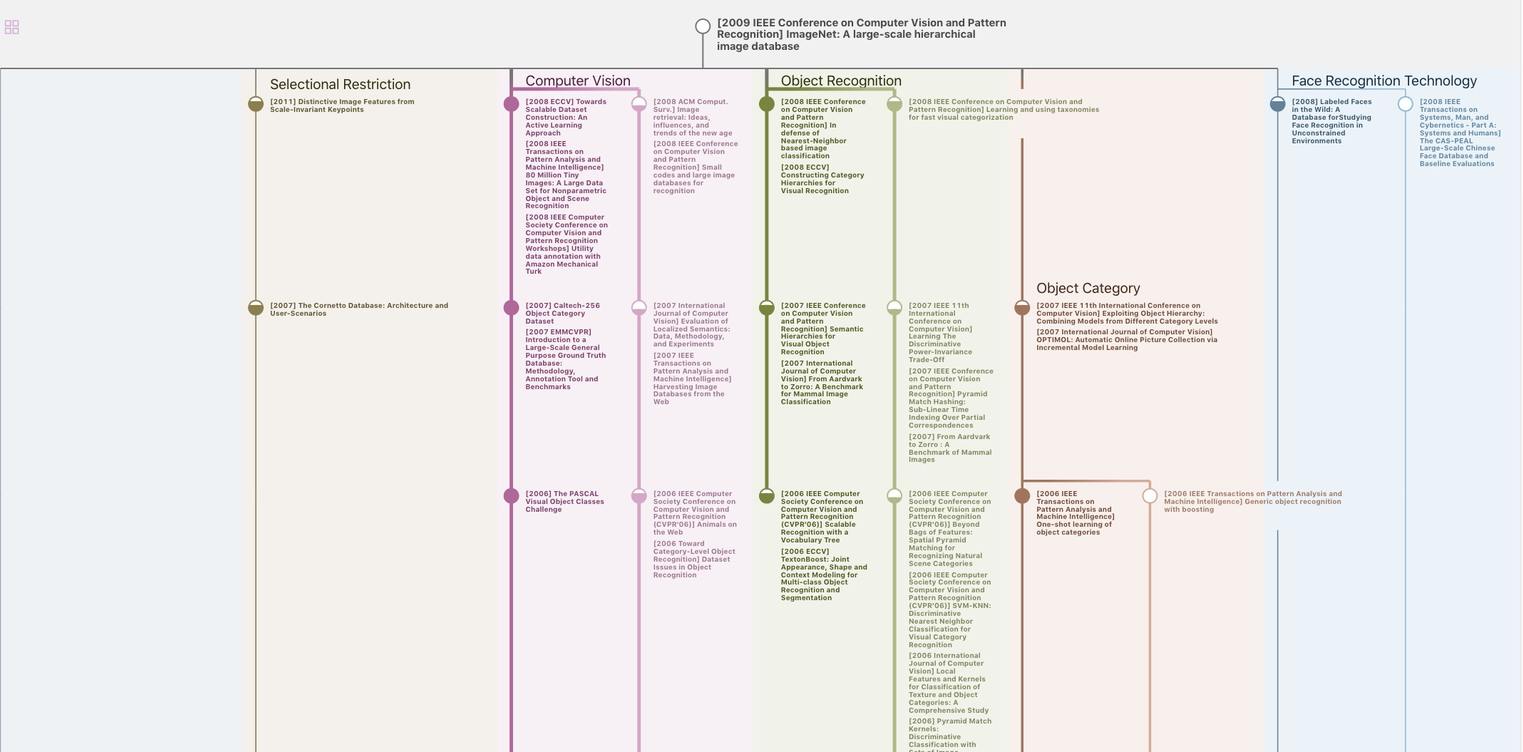
生成溯源树,研究论文发展脉络
Chat Paper
正在生成论文摘要