Effectiveness Evaluation of Evasion Attack on Encrypted Malicious Traffic Detection
2022 IEEE WIRELESS COMMUNICATIONS AND NETWORKING CONFERENCE (WCNC)(2022)
摘要
With more and more TLS encrypted traffic on the Internet, an increasing amount of malware is using TLS to hide their tracks. The encrypted traffic makes the traditional malicious traffic detection methods invalid. Machine learning algorithms have become essential options for detecting encrypted malicious traffic. Recently, researchers found that machine learning algorithms have flaws, and threat actors can use some tricks to evade detection. But it remains an open question on how these machine learning-based encrypted malicious traffic detection algorithms perform in the face of evasion attacks.We explore the answer in this paper. We first define five mutation rules to generate adversarial examples. With these mutation rules, we can evaluate the ability of several detection algorithms to deal with evasion attacks when detecting encrypted malicious traffic. The encrypted malicious traffic collected for 12 months is used for experiments. Experiments show that modifying the destination port can reduce the detection rate of detection algorithms in feature space, except for random forest algorithms. Inserting junk data has minimal effect on these algorithms. Whether in the problem space or feature space, inserting useless cipher suites and simulating browser’s traffic can significantly reduce the detection rate of these algorithms. When simulating browser’s traffic, the random forest algorithm almost loses its usability. The same situation arises when SVM is faced with inserting useless cipher suites. Compared with inserting useless cipher suites, inserting useless extensions has a minor effect on these algorithms. Our findings will contribute to future research on encrypted malicious traffic detection.
更多查看译文
关键词
Evasion Attack, Adversarial Machine Learning, Encrypted Malicious Traffic, Network Security
AI 理解论文
溯源树
样例
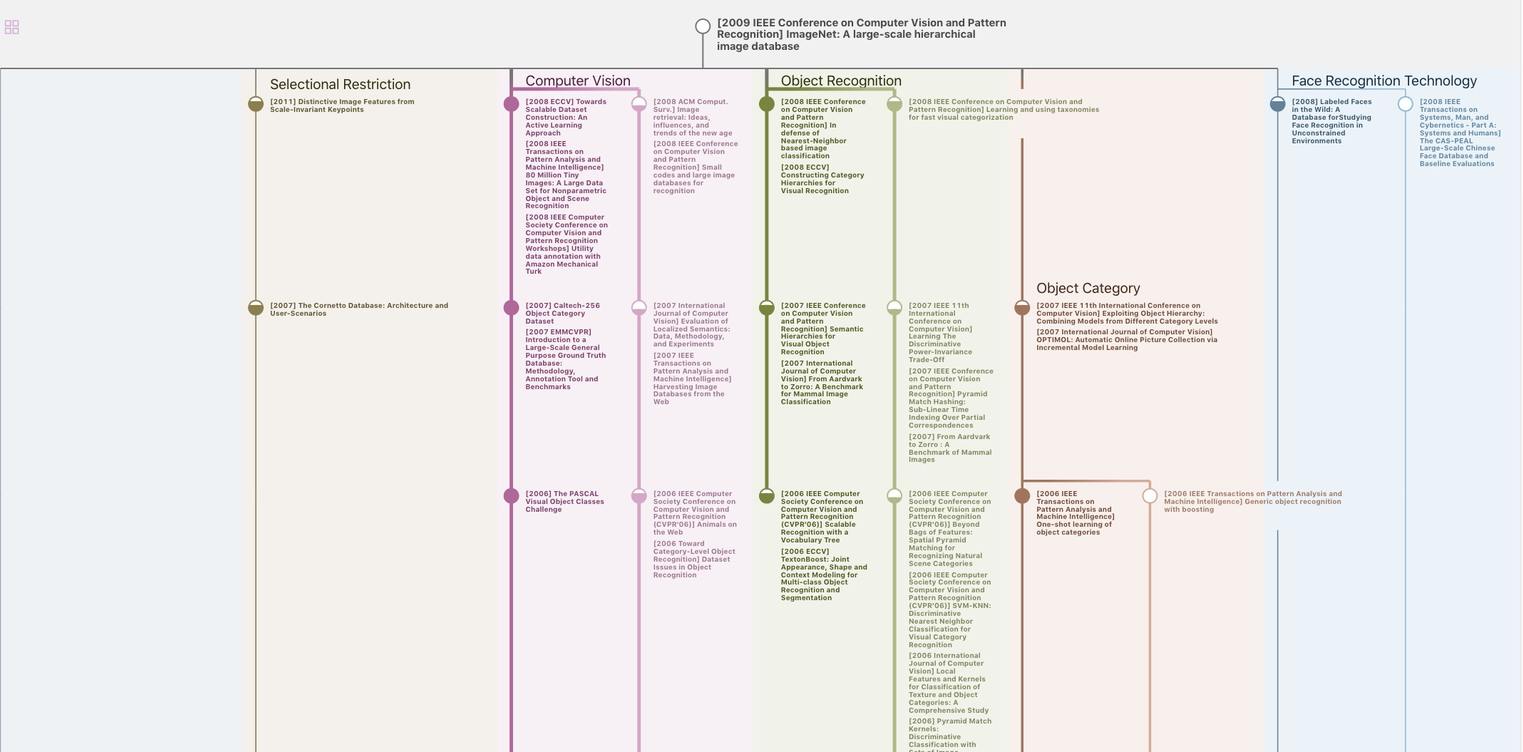
生成溯源树,研究论文发展脉络
Chat Paper
正在生成论文摘要