Multi-Commodity Flow Routing for Large-Scale LEO Satellite Networks Using Deep Reinforcement Learning
2022 IEEE WIRELESS COMMUNICATIONS AND NETWORKING CONFERENCE (WCNC)(2022)
摘要
With the explosive growth of low earth orbit (LEO) satellite networks, such as Starlink, satellite communication has lower latency and can achieve high-speed transmission than before. However, the time-variant topology during all network lifetimes makes the routing problem in the LEO satellite networks challenging. Therefore, in this paper, we propose the deep reinforcement learning-based satellite routing (DRL-SR) method to tackle the multi-commodity flow routing problem in the LEO satellite networks. Given the current state of the satellite network environment, the satellite operation center will determine how to route the requests to the matching destinations. Particularly, the single agent in our DRL-SR approach can determine the multiple next hops as actions for all the corresponding requests each timeslot. Finally, simulation results show that our proposed algorithm yields lower latency than the shortest path approach.
更多查看译文
关键词
LEO satellite networks, satellite routing, multi-commodity flow, deep reinforcement learning
AI 理解论文
溯源树
样例
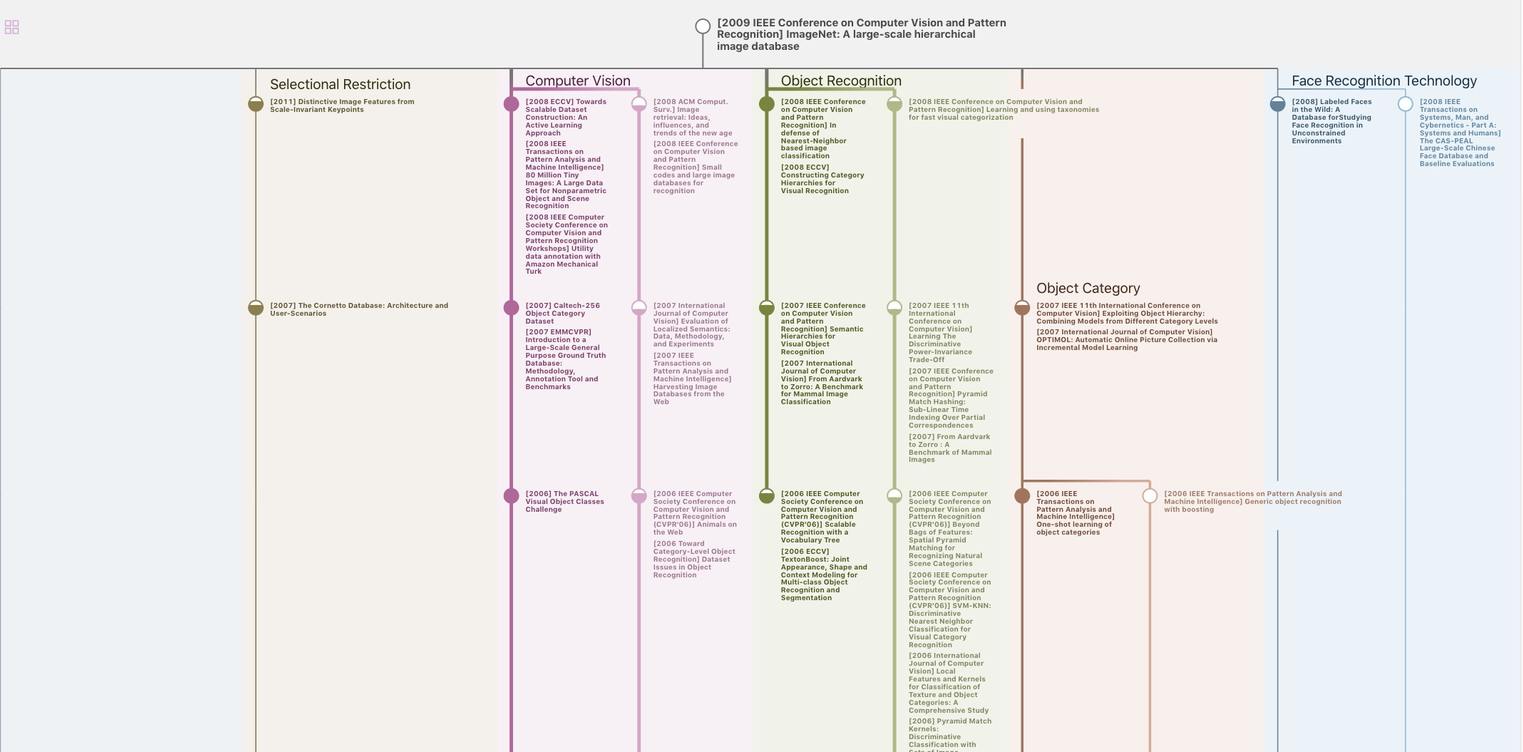
生成溯源树,研究论文发展脉络
Chat Paper
正在生成论文摘要