Asynchronous Federated Learning Empowered Computation Offloading in Collaborative Vehicular Networks
2022 IEEE WIRELESS COMMUNICATIONS AND NETWORKING CONFERENCE (WCNC)(2022)
摘要
Collaborative vehicular networks (CVNs) provide on-demand data processing via computation offloading empowered by edge and fog computing. However, intelligent computation offloading in CVNs still faces several challenges such as long-term quality of service (QoS) guarantee, inefficient utilization of environmental information, and asynchronous information exchange. In this paper, we aim at maximizing the throughput under long-term QoS constraints such as queuing delay. The problem of server-side computation resource allocation and user vehicle (UV)-side task offloading is decoupled by Lyapunov optimization. Firstly, we propose an asynchronous federated deep Q-learning network based task offloading (AF-DQN) algorithm to solve the task offloading subproblem by exploring the semi-distributed learning framework. Secondly, we develop a heuristic queue backlog-aware algorithm to solve the computation resource allocation subproblem. Simulation results demonstrate that the proposed algorithm effectively reduces end-to-end queuing delay.
更多查看译文
关键词
Vehicular networks, computation offloading, AF-DQN, Lyapunov optimization
AI 理解论文
溯源树
样例
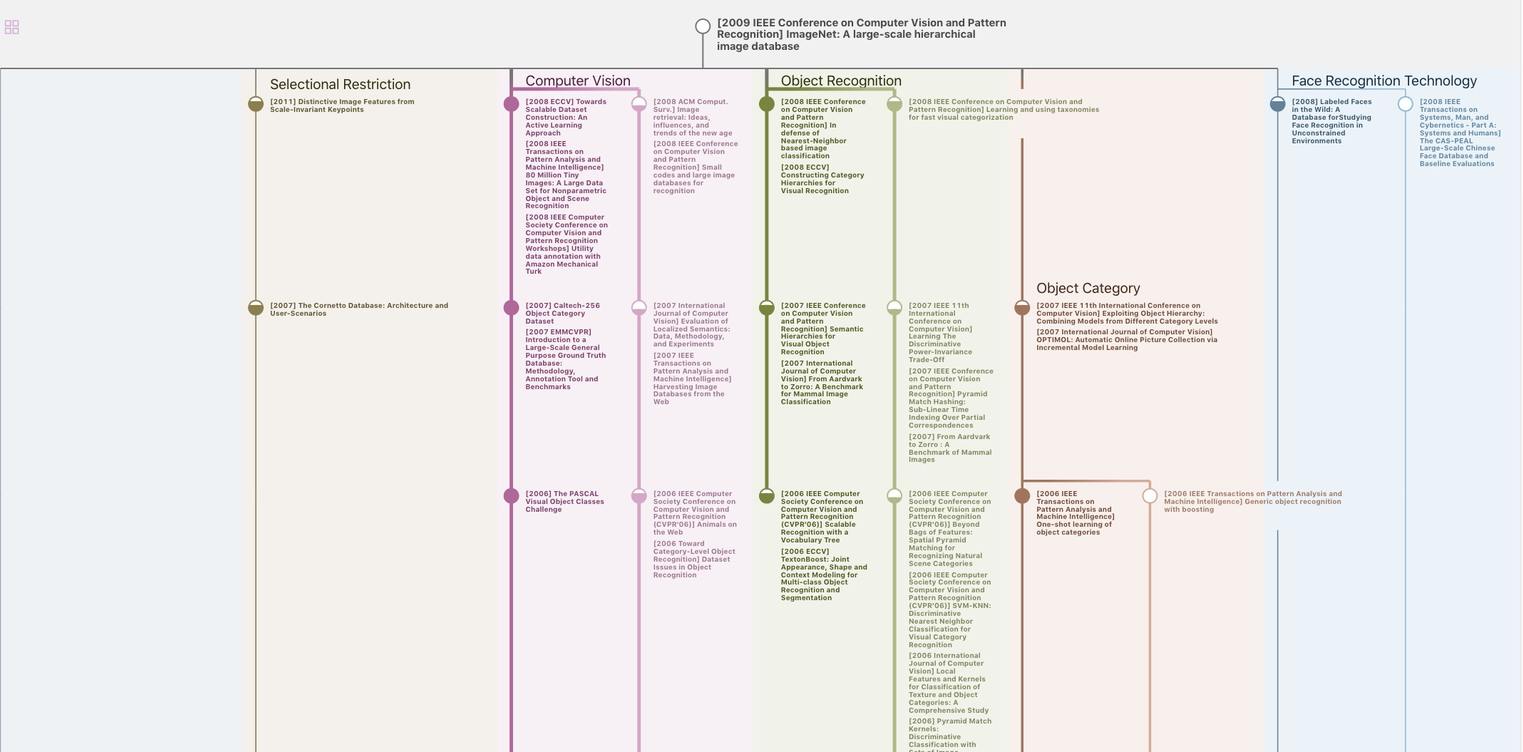
生成溯源树,研究论文发展脉络
Chat Paper
正在生成论文摘要