Combining Remote and In-situ Sensing for Persistent Monitoring of Water Quality
OCEANS 2022(2022)
摘要
Many studies suggest that water quality parameters can be estimated by applying statistical and machine learning methods using remote sensing or in-situ data. However, identifying best practices for implementing solutions appears to be done on a case-by-case basis. In our case, we have in-situ data that covers a large period, but only small areas of Biscayne Bay, Florida. In this paper, we combine available in-situ data with remote sensing data captured by Landsat 8 OLI-TIRS Collection 2 Level 2(L8), Sentinel-2 L2A(S2), and Sentinel-3 OLCI L1B(S3). The combined data set is for use in a water quality parameter estimation application. Our contributions are two-fold. First, we present a pipeline for data collection, processing, and co-location that results in a usable data set of combined remote sensing and in-situ data. Second, we propose a classification model using the combined data set to identify areas of interest for future data collection missions based on chlorophyll-a in-situ measurements. To further prove our methodology, we conduct a data collection mission using one of the predicted paths from our model.
更多查看译文
关键词
remote sensing, machine learning, water quality, logistic regression, estimation, robots, chlorophyll-a
AI 理解论文
溯源树
样例
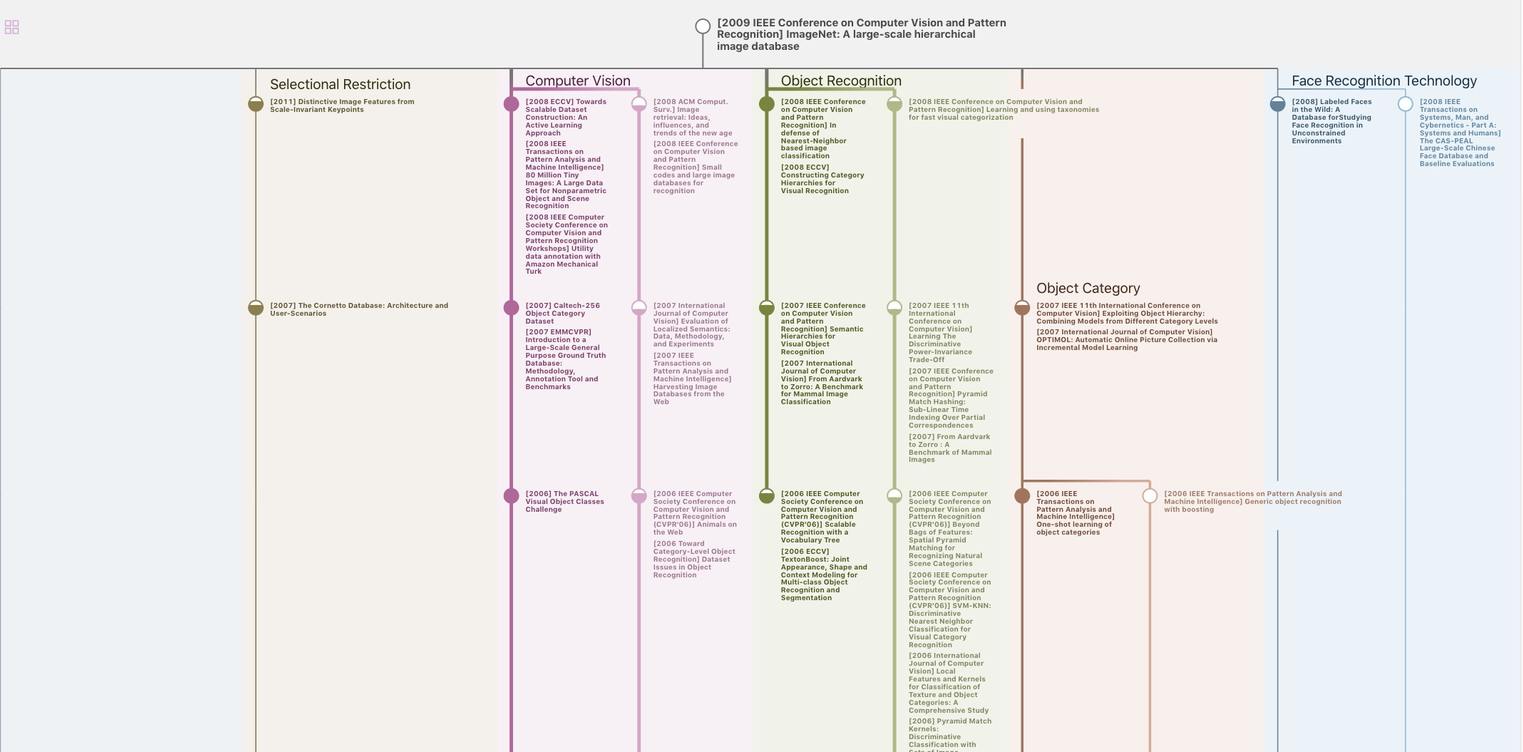
生成溯源树,研究论文发展脉络
Chat Paper
正在生成论文摘要