Thorough Descriptor Search to Machine Learn the Lattice Thermal Conductivity of Half-Heusler Compounds
ACS APPLIED ENERGY MATERIALS(2022)
摘要
Predicting the lattice thermal conductivity (kappa(L)) of compounds prior to synthesis is an extremely challenging task because of complexity associated with determining the phonon scattering lifetimes for underlying normal and Umklapp processes. An accurate ab initio prediction is computationally very expensive, and hence one seeks for data-driven alternatives. We perform machine learning (ML) on theoretically computed kappa(L) of half-Heusler (HH) compounds. An exhaustive descriptor list comprising elemental and compound descriptors is used to build several ML models. We find that ML models built with compound descriptors can reach high accuracy with a fewer number of descriptors, while a set of a large number of elemental descriptors may be used to tune the performance of the model as accurately. Thereby, using only the elemental descriptors, we build a model with exceptionally high accuracy (with an R-2 score of ~ 0.98/0.97 for the train/test set) using one of the compressed sensing techniques. This work, while unfolding the complex interplay of the descriptors in different dimensions, reveals the competence of the readily available elemental descriptors in building a robust model for predicting kappa(L).
更多查看译文
关键词
lattice thermal conductivity, machine learning, half-Heusler compounds, regression models, descriptors, SISSO
AI 理解论文
溯源树
样例
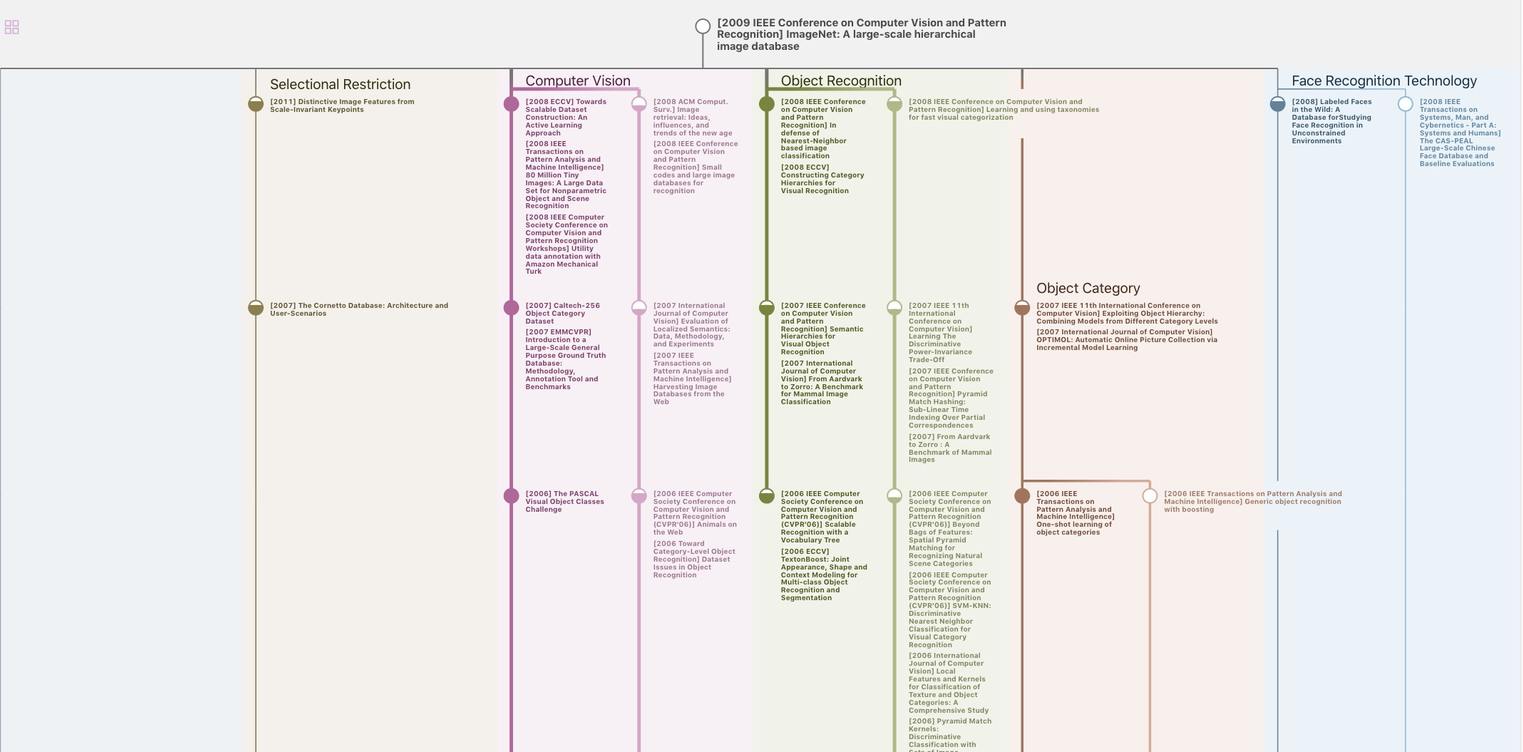
生成溯源树,研究论文发展脉络
Chat Paper
正在生成论文摘要