Learning to transfer attention in multi-level features for rotated ship detection
NEURAL COMPUTING & APPLICATIONS(2022)
摘要
Multi-scale object detection is one of the focuses of object detection, which is particularly vital for ship detection. In order to achieve the desired effects, most advanced Convolutional Neural Network-based detectors enumerate and make inferences over multi-resolution feature maps. However, the existing methods bring two critical problems: (1) Over-fitted anchor settings and supervisions for object scales will restrict the generalized performance of the algorithm. (2) Similar multi-resolution prediction branches insulate the feature space and prevent learning from branches at different levels. Drawing on the human cognitive process, this paper proposes a novel structure for multi-scale rotated ship detection called the Feature Attention Transfer module, which generates and transfers attention in multi-level feature maps to instruct each prediction branch to focus on the features that are not well extracted in other branches. Accordingly, a customized supervision method called “Inclusion–Exclusion Learning” is proposed for associative learning based on the prediction results on multi-scale branches. We employ an anchor-free rotated ship detection framework to verify the proposed module. Extensive experiments are conducted to demonstrate the effectiveness of the proposed algorithm, called SKFat, on three optical remote sensing image datasets. Experimental results show that the proposed modules improve the multi-resolution detection framework while introducing negligible inference overhead. The best result of the proposed algorithm achieves the state-of-the-art average precision while reaching a high inference speed.
更多查看译文
关键词
Multi-scale object,Ship detection,Feature attention transfer,Inclusion–exclusion learning
AI 理解论文
溯源树
样例
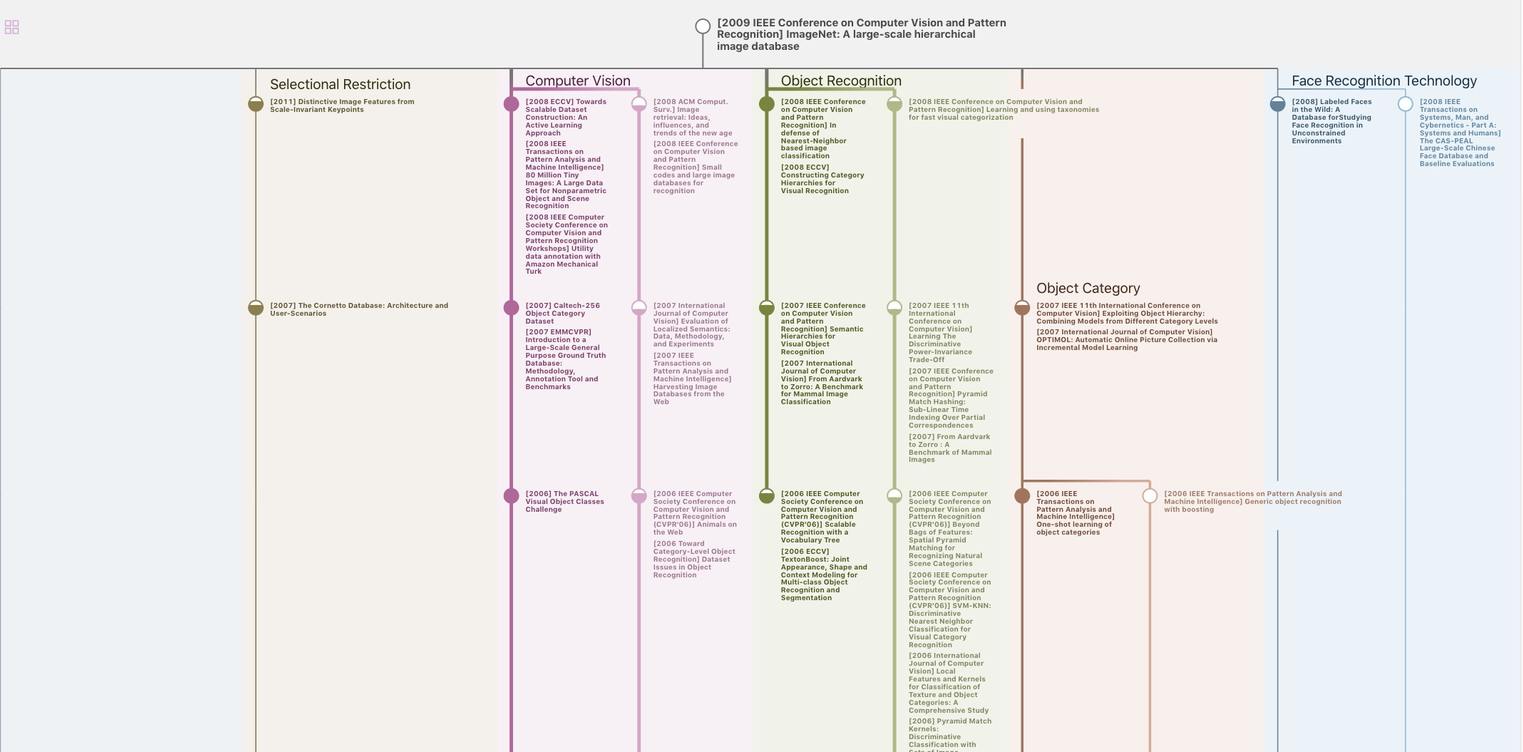
生成溯源树,研究论文发展脉络
Chat Paper
正在生成论文摘要