A physics-guided machine learning for multifunctional wave control in active metabeams
Extreme Mechanics Letters(2022)
摘要
With the growing interest in the field of artificial materials, more advanced and sophisticated wave functionalities are required from phononic crystals and acoustic metamaterials. Due to expensive iterative fitness evaluations, inverse designing acoustic metamaterials with desired physical responses using conventional optimization approaches remains challenging especially in high-dimensional design space. To address this issue, we suggest a physics-guided machine-learning-based inverse design approach for realizing multifunctional wave control in active metabeams connecting with negative capacitances (NCs). The transfer matrix method which relates the wave field and its derivative to carry the fundamental wave propagation information will be embedded in the ML network to construct the complex mapping between the input and output responses of the unit cell. After this network is well trained, global wave propagation behavior in the active metabeam can be accurately described by the concatenation of networks of each unit cells into a global stiffness matrix. After the performance of the network is validated by conducting numerical simulation, we further apply the proposed network as a surrogate model for genetic algorithm on the inverse design of the metabeam for quick realization multifunctional wave control abilities without changing microstructures. Our proposed approach can not only be easily extended to design other types of active/passive metamaterials, but also provides some insights into optimization aided engineering in high-dimensional (2D and 3D) design space of active metamaterials.
更多查看译文
关键词
Active metabeams,Machine learning,Multifunctional wave control,Surrogate optimization
AI 理解论文
溯源树
样例
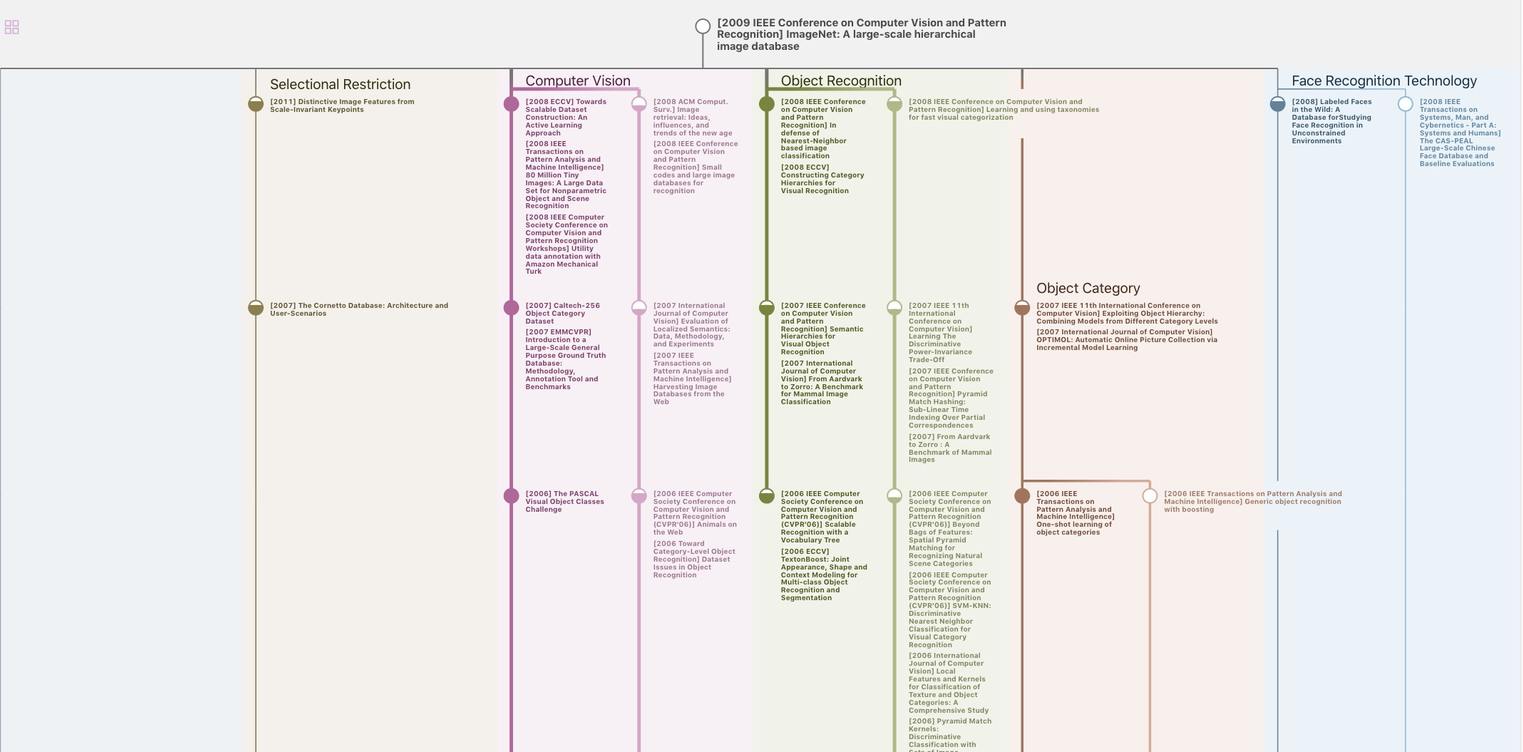
生成溯源树,研究论文发展脉络
Chat Paper
正在生成论文摘要