Numerical and experimental correlation of a catamaran’s vibration modes using supervised machine learning
Ocean Engineering(2022)
摘要
Modal parameters are crucial in investigating structural vibration problems and virtual sensing of vibration responses. This work proposes machine learning (ML) models such as Decision Tree, Random Forest, Adaboost Trees and RUSboost Trees to correlate 13 finite element (FE) modal shapes with the experimental ones. A catamaran’s FE model was assembled and used as a case study. Firstly, a full FE model with 93591 DOFs was reduced to 174 DOFs using SEREP. Secondly, as the experimental mode shapes have a low spatial resolution (only six measured DOFs), another goal of this work is to expand these 6 DOFs to the 174 DOFs of the reduced FE model, that had not been measured. The ML models were used to correlate and expand the experimental mode shapes, thus, solving their lack of spatial resolution. As a result, the ML models identified the most relevant parameters for the scaling process of each of the 13 numerical modes using a supervised database. Adaboost Trees and Random Forest were used to correlate and expand five mode shapes each and RUSboost Trees were used to correlate and expand three mode shapes. In conclusion, Adaboost Trees and Random Forest models showed better performance during the correlation process.
更多查看译文
关键词
Catamaran,Random Forest,Decision Tree,Adaboost trees,RUSboost trees,Modal Expansion
AI 理解论文
溯源树
样例
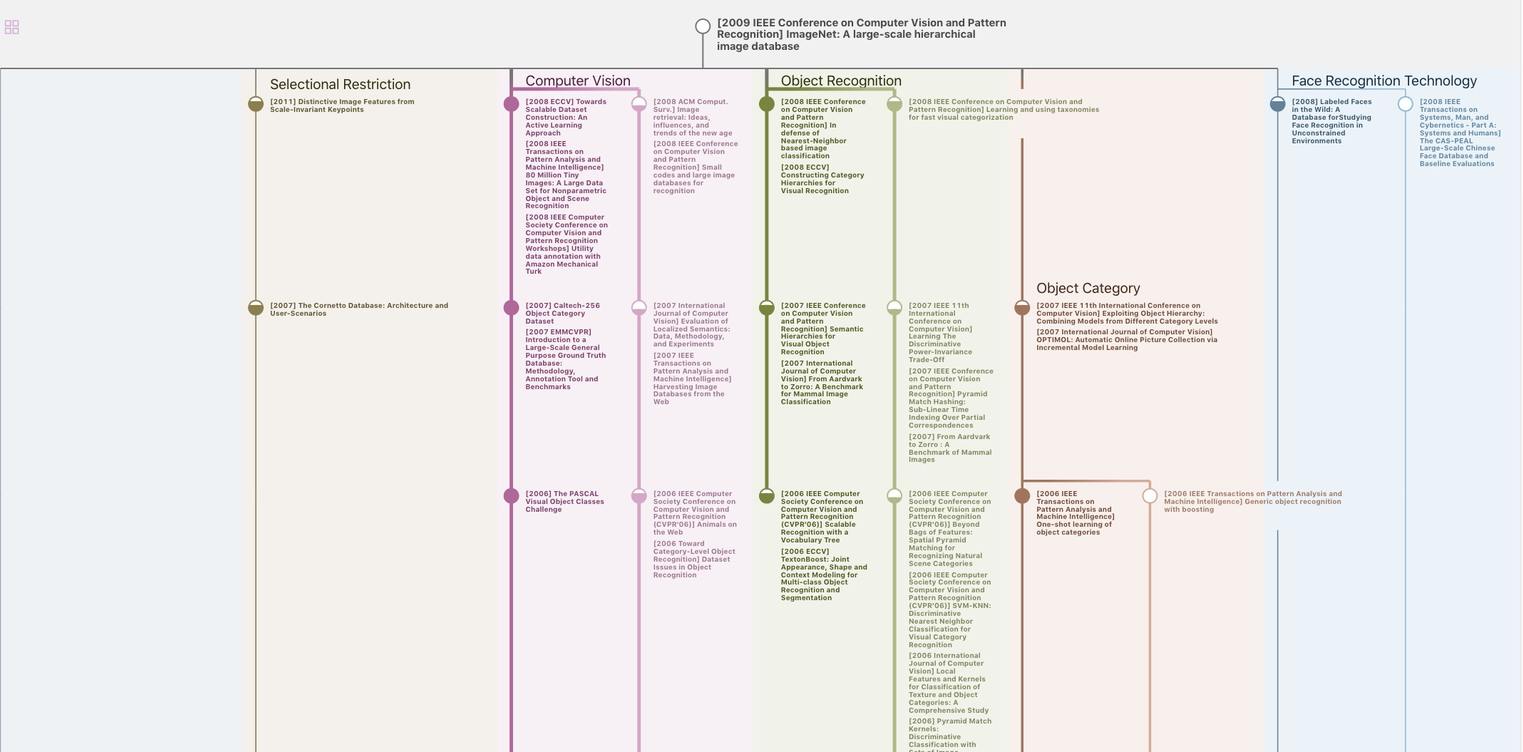
生成溯源树,研究论文发展脉络
Chat Paper
正在生成论文摘要