Driver Psychology Latent Classes as Predictors of Traffic Incident Occurrence in Naturalistic Driving Study Data
TRANSPORTATION RESEARCH RECORD(2023)
摘要
Crash risk depends on several factors, driver factors being the most significant of all. Previous studies have tended to use roadway and driver demographic information to explain crash risk, overlooking driver psychological characteristics, which are also important for crash risk estimation. Crash data from police reports are available only for reportable crashes and do not detail driver characteristics or comparable information on driving activities. Naturalistic driving studies (NDSs) offer unique opportunities to obtain information about driver attributes, behavior, or other precrash factors for predicting crash occurrence. This study estimated NDS event-oriented models to evaluate the interaction between driver attributes and roadway environmental factors for predicting safety critical events. A latent class clustering approach was used to uncover categories of drivers by psychological, perceptual, and cognitive characteristics, and by driving experience. The results revealed four driver types: risk-taker, careful-impaired, careful-unimpaired, and distractible. These types were incorporated in mixed-effects binary logistic models, with roadway, traffic, and environmental variables to estimate and predict crash risk. The models that included driver factors more successfully predicted crash risk than those without. Risk-takers showed the highest probability of being in crashes. However, careful-impaired drivers-those whose impairments made it difficult to identify the location of another vehicle, visualize missing information, who had difficulties with visual-spatial perception and executive functioning-posed a higher crash risk in roadway conditions such as snow, lack of lane markings, and certain traffic operating conditions. The results point to novel avenues for educational and behavioral interventions to improve road safety.
更多查看译文
关键词
pedestrians, bicycles, human factors, human factors of vehicles, safety, crash analysis, crash data, crash prediction models, crash severity, general, modeling and forecasting
AI 理解论文
溯源树
样例
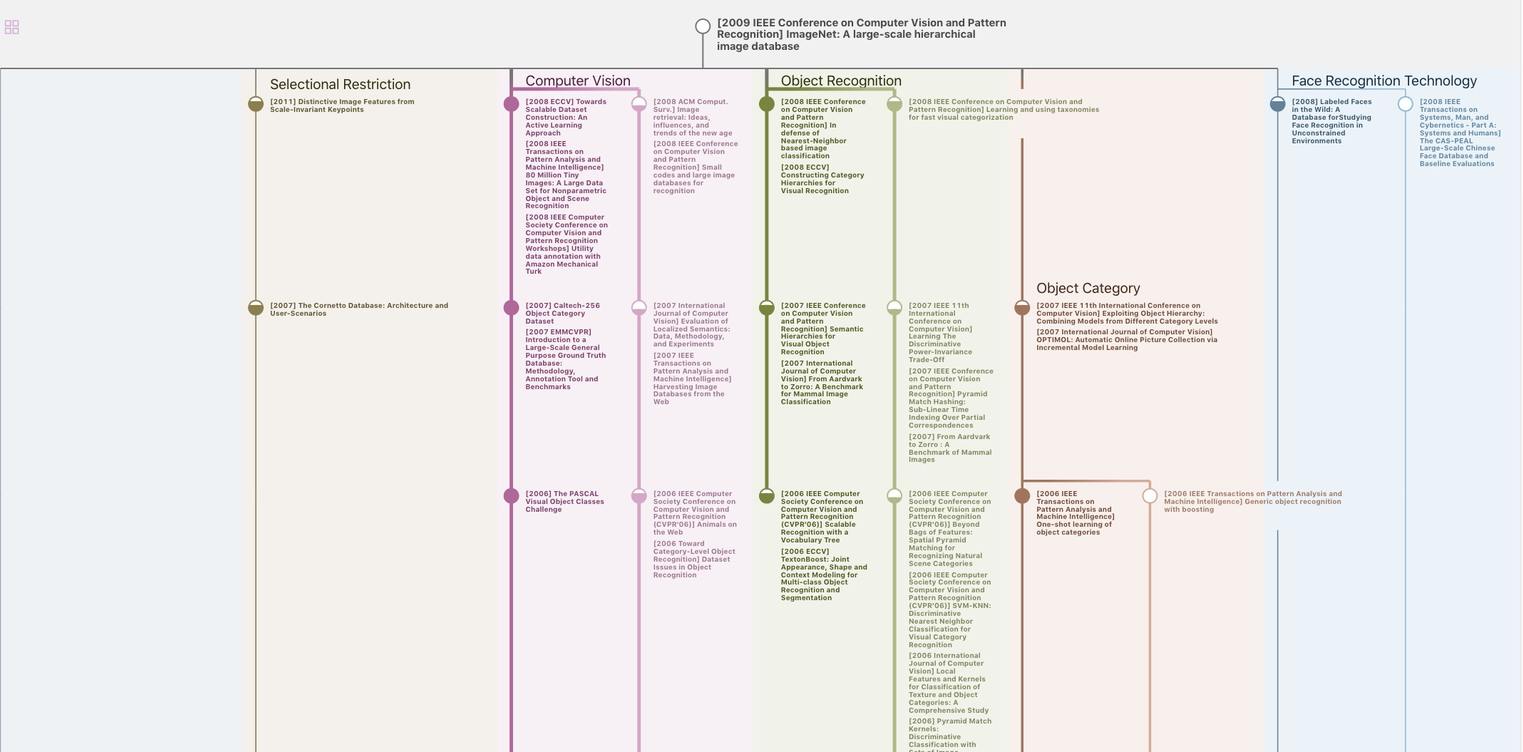
生成溯源树,研究论文发展脉络
Chat Paper
正在生成论文摘要