Dense Sampling of Time Series for Forecasting
IEEE ACCESS(2022)
摘要
A time series contain a large amount of information suitable for forecasting. Classical statistical and recent deep learning models have been widely used in a variety of forecasting applications. During the training data preparation stage, most models collect samples by sliding a fixed-sized window over the time axis of the input time series. We refer to this conventional method as "sparse sampling" because it cannot extract sufficient samples because it ignores another important axis representing the window size. In this study, a dense sampling method is proposed that extends the sampling space from one to two dimensions. The new space consists of time and window axes. Dense sampling provides several desirable effects, such as a larger training dataset, an intra-model ensemble, model-agnosticism, and an easier setting of the optimal window. The experiments were conducted using four real datasets: Bitcoin price, influenza-like illness, household electric power consumption, and wind speed. The mean absolute percentage error was measured extensively in terms of varying window sizes, horizons, and lengths of time series. The resulting data showed that dense sampling significantly and consistently outperformed sparse sampling. The source codes and datasets are available at https://github.com/isoh24/Dense-sampling-time-series.
更多查看译文
关键词
Forecasting, Time series analysis, Training data, Predictive models, Convolutional neural networks, Training, Deep learning, Deep learning, forecasting problem, LSTM, time series, training data sampling
AI 理解论文
溯源树
样例
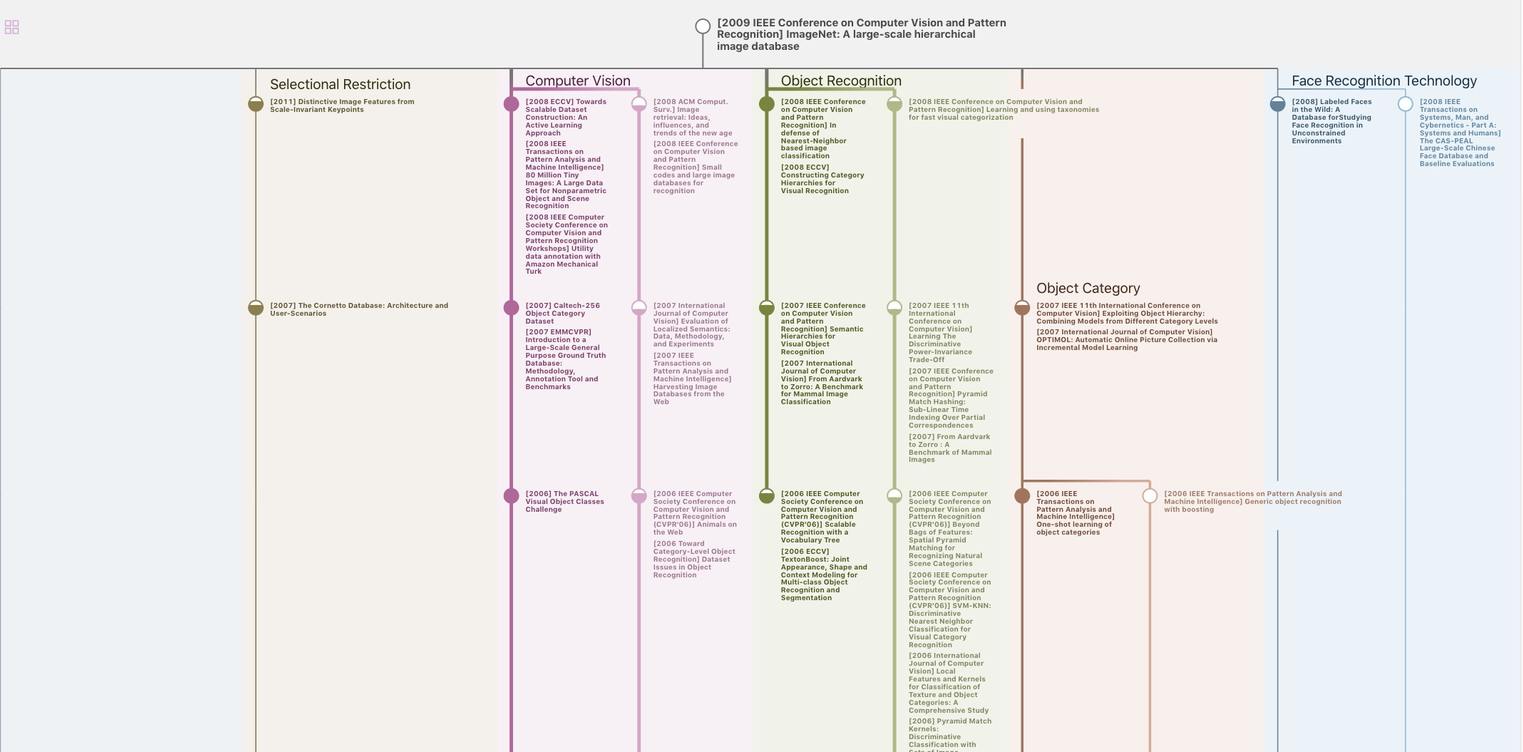
生成溯源树,研究论文发展脉络
Chat Paper
正在生成论文摘要