Deep Alternating Projection Networks for Gridless DOA Estimation With Nested Array
IEEE SIGNAL PROCESSING LETTERS(2022)
摘要
Recently, deep unfolding networks with interpretable parameters have been widely utilized in direction of arrival (DOA) estimation due to the faster convergence speed and better generalization ability. However, few consider the nested array for gridless DOA estimation. In this letter, we propose a deep alternating projection network to address the problem. We first convert the covariance matrix into a measurement vector in the form of atomic norm, which can reduce the matrix dimension during projection. We then train the proposed network to alternately obtain the positive semi-definite matrix and the corresponding irregular Hermitian Toeplitz matrix, where the loss function is derived by employing the trace of network output. Finally, we apply the irregular root Multiple Signal Classification (MUSIC) method to obtain gridless DOA via nested array. We demonstrate that the proposed networks can accelerate the convergence rate and reduce computational cost. Simulations verify the performance of proposed networks in comparison with the existing methods.
更多查看译文
关键词
Direction-of-arrival estimation, Estimation, Covariance matrices, Atomic measurements, Eigenvalues and eigenfunctions, Convergence, Computational efficiency, Gridless, direction of arrival (DOA), deep unfolding network, alternating projection, nested array
AI 理解论文
溯源树
样例
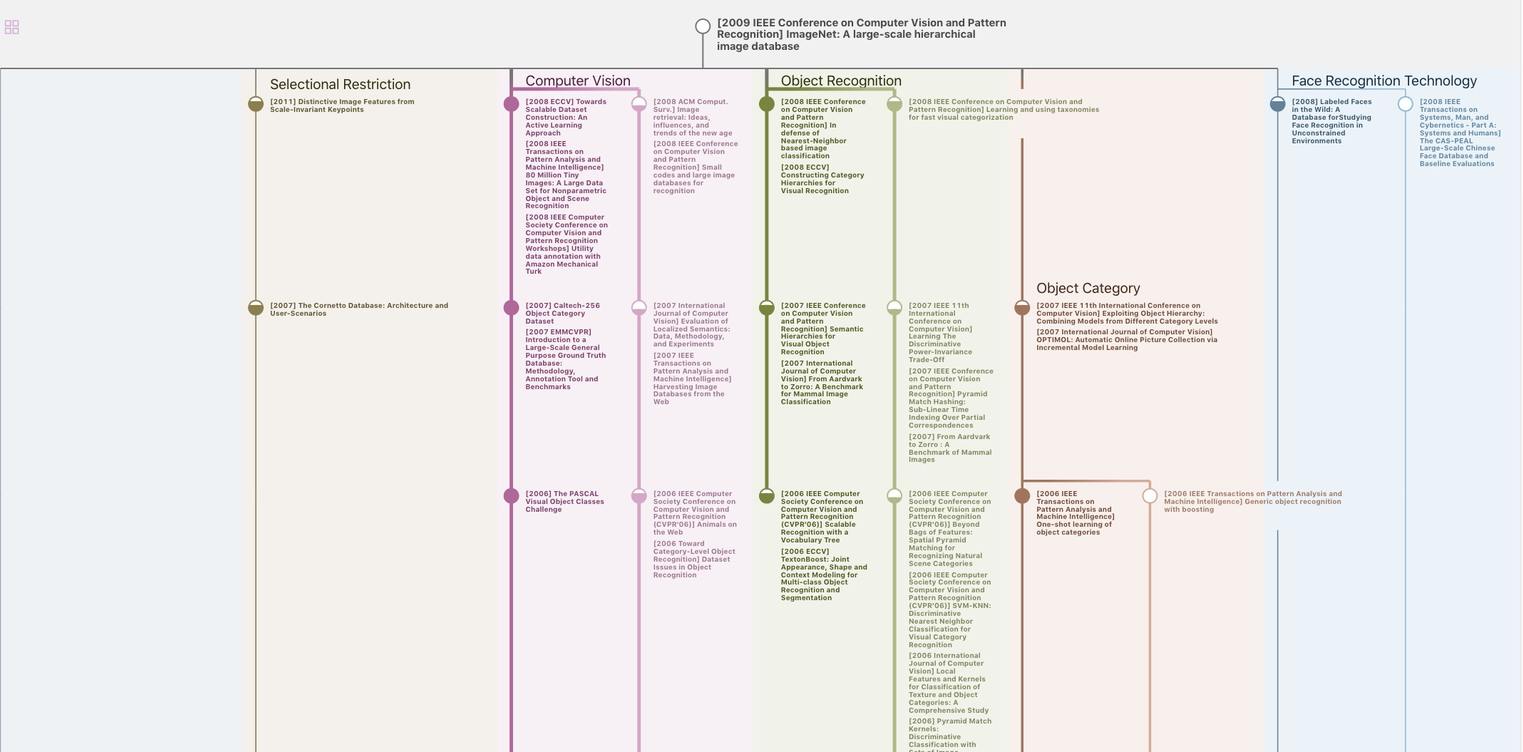
生成溯源树,研究论文发展脉络
Chat Paper
正在生成论文摘要