Loss-based differentiation strategy for privacy preserving of social robots
JOURNAL OF SUPERCOMPUTING(2022)
摘要
The training and application of machine learning models encounter the leakage of a significant amount of information about their training dataset that can be investigated by inference attack or model inversion in the fields, such as computer vision, social robots. The conventional methods for the privacy preserving exploit to apply differential privacy into the training process, which might bring a negative influence on the convergence or robustness. We first conjecture the necessary steps to carry out a successful membership inference attack in a machine learning setting and then explicitly formulate the defense based on the conjecture. This paper investigates the construction of new training parameters with a Loss-based Differentiation Strategy (LDS) for a new learning model. The main idea of LDS is to partition the training dataset into some folds and sort their training parameters by similarity to enable privacy-accuracy inequality. The LDS-based model leakages less information on MIA than the primitive learning model and makes it impossible for the adversary to generate the representative samples. Finally, extensive simulations are conducted to validate the proposed scheme and the results demonstrate that the LDS can lower the MIA accuracy in terms of most CNNs models.
更多查看译文
关键词
Machine learning,Training loss differentiation,Information leakage,Privacy preserving
AI 理解论文
溯源树
样例
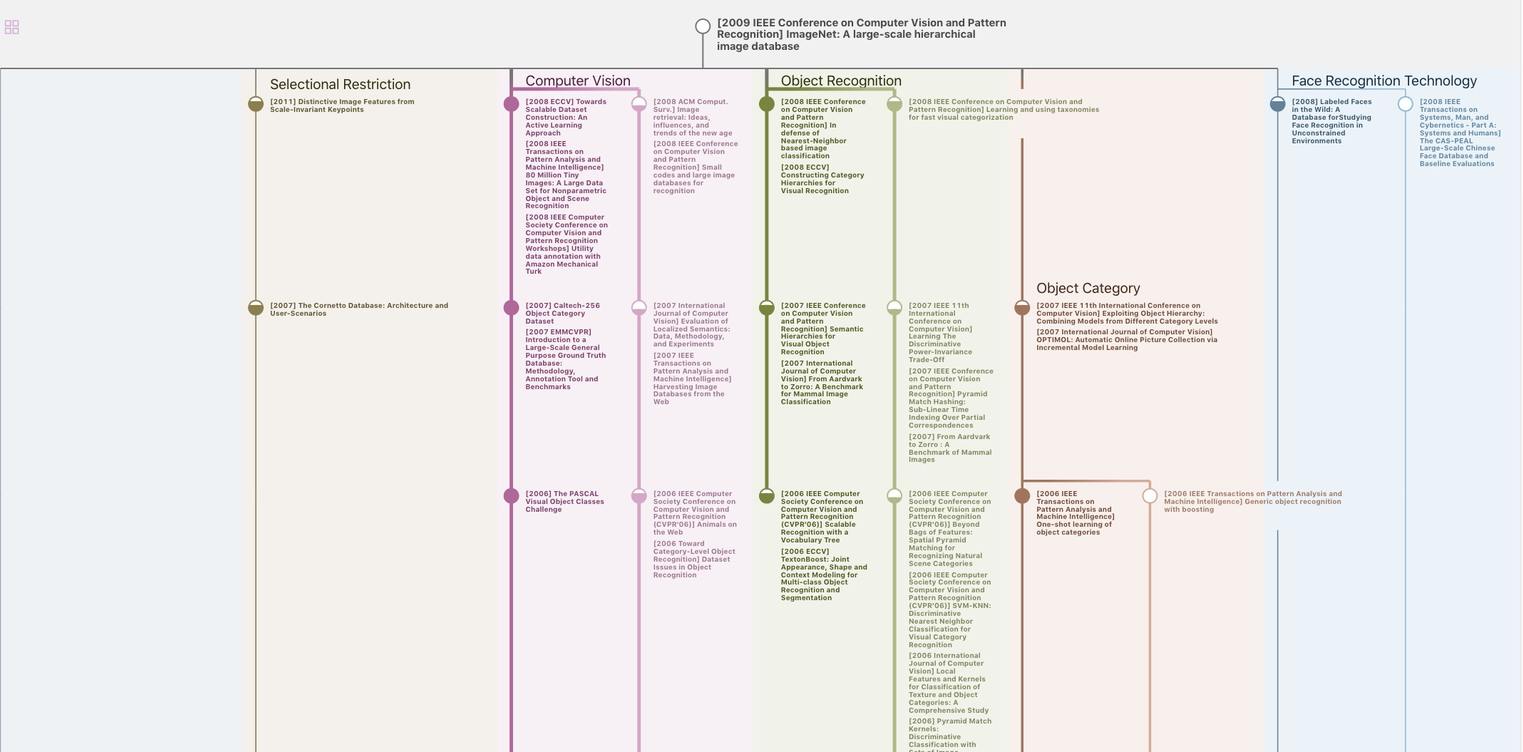
生成溯源树,研究论文发展脉络
Chat Paper
正在生成论文摘要