Low-overhead inverted LUT design for bounded DNN activation functions on floating-point vector ALUs
Microprocessors and Microsystems(2022)
摘要
An inference engine uses floating-point numbers to provide high accuracy in deep neural network computing despite its computing resource limitations. However, the computation for non-linear activation functions occurs the performance bottleneck, and we may alleviate it by adopting a lookup table (LUT) method. However, the floating-point number system’s characteristic, where intervals between mantissa numbers differ depending on their exponent values, makes it challenging to calculate LUT index values and produce the error-tolerant outputs.
更多查看译文
关键词
Lookup table,Bfloat16,Activation functions,Deep neural networks
AI 理解论文
溯源树
样例
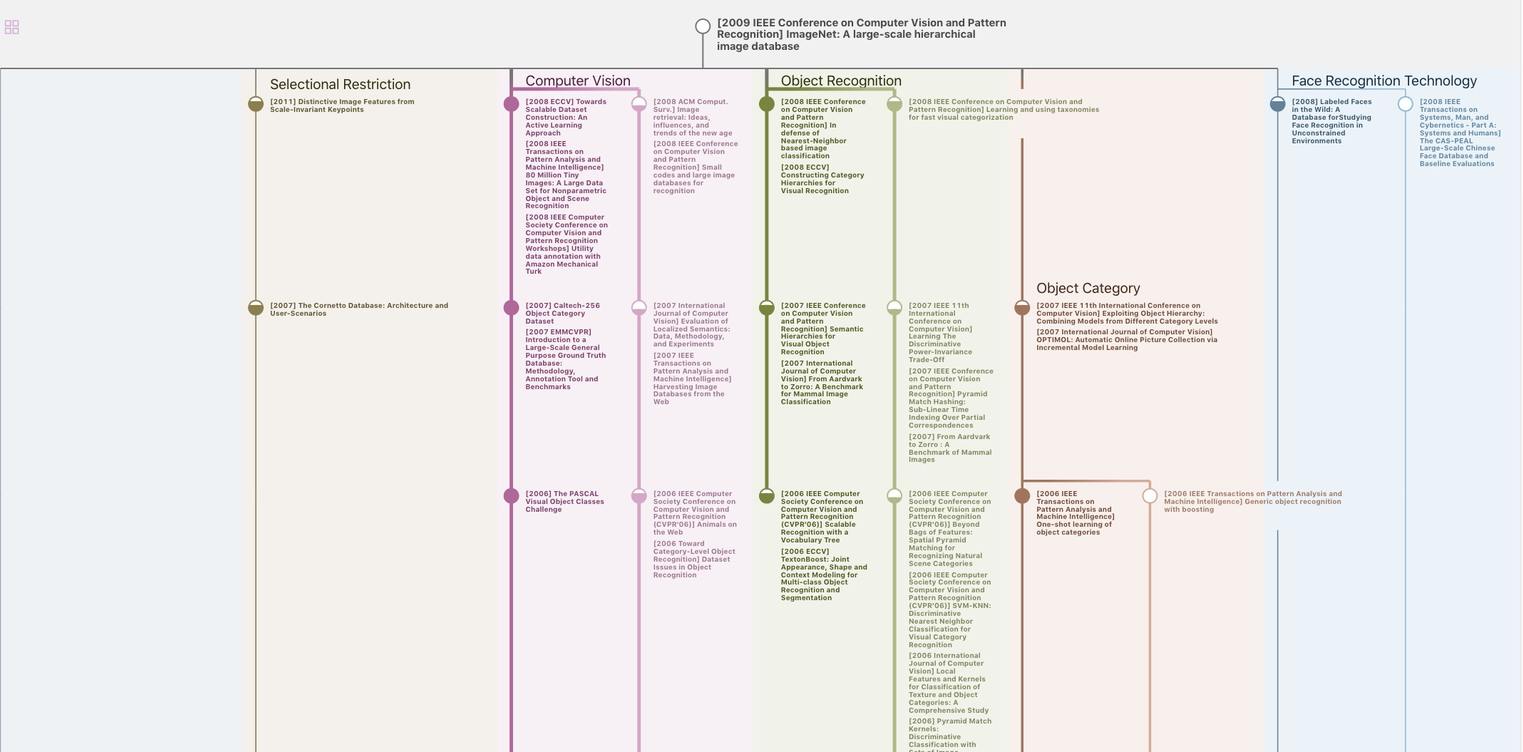
生成溯源树,研究论文发展脉络
Chat Paper
正在生成论文摘要