InfoAT: Improving Adversarial Training Using the Information Bottleneck Principle
IEEE TRANSACTIONS ON NEURAL NETWORKS AND LEARNING SYSTEMS(2024)
摘要
Adversarial training (AT) has shown excellent high performance in defending against adversarial examples. Recent studies demonstrate that examples are not equally important to the final robustness of models during AT, that is, the so-called hard examples that can be attacked easily exhibit more influence than robust examples on the final robustness. Therefore, guaranteeing the robustness of hard examples is crucial for improving the final robustness of the model. However, defining effective heuristics to search for hard examples is still difficult. In this article, inspired by the information bottleneck (IB) principle, we uncover that an example with high mutual information of the input and its associated latent representation is more likely to be attacked. Based on this observation, we propose a novel and effective adversarial training method (InfoAT). InfoAT is encouraged to find examples with high mutual information and exploit them efficiently to improve the final robustness of models. Experimental results show that InfoAT achieves the best robustness among different datasets and models in comparison with several state-of-the-art methods.
更多查看译文
关键词
Robustness,Mutual information,Training,Neural networks,Perturbation methods,Standards,Linear programming,Adversarial training (AT),information bottleneck (IB),mutual information,robustness
AI 理解论文
溯源树
样例
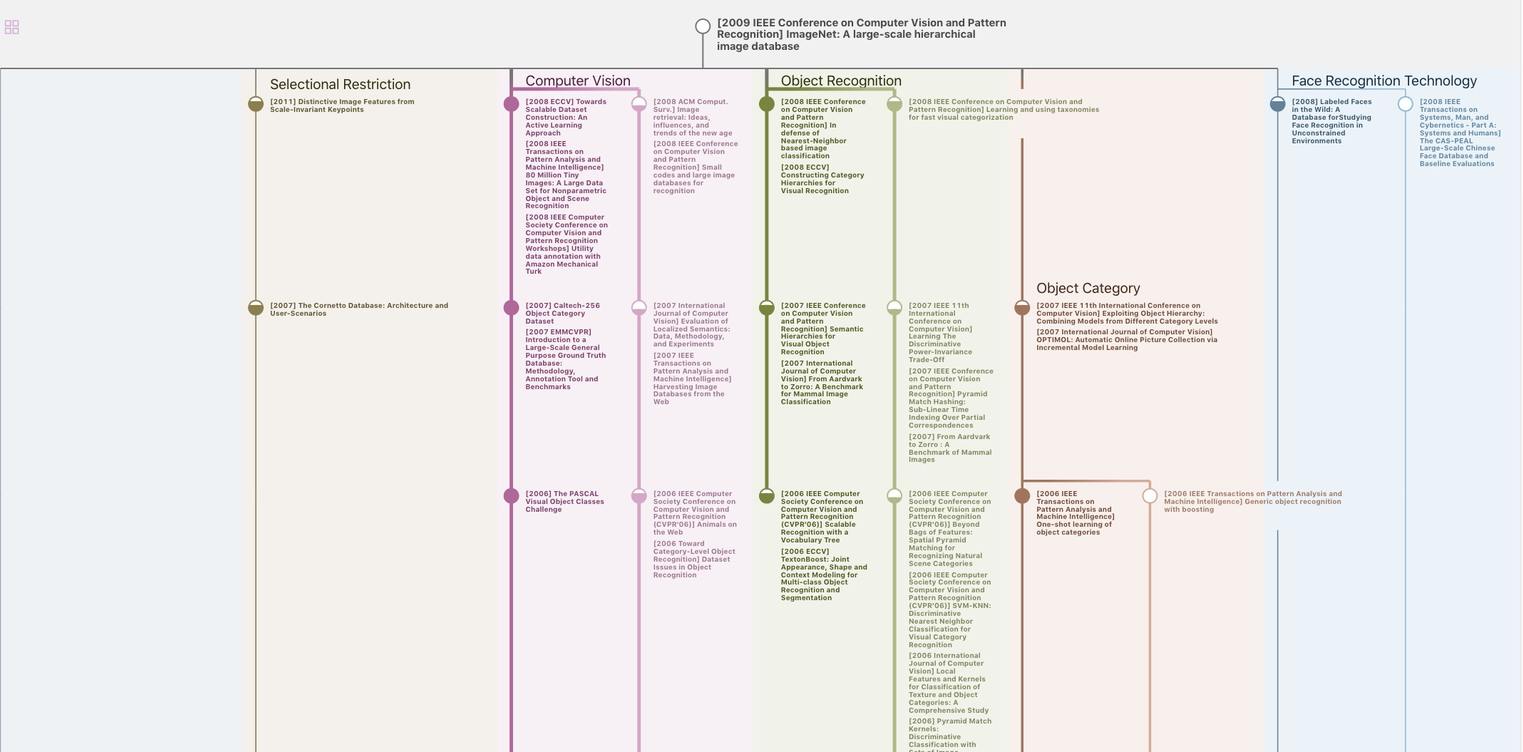
生成溯源树,研究论文发展脉络
Chat Paper
正在生成论文摘要