An Augmented Neural Network for Sentiment Analysis Using Grammar
FRONTIERS IN NEUROROBOTICS(2022)
摘要
Understanding human sentiment from their expressions is very important in human-robot interaction. But deep learning models are hard to represent grammatical changes for natural language processing (NLP), especially for sentimental analysis, which influence the robot's judgment of sentiment. This paper proposed a novel sentimental analysis model named MoLeSy, which is an augmentation of neural networks incorporating morphological, lexical, and syntactic knowledge. This model is constructed from three concurrently processed classical neural networks, in which output vectors are concatenated and reduced with a single dense neural network layer. The models used in the three grammatical channels are convolutional neural networks (CNNs), long short-term memory (LSTM) networks, and fully connected dense neural networks. The corresponding output in the three channels is morphological, lexical, and syntactic results, respectively. Experiments are conducted on four different sentimental analysis corpuses, namely, hotel, NLPCC2014, Douban movie reviews dataset, and Weibo. MoLeSy can achieve the best performance over previous state-of-art models. It indicated that morphological, lexical, and syntactic grammar can augment the neural networks for sentimental analysis.
更多查看译文
关键词
sentiment analysis, multi-channel CNN, grammar, augmentation, morphological
AI 理解论文
溯源树
样例
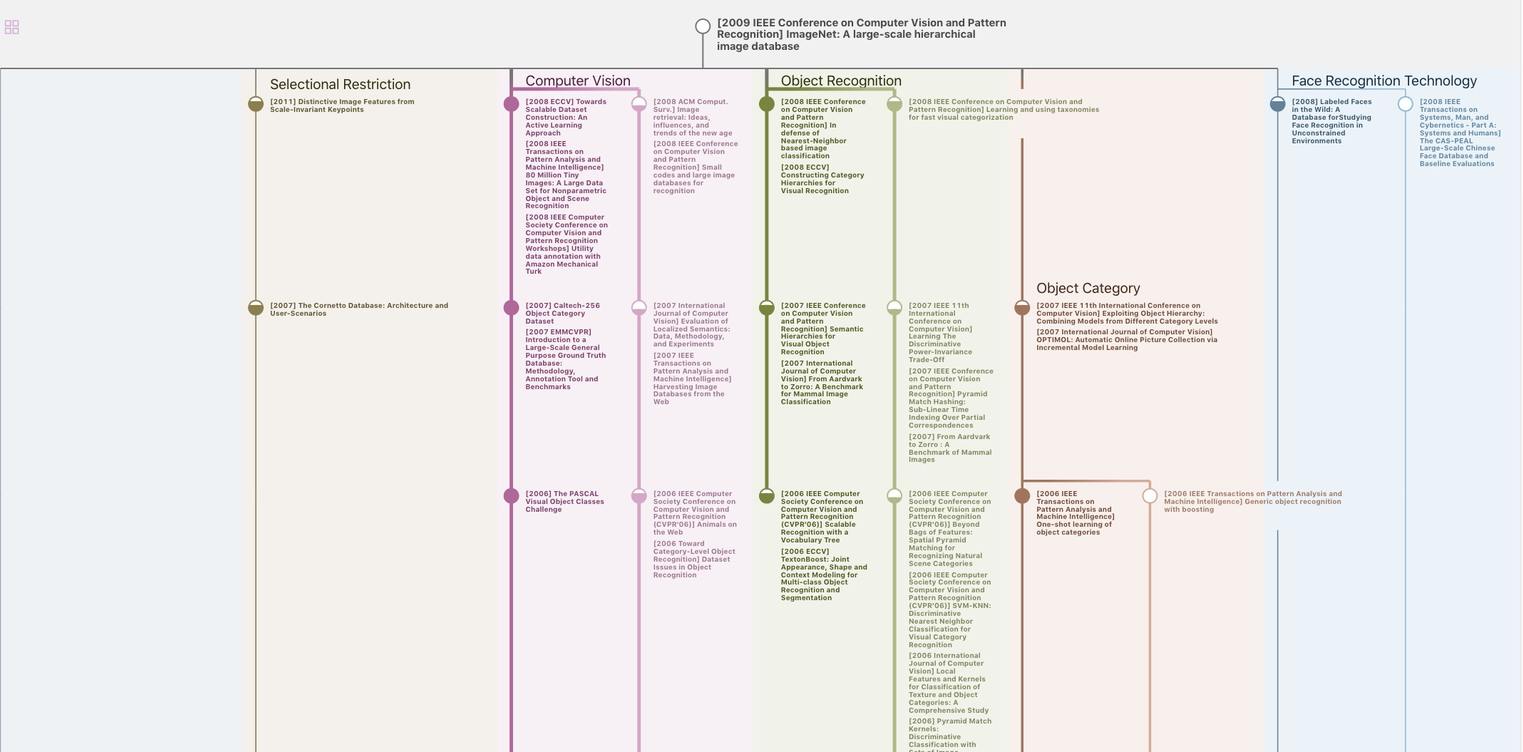
生成溯源树,研究论文发展脉络
Chat Paper
正在生成论文摘要