Active space control of underactuated robots: From adaptation to robustness to optimality
INTERNATIONAL JOURNAL OF ADAPTIVE CONTROL AND SIGNAL PROCESSING(2022)
摘要
This article develops a controller for the active space control of underactuated robotic systems with the ability to adapt to unknown parameters, be robust to unmodeled dynamics and disturbances, and generate optimal control policies in a pointwise fashion. To achieve the set forth objectives, the model dynamics are first partitioned into active and passive spaces. As a remedy for nonlinear coupling between these spaces, the system's acceleration is estimated for use in the control algorithm. The modeling uncertainty associated with both unknown system parameters and unknown control map is then estimated by proposing a concurrent learning adaptive approach. An online quadratic program (QP) is synthesized utilizing an intelligent robust control Lyapunov function (IRCLF) constraint to ensure the system stability with minimal control effort while using the estimates of the unknown dynamics. The proposed IRCLF scheme automatically compensate for acceleration estimation errors, unmodeled dynamics, and disturbances without the knowledge of their bounds a priori. The uniform ultimate boundedness of all system signals is proven using the Lyapunov stability argument and Barbalat's lemma. The performance of the proposed QP-AIR-CLF control framework is validated on two different underactuated systems: a foot-leg model on a deformable ground, and an overhead crane system. Simulation results show the benefits of the proposed controller over the baseline QP-RCLF and an adaptive QP-RCLF in terms of the control optimality, tracking accuracy, dynamic estimation performance, and robustness to disturbances.
更多查看译文
关键词
adaptive concurrent learning, quadratic program, robust control Lyapunov function, underactuated system
AI 理解论文
溯源树
样例
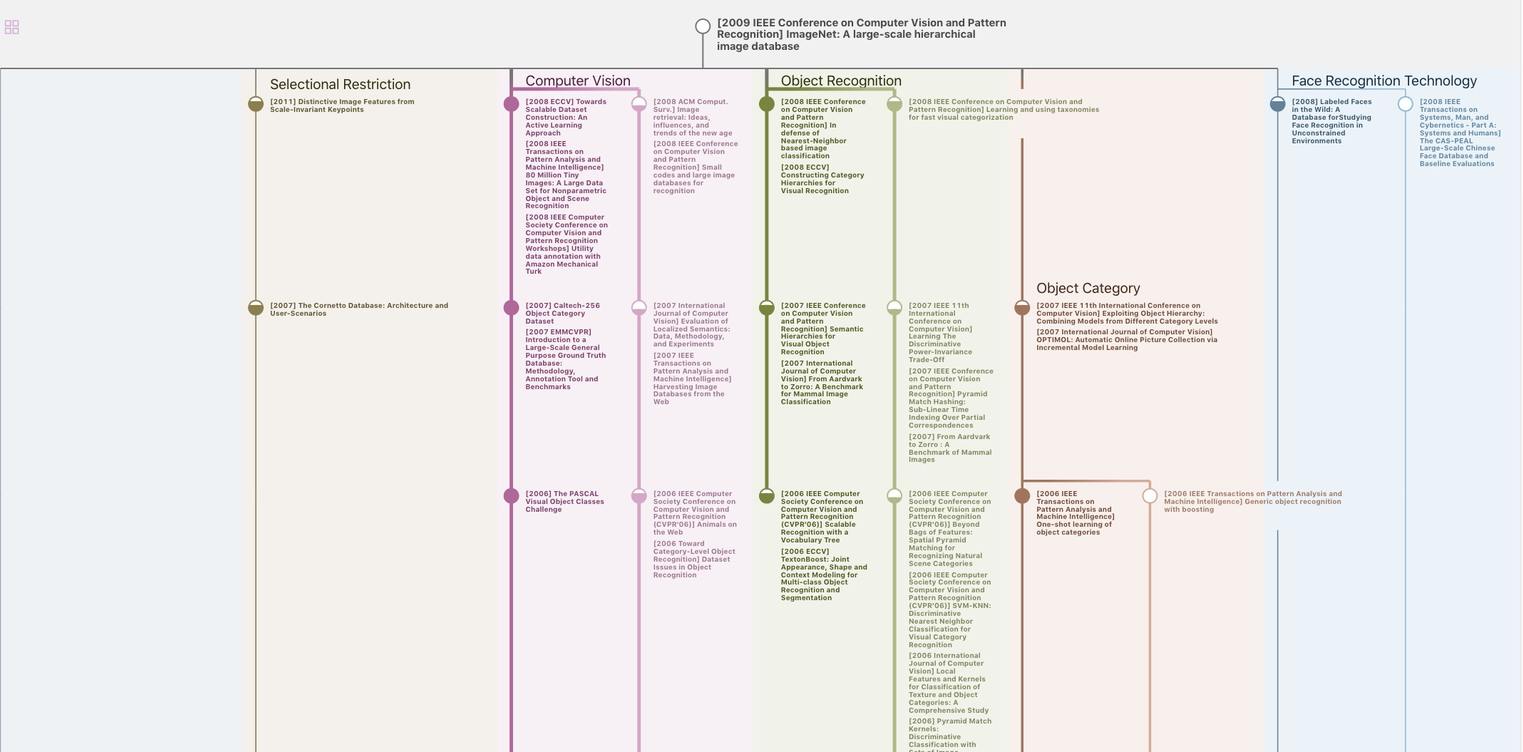
生成溯源树,研究论文发展脉络
Chat Paper
正在生成论文摘要