Accurate Raman indicators of protein synthesis through sparse classification
BIOMEDICAL VIBRATIONAL SPECTROSCOPY 2022: ADVANCES IN RESEARCH AND INDUSTRY(2022)
摘要
Raman spectroscopy has the ability to retrieve in a non-invasive way molecular information from cells and tissues, allowing the detection of various biological responses such as cancer, inflammation or drug response, for example. Due to the relatively low signals that are retrieved with this technique, multivariate analysis is often employed to detect the changes of interest. We study different variable reduction approaches to improve stability and accuracy of detection, namely classical PCA/LDA, regularized regression (Lasso) and PLS-DA, and apply them to high-throughput single-cell measurements for the detection of inflammation or distinction of close phenotypes. We show how both Lasso and PLS-DA yield better performance than PCA/LDA with models reaching stability of over a year even in the case of small cellular changes. We then used protein synthesis, a well understood biological process, as a case study to assess which Raman bands are the most significant for classification. As Lasso yields sparse spectra with a limited set of bands that are selected by regularized regression, it is possible to ease the interpretation of the relevant biomarkers detected through spectroscopy. Also in the case of protein synthesis measurements, both Lasso and PLS-DA to reach high accuracy (over 95%), but Lasso provides signals that are easier to interpret as only a small subset of bands are present in the vector.
更多查看译文
关键词
Raman, Single-cell, Machine learning, Macrophages, Protein synthesis
AI 理解论文
溯源树
样例
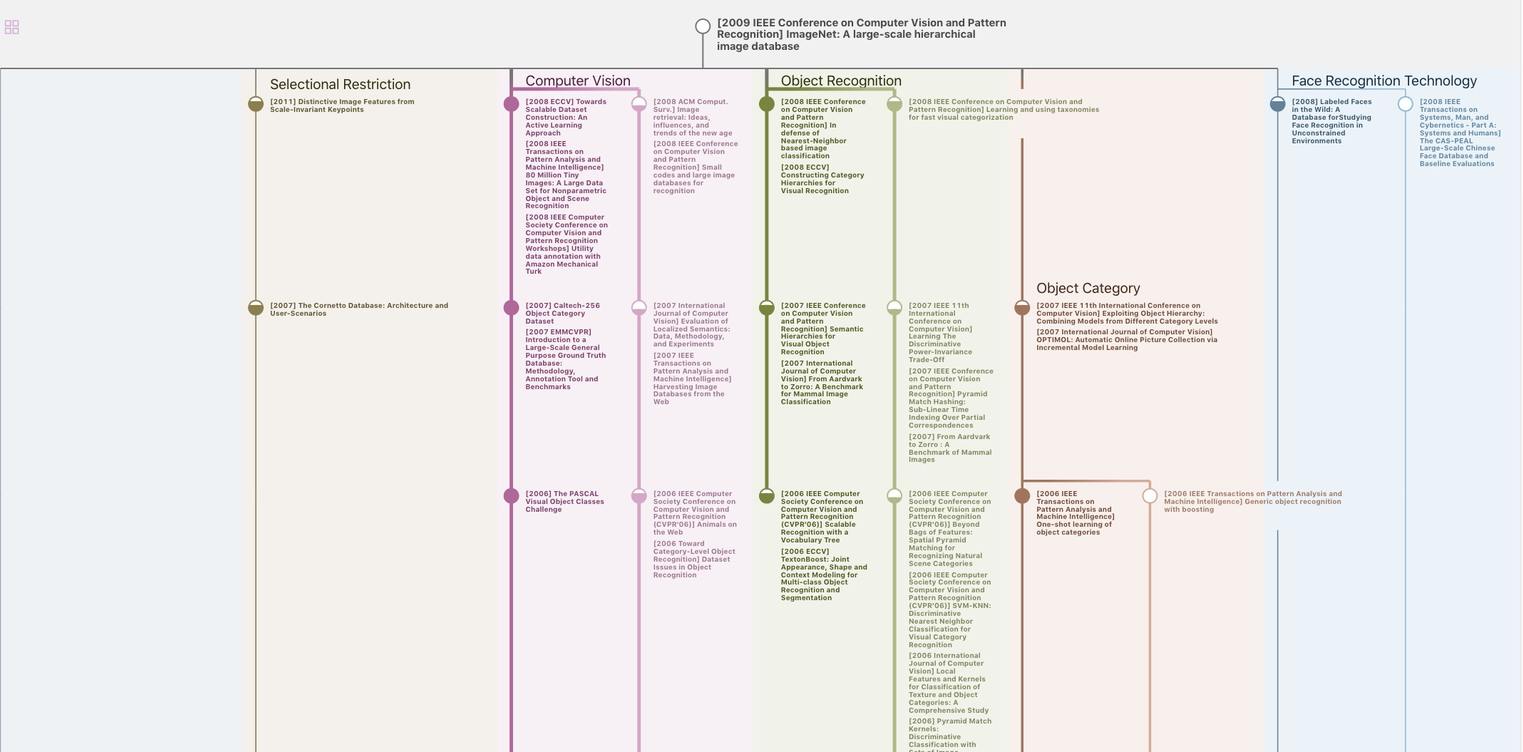
生成溯源树,研究论文发展脉络
Chat Paper
正在生成论文摘要