Surrogate-Assisted Multi-Objective Evolutionary Optimization With Pareto Front Model-Based Local Search Method
IEEE TRANSACTIONS ON CYBERNETICS(2024)
摘要
Some local search methods have been incorporated into surrogate-assisted multi-objective evolutionary algorithms to accelerate the search toward the real Pareto front (PF). In this article, a PF model-based local search method is proposed to accelerate the exploration and exploitation of the PF. It first builds a predicted PF model with current nondominated solutions. Then, some sparse points in the predicted PF are selected to guide the search directions of the local search in order to promote the search of promising sparse areas. The approximation degree of the predicted and real PFs will influence the speed of the local search, while extreme points can significantly influence the shape of the PF. To accelerate the search progress, the optima of surrogate models are utilized to promote the progress of finding extreme points. The proposed local search method is incorporated into a surrogate-assisted multi-objective evolutionary algorithm. The proposed surrogate-assisted multi-objective evolutionary algorithm with the proposed local search method is tested with Zitzler-Deb-Thiele (ZDT), Deb-Thiele-Laummans-Zitzler (DTLZ), and MAF instances. The experimental results demonstrated the efficiency of the proposed local search method and the superiority of the proposed algorithm.
更多查看译文
关键词
Search problems,Optimization,Evolutionary computation,Statistics,Sociology,Predictive models,Computational modeling,Local search method,multi-objective expensive problems,Pareto front (PF) model,surrogate model
AI 理解论文
溯源树
样例
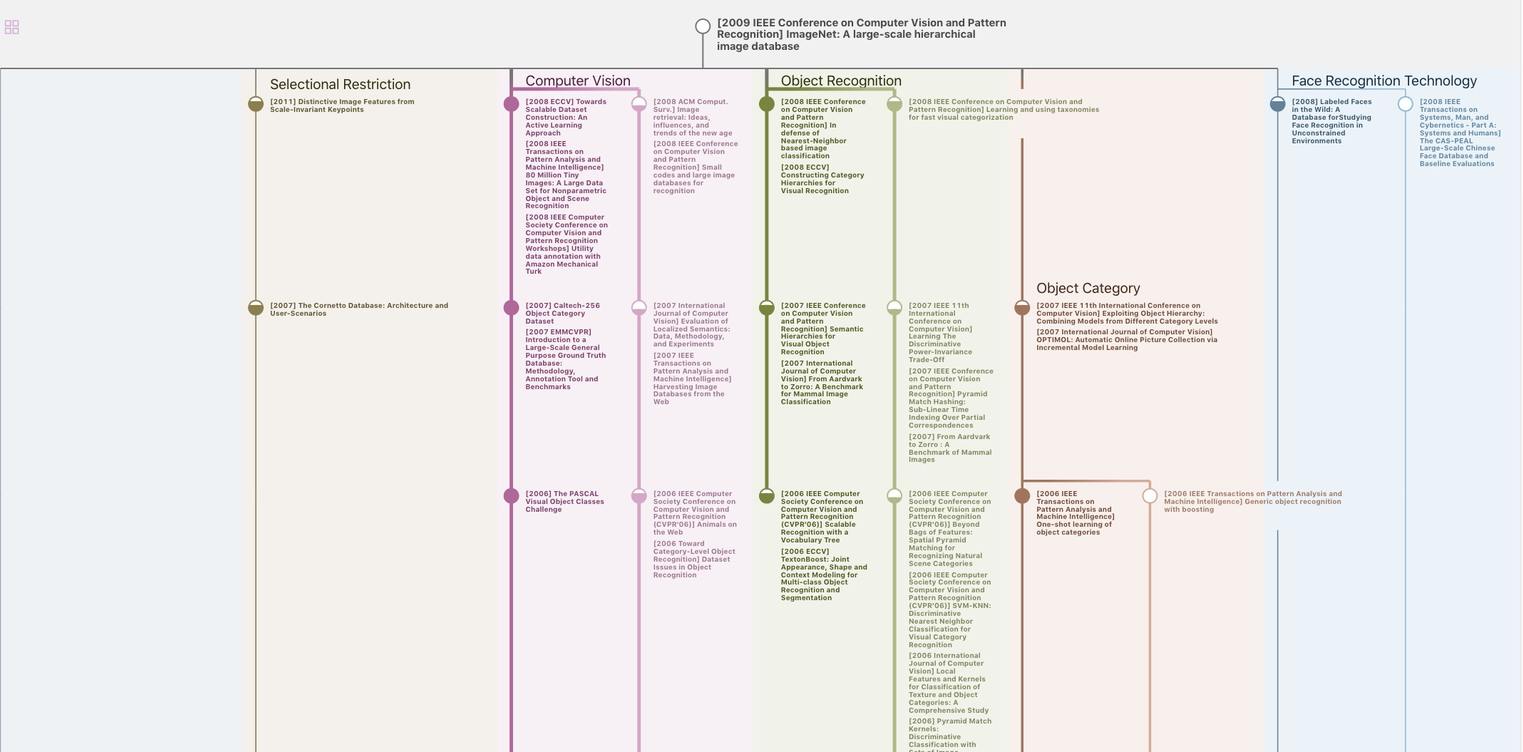
生成溯源树,研究论文发展脉络
Chat Paper
正在生成论文摘要