PARS-Push: Personalized, Asynchronous and Robust Decentralized Optimization
IEEE Control Systems Letters(2023)
摘要
We study the multi-step Model-Agnostic Meta-Learning (MAML) framework where a group of
$n$
agents seeks to find a common point that enables “few-shot” learning (personalization) via local stochastic gradient steps on their local functions. We formulate the personalized optimization problem under the MAML framework and propose PARS-Push, a decentralized asynchronous algorithm robust to message failures, communication delays, and directed message sharing. We characterize the convergence rate of PARS-Push under arbitrary multi-step personalization for smooth strongly convex, and smooth non-convex functions. Moreover, we provide numerical experiments showing its performance under heterogeneous data setups.
更多查看译文
关键词
Decentralized optimization,personalization,few-shot learning,model-agnostic meta-learning,asynchronous communications
AI 理解论文
溯源树
样例
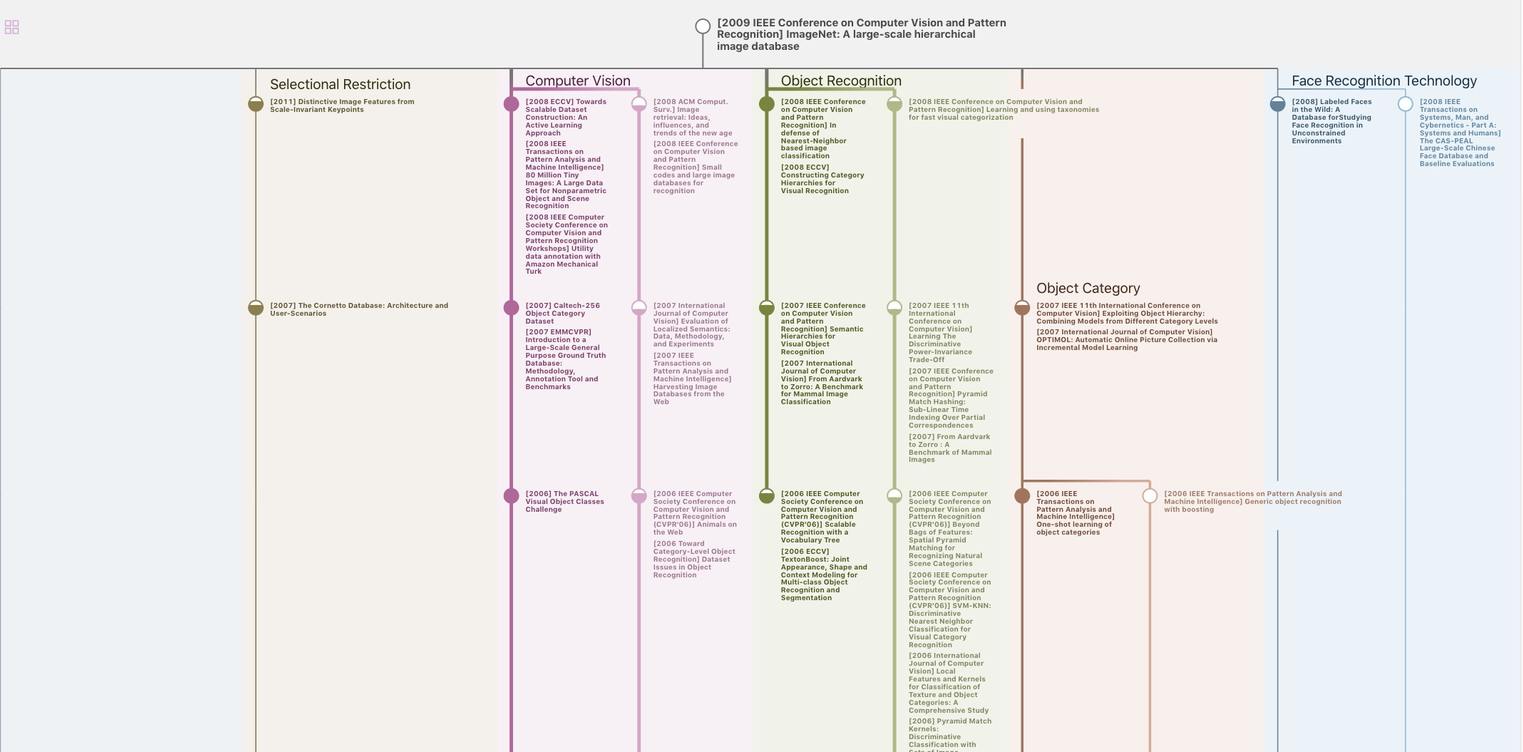
生成溯源树,研究论文发展脉络
Chat Paper
正在生成论文摘要