Machine learning and transfer learning for correction of the chemical and physical matrix effects in the determination of alkali and alkaline earth metals with LIBS in rocks
Spectrochimica Acta Part B: Atomic Spectroscopy(2022)
摘要
Alkali and alkaline earth metals incorporated in rocks can indicate past water activities. Their precise determination with laser-induced breakdown spectroscopy (LIBS) is required for geological investigations on Earth as well as on Mars. In previous studies, laboratory standard samples in the form of pressed powder pellets were used to train univariate or multivariate regression models. Although various chemical compositions of the used samples cover the chemical and mineralogical diversities of the rocks encountered in field measurements, the difference in physical forms between the laboratory standards and the real samples analyzed in situ leads to the physical matrix, which dramatically reduces the prediction performance of the models for real samples. The present work performs a systematic study of the influences of the chemical and physical matrix effects, and develops multivariate regression models based on machine learning and transfer learning to effectively correct these effects. The experiment involved a set of 27 fully characterized natural rocks. A part of each rock was prepared in a pressed powder pellet, while another part was left in its raw state. LIBS spectra recorded from pellets were first used to train multivariate regression models based on a machine learning approach combining feature selection and back-propagation neural network (BPNN). The trained models were tested respectively by independent pellets and raw rocks with and without counterpart pellets in the training sample set. An effective correction of the chemical matrix effect was observed for the models when tested with pellets. While the tests with raw rocks revealed the influence of the physical matrix effect on the prediction performance of the models. Transfer learning was then introduced by including a part of the raw rocks in the training sample set, leading to a significant improvement of the prediction performances for raw rocks, with root mean square errors of prediction (RMSEPs) of 1.6, 18, 101, and 162 wt. ppm respectively for Li, Rb, Sr, and Ba.
更多查看译文
关键词
LIBS,Rocks,Alkali and alkaline earth metals,Chemical and physical matrix effects,Machine learning,Transfer learning
AI 理解论文
溯源树
样例
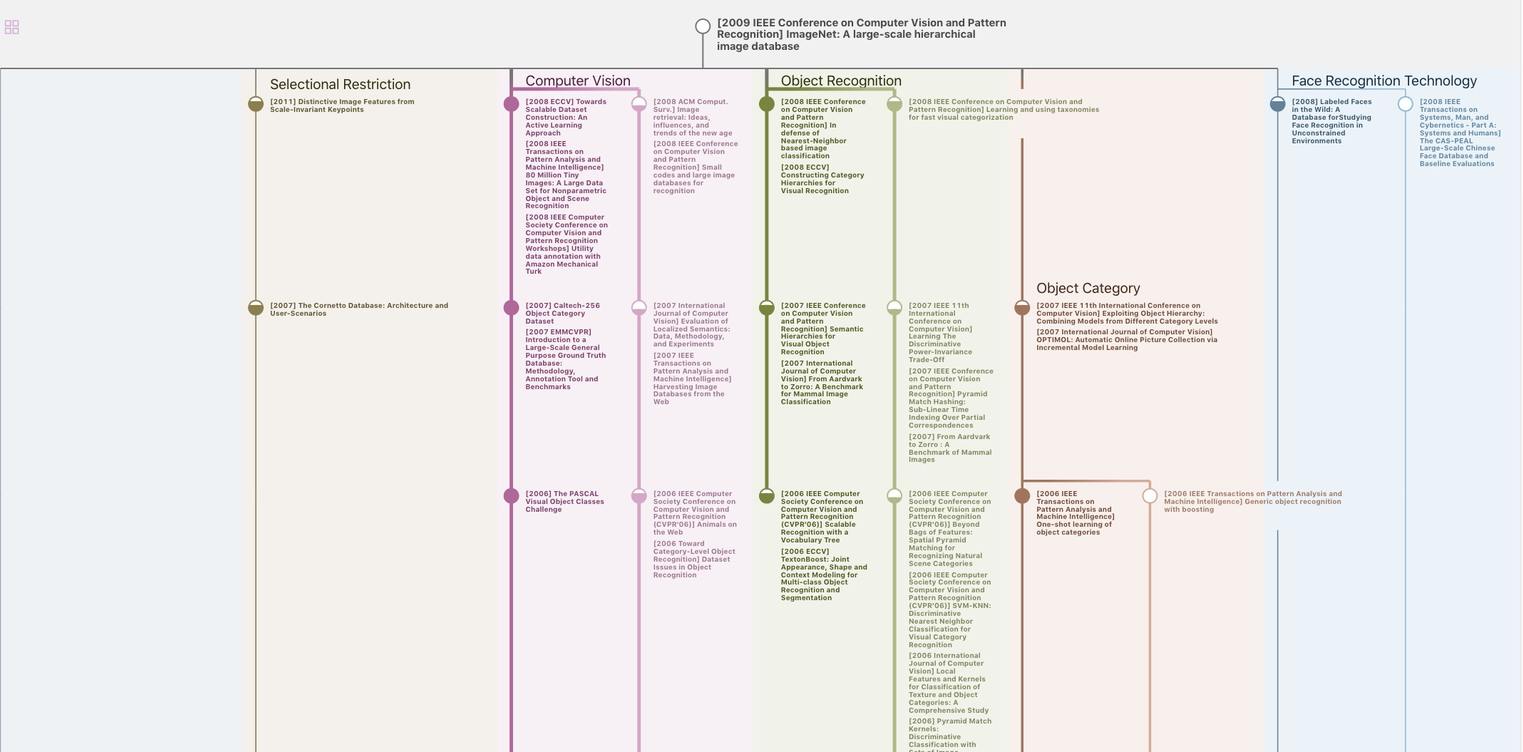
生成溯源树,研究论文发展脉络
Chat Paper
正在生成论文摘要