Two-stage 3D object detection guided by position encoding
Neurocomputing(2022)
摘要
Voxel-based structures in 3D detection have achieved rapid advancement due to their superior capability for feature extraction. However, the accuracy is usually low because the point cloud is divided into a grid. In order to overcome the above problems and improve detection accuracy, we propose a flexible two-stage 3D object detection architecture, which adopts two branches to refine generated proposals, aggregating voxel features and raw point features simultaneously. We also design a new gating mechanism to achieve fusion features from different levels. In addition, we propose a novel feature aggregation module to reduce the semantic gap between the features of the two types. First, a transformer based on raw points is employed as an encoder to aggregate the contextual information. Then, the point-based channel-wise self-attention mechanism serves as a decoder to aggregate the global features. Experiment results on the KITTI 3D dataset and Waymo Open datest demonstrate that our approach outperforms the state-of-the-art methods and exhibits excellent scalability.
更多查看译文
关键词
3D detection,Position encoding,Transformer,Self-attention
AI 理解论文
溯源树
样例
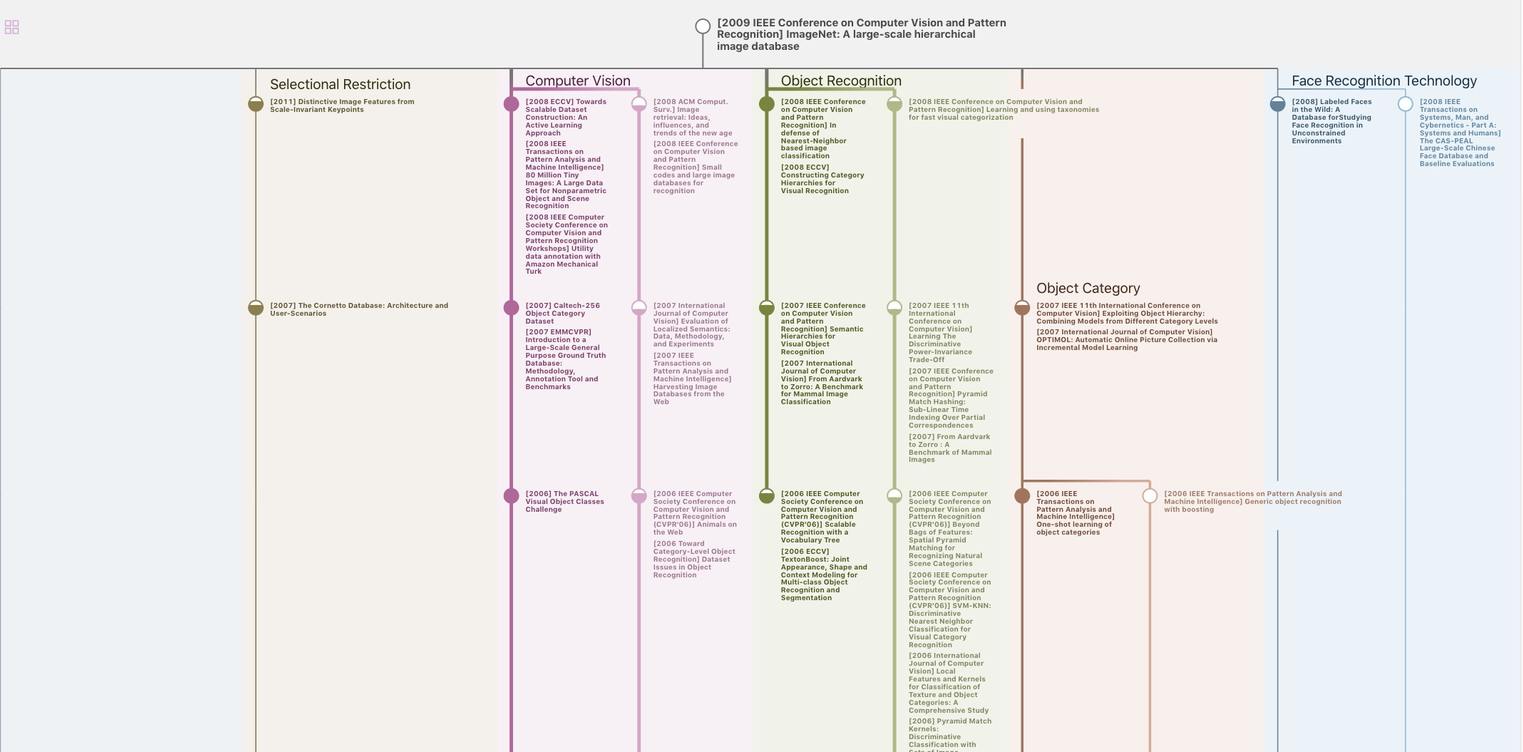
生成溯源树,研究论文发展脉络
Chat Paper
正在生成论文摘要