Actuarial intelligence in auto insurance: Claim frequency modeling with driving behavior features and improved boosted trees
Insurance: Mathematics and Economics(2022)
摘要
Usage-based insurance (UBI) is now a sought-after auto insurance product in the market. By using a wide range of telematics data, insurance companies can better understand the insured's driving behavior and capture the relationship between insurance loss and the relevant risk factors. This study examines the frequency of UBI claims and combines machine learning algorithms with classic actuarial distributions to establish the predictive model. More specifically, considering the large number of driving behavior features and their complex interactions, we replace generalized linear models with boosted trees, and synchronously update the estimation results of the zero-inflation probability and mean parameter under a zero-inflated Poisson or zero-inflated negative binomial assumption. We further discuss the role of regularization terms and “dropout” in dual-parameter boosted trees, and propose a general framework for insurance claim frequency modeling, which shows high prediction accuracy on both UBI and French motor third-party liability datasets, as well as the interpretability. The potential of extensive driving behavior features has been further verified on a Chinese insurance dataset, and the factors that have a significant impact on vehicle risk are identified and quantified on this basis. In addition, we discuss in detail the key points of applying boosted trees in actuarial science, which also promotes predictive insurance analytics.
更多查看译文
关键词
C53,G22
AI 理解论文
溯源树
样例
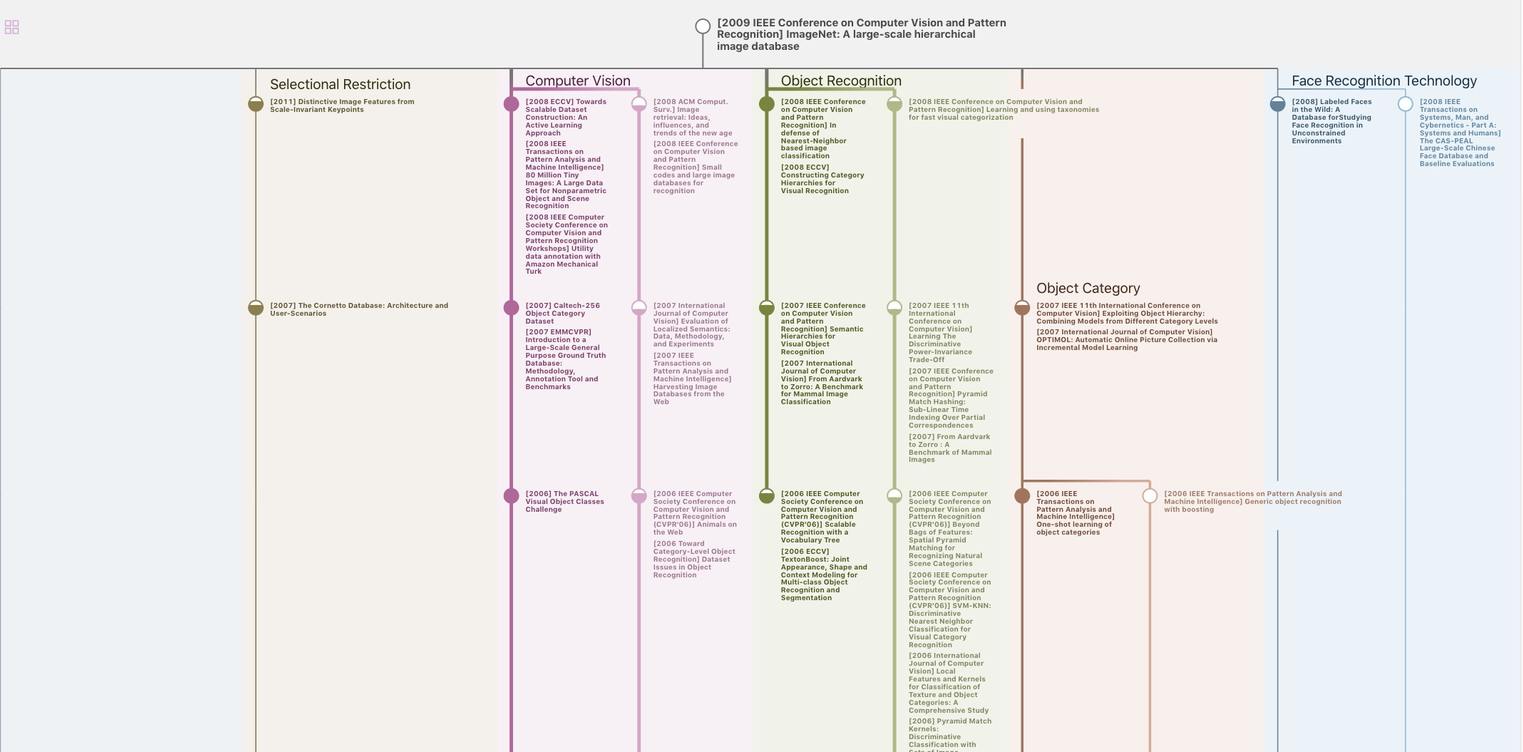
生成溯源树,研究论文发展脉络
Chat Paper
正在生成论文摘要