A physics-regularized data-driven approach for health prognostics of complex engineered systems with dependent health states
Reliability Engineering & System Safety(2022)
摘要
Advances in sensing technology enable the monitoring of critical operating parameters of complex engineering systems. However, having sensor measurements does not necessarily imply that one has observed the true system health states, which are often hidden and need to be estimated from observable sensor signals. This paper proposes a physics-regularized data-driven approach for the health prognostics of complex engineered systems with multiple hidden and dependent health states. The framework consists of a data layer and a physics layer. The data layer captures the statistically-correlated temporal dynamics of hidden system states (such as degradation), while the physics layer imposes regularizations among observed system operating parameters and system health states through system working principles and governing physics. The proposed approach addresses some common challenges arising from the health prognostics of complex engineered systems, including the integration of engineering domain knowledge and sensor data streams, the estimation of hidden system health states from monitored system operation parameters, and the statistical dependency among the temporal dynamics of multiple system state variables. A case study based on a real dataset is presented to illustrate the proposed physical–statistical approach. It is shown that the interpretability of data-driven system prognostics can be significantly strengthened if a solid connection is established between sensor data and system physics.
更多查看译文
关键词
Prognostics,Reliability,Physics-informed statistical learning,Archimedean copula,Cubic B-splines,Dynamic models
AI 理解论文
溯源树
样例
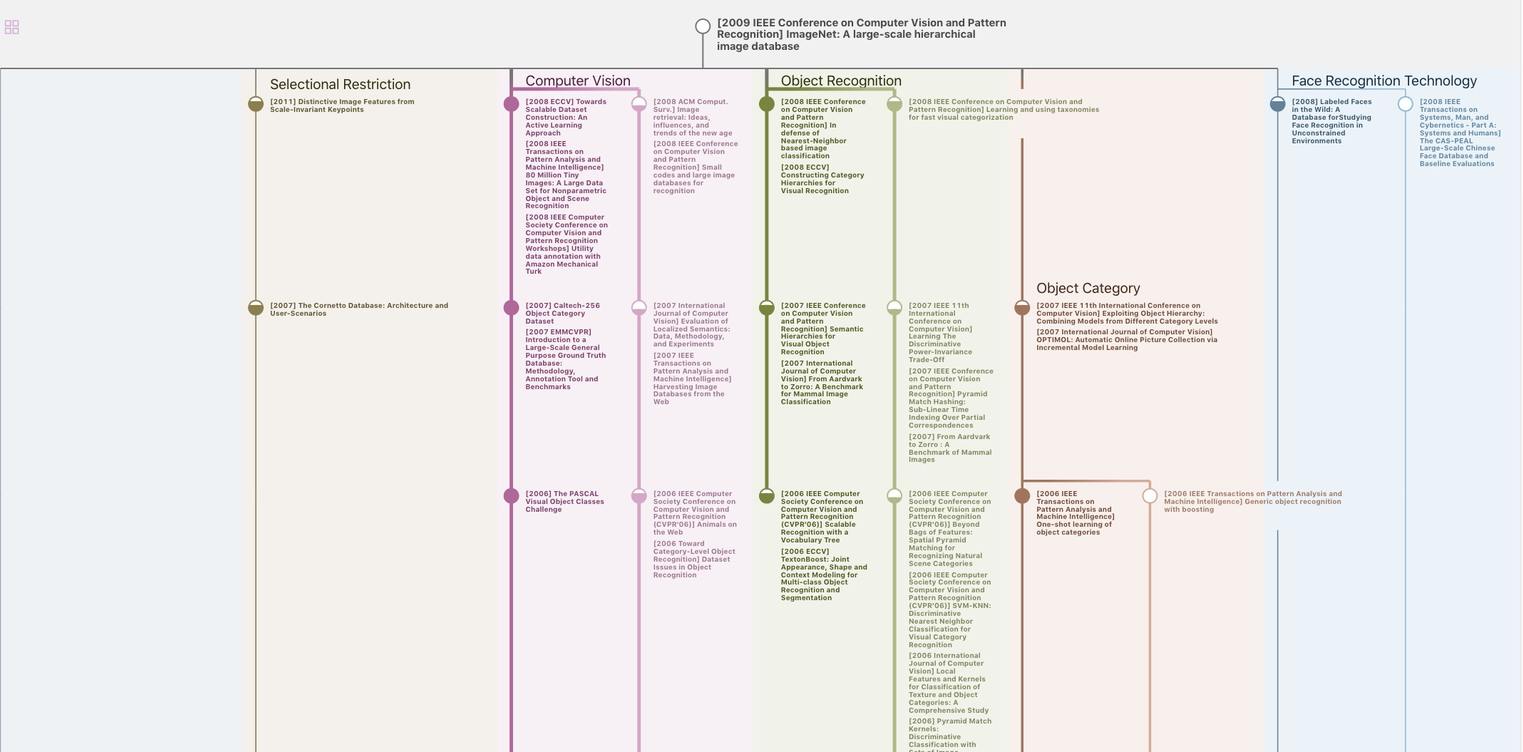
生成溯源树,研究论文发展脉络
Chat Paper
正在生成论文摘要